Federated Averaging as Expectation Maximization
user-5bd69975530c70d56f390249(2021)
摘要
Federated averaging (FedAvg), despite its simplicity, has been the main approach in training neural networks in the federated learning setting. In this work, we show that the algorithmic choices of the FedAvg algorithm correspond to optimizing a single objective function that involves the global and all of the shard specific models using a hard version of the well known Expectation-Maximization (EM) algorithm. As a result, we gain a better understanding of the behavior and design choices of federated averaging while being able to provide interesting connections to recent literature. Based on this view, we further propose FedSparse, a version of federated averaging that employs prior distributions to promote model sparsity. In this way, we obtain a procedure that leads to reductions in both server-client and client-server communication costs as well as more efficient models.
更多查看译文
关键词
Maximization,Shard,Artificial neural network,Expectation–maximization algorithm,Mathematical optimization,Computer science,Federated learning,Single objective
AI 理解论文
溯源树
样例
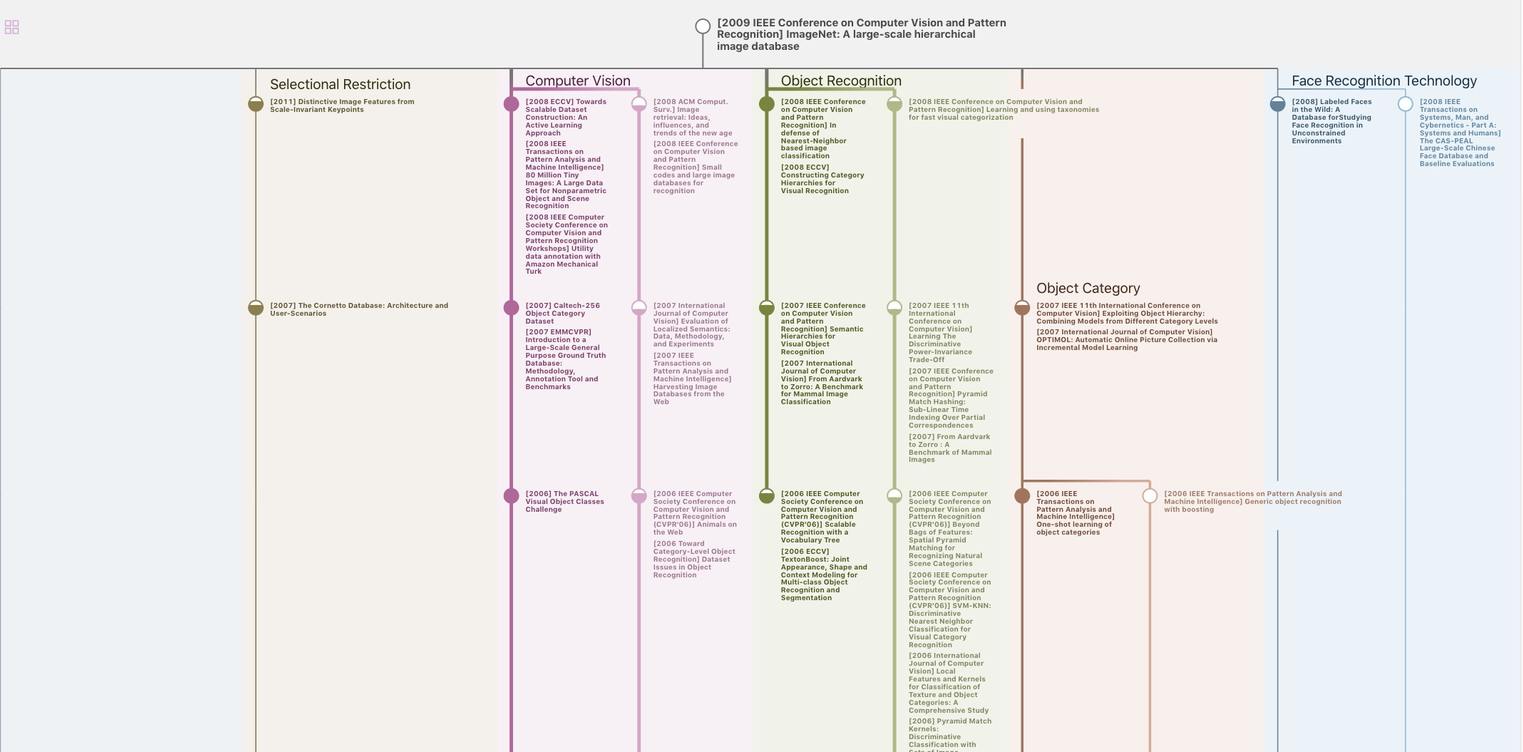
生成溯源树,研究论文发展脉络
Chat Paper
正在生成论文摘要