Robust And Label Efficient Bi-Filtering Graph Convolutional Networks For Node Classification
KNOWLEDGE-BASED SYSTEMS(2021)
摘要
Due to their success at node classification, Graph Convolutional Networks (GCN) have raised a research upsurge of deep learning on graph-structured data. For the semi-supervised classification, graph convolution essentially acts as a low-pass filter on graph spectral domain. According to Graph Signal Processing theory, the low-pass filter in GCN is a finite impulse response (FIR) graph filter. However, compared with FIR graph filters, infinite impulse response (IIR) graph filters exhibit more powerful representation ability and flexibility. Intuitively, it is feasible to replace FIR filter in GCN with IIR graph filter to improve GCN. Therefore, inspired by the direct implementation of IIR graph filters, we propose a Bi-filtering Graph Convolutional Network (BGCN) which can be realized by simply cascading two sub filtering modules. Experimental results demonstrate that BGCN works well in node classification task and achieves comparable performance to GCN and its variants. The improvement of BGCN, however, is at the expense of a time-complexity increase. To simplify the proposed BGCN, we construct a Simple Bi-filtering Graph Convolution framework (SBGC) from the perspective of Graph Signal Processing. Furthermore, for the implementations of BGCN and SBGC, we design a novel low-pass graph filter to capture the low-frequency components that are beneficial to data representation for the task of node classification. Extensive experiments show that SBGC not only outperforms other baseline methods in performance, but also keeps a high level in computational efficiency. Moreover, it is particularly worth noting that both BGCN and SBGC are robust to feature noise and exhibit high label efficiency. (C) 2021 Published by Elsevier B.V.
更多查看译文
关键词
Graph Convolutional Networks (GCN), Graph signal processing, Infinite impulse response (IIR), Node classification, Low-pass filter
AI 理解论文
溯源树
样例
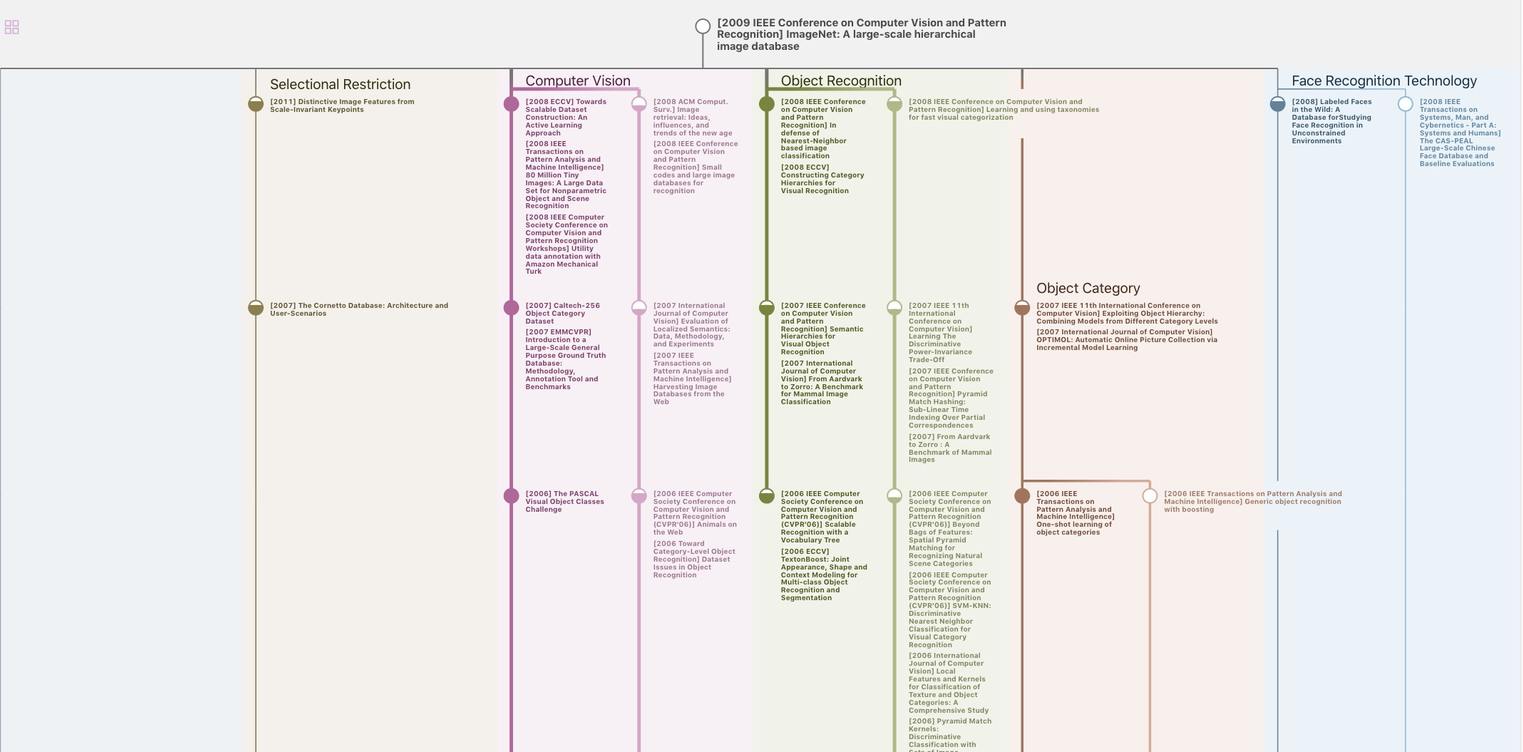
生成溯源树,研究论文发展脉络
Chat Paper
正在生成论文摘要