Neural Network Adaptation And Data Augmentation For Multi-Speaker Direction-Of-Arrival Estimation
IEEE-ACM TRANSACTIONS ON AUDIO SPEECH AND LANGUAGE PROCESSING(2021)
摘要
Deep neural networks have been successfully applied to sound direction-of-arrival estimation under challenging conditions. However, such a learning-based approach requires a large amount of labeled training data, which is difficult to acquire. To address this problem, we propose a novel approach for multi-speaker direction-of-arrival estimation with data augmentation and weakly-supervised domain adaptation. We generate source domain data with simulation, and collect real data annotated with the number of sound sources as the weak labels. The real data are further augmented by mixing single-source segments. Then, weakly-supervised domain adaptation is applied to models pre-trained on the simulated data. We define a loss function for the adaptation process which exploits the weak labels and the mixture component information in the augmented data. Experiments with real robot audio data show that our proposed approach achieves similar performance as if the fully-labeled real data are used. This paper suggests an effective development procedure for DOA estimation models applied to new types of microphone arrays with minimal data collection efforts.
更多查看译文
关键词
Data models, Adaptation models, Direction-of-arrival estimation, Neural networks, Location awareness, Data collection, Robots, DOA estimation, data augmentation, sound source localization, weakly-supervised learning
AI 理解论文
溯源树
样例
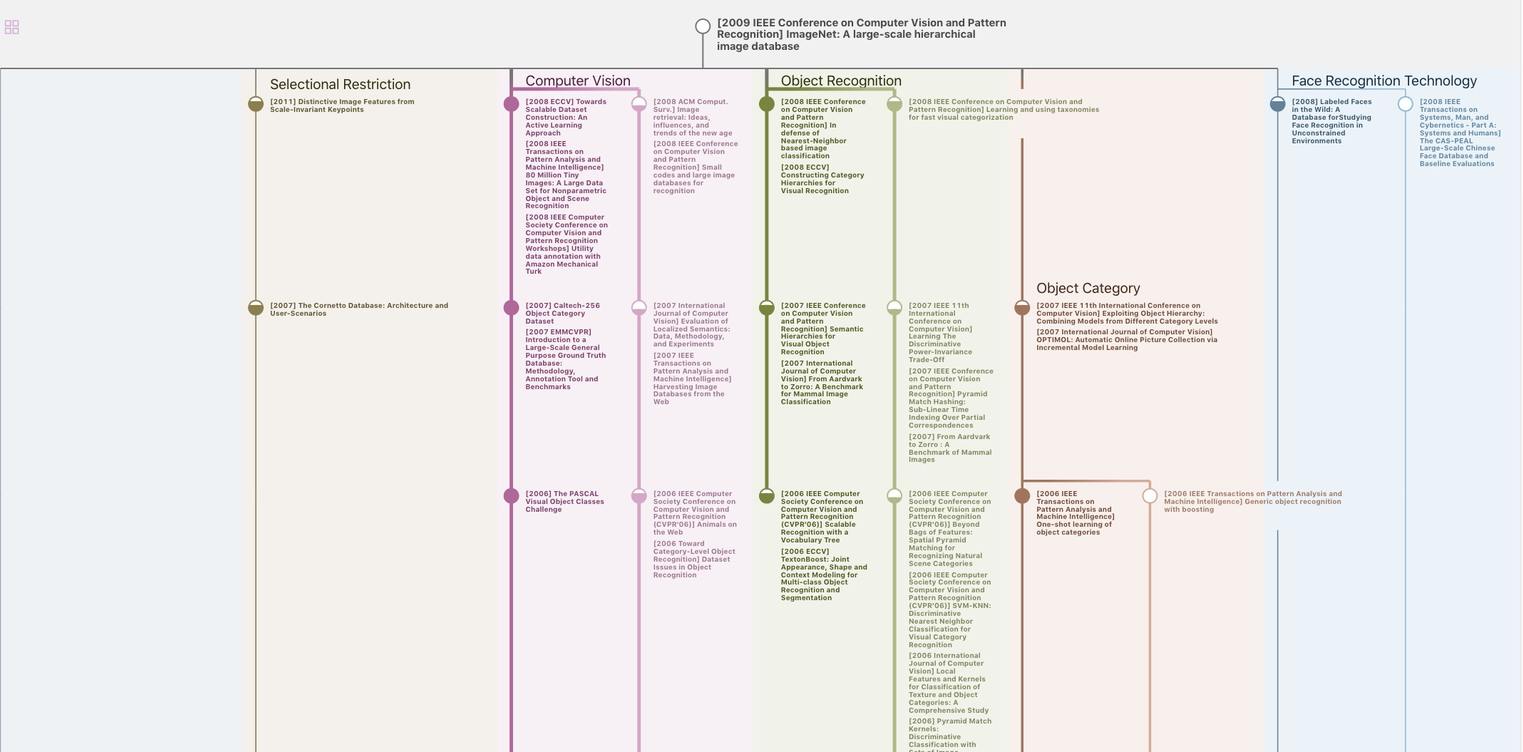
生成溯源树,研究论文发展脉络
Chat Paper
正在生成论文摘要