Model-Free Counterfactual Credit Assignment
user-5f8cf9244c775ec6fa691c99(2021)
摘要
Credit assignment in reinforcement learning is the problem of measuring an action’s influence on future rewards. In particular, this requires separating \emph{skill} from \emph{luck}, ie. disentangling the effect of an action on rewards from that of external factors and subsequent actions. To achieve this, we adapt the notion of counterfactuals from causality theory to a model-free RL setup. The key idea is to condition value functions on \emph{future} events, by learning to extract relevant information from a trajectory. We then propose to use these as future-conditional baselines and critics in policy gradient algorithms and we develop a valid, practical variant with provably lower variance, while achieving unbiasedness by constraining the hindsight information not to contain information about the agent’s actions. We demonstrate the efficacy and validity of our algorithm on a number of illustrative problems.
更多查看译文
关键词
credit,model-free
AI 理解论文
溯源树
样例
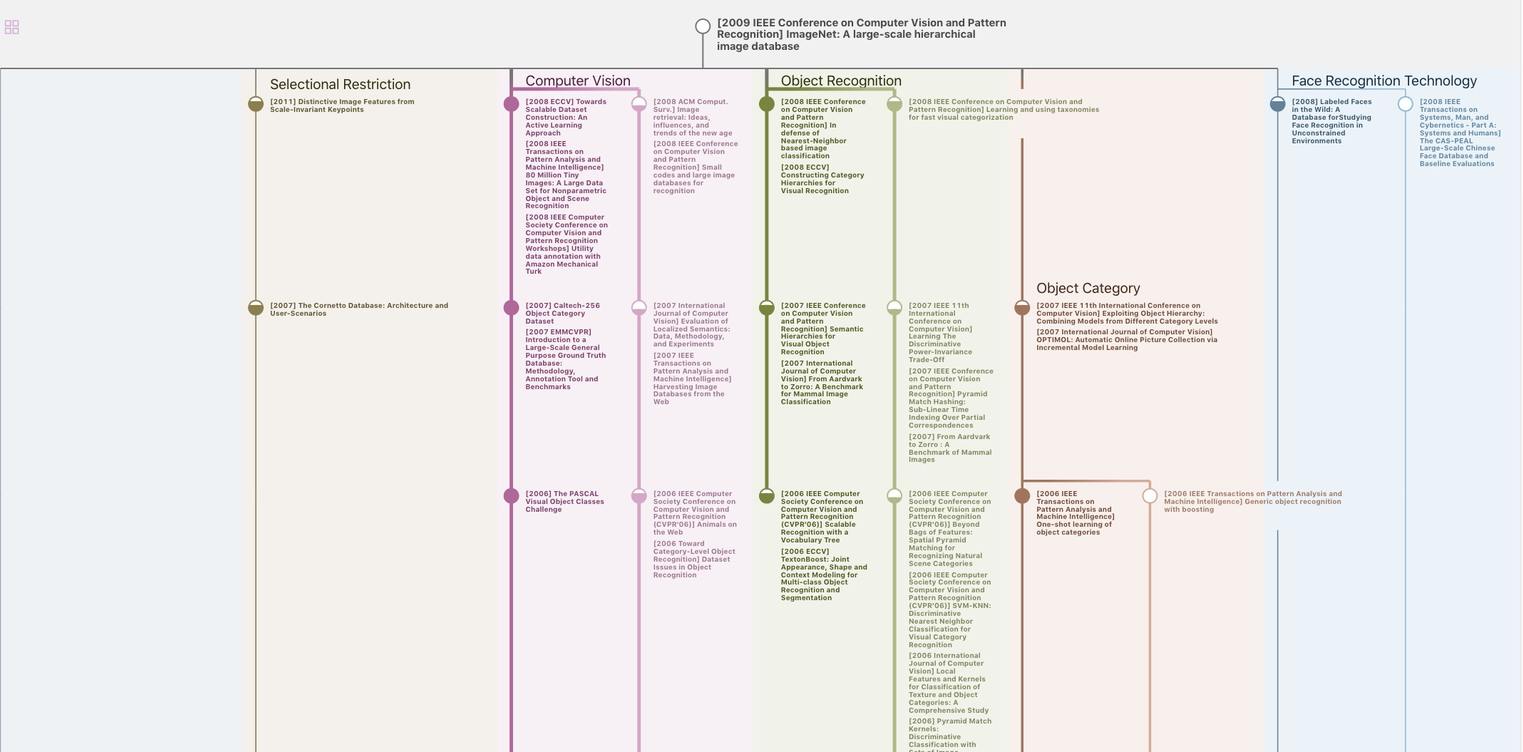
生成溯源树,研究论文发展脉络
Chat Paper
正在生成论文摘要