A Forest Fire Detection System Based On Ensemble Learning
FORESTS(2021)
摘要
Due to the various shapes, textures, and colors of fires, forest fire detection is a challenging task. The traditional image processing method relies heavily on manmade features, which is not universally applicable to all forest scenarios. In order to solve this problem, the deep learning technology is applied to learn and extract features of forest fires adaptively. However, the limited learning and perception ability of individual learners is not sufficient to make them perform well in complex tasks. Furthermore, learners tend to focus too much on local information, namely ground truth, but ignore global information, which may lead to false positives. In this paper, a novel ensemble learning method is proposed to detect forest fires in different scenarios. Firstly, two individual learners Yolov5 and EfficientDet are integrated to accomplish fire detection process. Secondly, another individual learner EfficientNet is responsible for learning global information to avoid false positives. Finally, detection results are made based on the decisions of three learners. Experiments on our dataset show that the proposed method improves detection performance by 2.5% to 10.9%, and decreases false positives by 51.3%, without any extra latency.
更多查看译文
关键词
forest fire detection, deep learning, ensemble learning, Yolov5, EfficientDet, EfficientNet
AI 理解论文
溯源树
样例
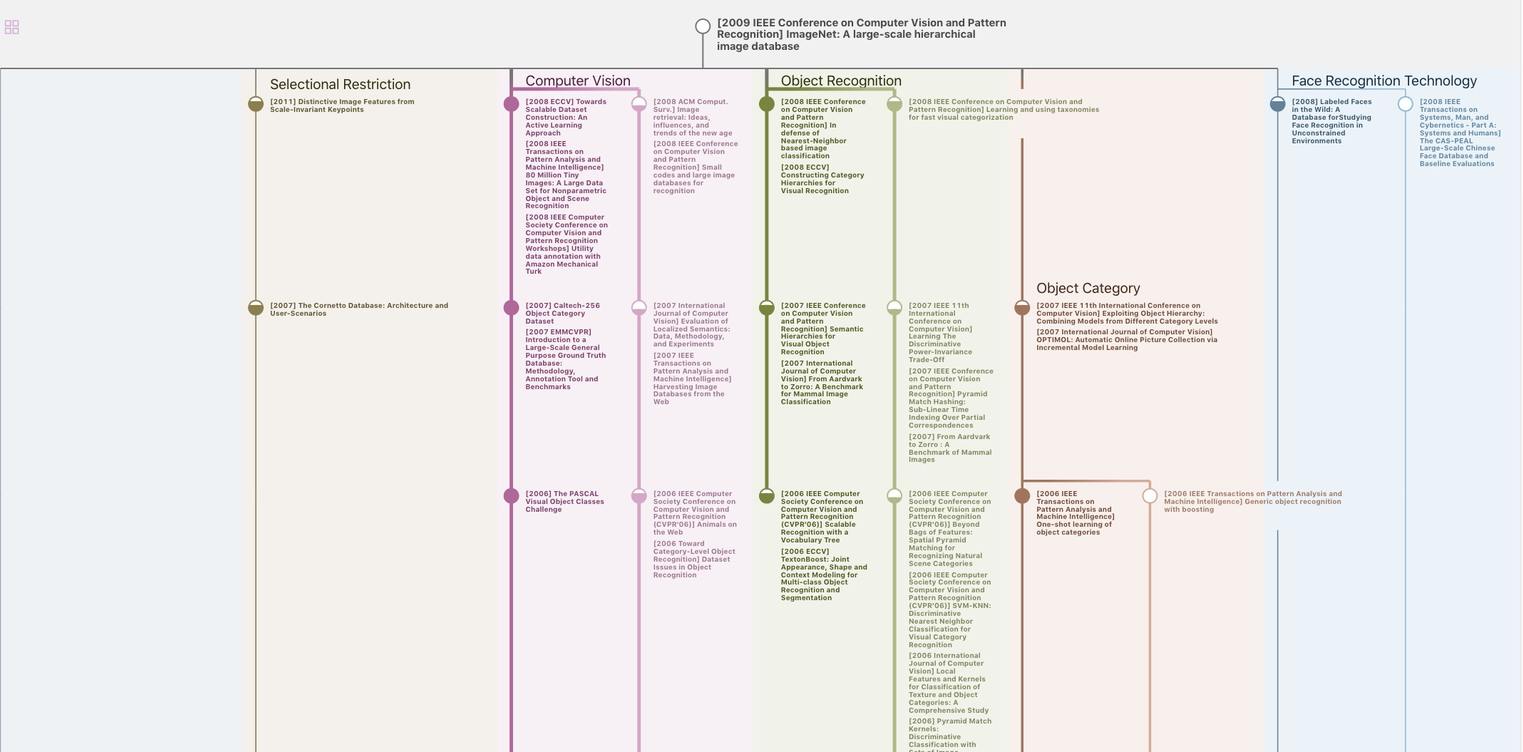
生成溯源树,研究论文发展脉络
Chat Paper
正在生成论文摘要