Semi-Supervised Link Prediction Based On Non-Negative Matrix Factorization For Temporal Networks *
CHAOS SOLITONS & FRACTALS(2021)
摘要
Temporal link prediction is a critical issue in the field of network analysis, which predicts the future links in temporal networks. In order to facilitate the performance of temporal link prediction approach, we should fuse the topological and temporal properties. Here we propose a novel semi-supervised non negative matrix factorization method for temporal link prediction. Potential useful prior information is obtained from community which naturally expresses topological structure of networks. Moreover, we capture the temporal information of networks by graph communicability. We factorize the communicability matrix respect to the temporal network by setting the historic networks as graph regularization and priors as node pair constraints. Extensive experiments on both synthetic and real-world networks demonstrate that the proposed method can improve the accuracy of temporal link prediction. Especially, our method performs stably when the wrong prior rate is up to 30% . (c) 2021 Elsevier Ltd. All rights reserved.
更多查看译文
关键词
Temporal link prediction, Semi-supervised learning, Graph regularized non-negative matrix, factorization, Temporal networks
AI 理解论文
溯源树
样例
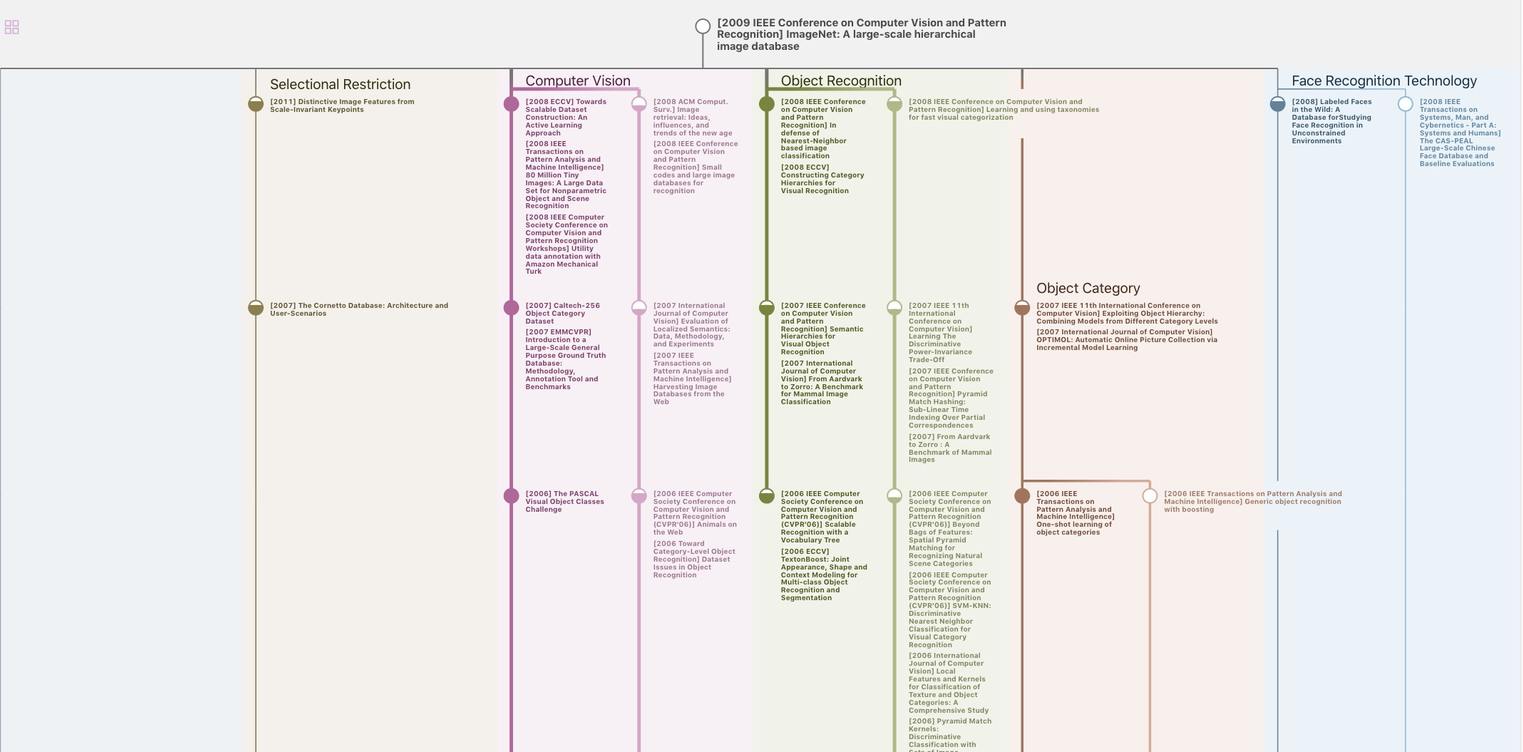
生成溯源树,研究论文发展脉络
Chat Paper
正在生成论文摘要