Hybrid Model For Predicting Anomalous Large Passenger Flow In Urban Metros
IET INTELLIGENT TRANSPORT SYSTEMS(2020)
摘要
Machine learning models have been widely adopted for passenger flow prediction in urban metros; however, the authors find machine learning models may underperform under anomalous large passenger flow conditions. In this study, they develop a prediction framework that combines the advantage of complex network models in capturing the collective behaviour of passengers and the advantage of online learning algorithms in characterising rapid changes in real-time data. The proposed method considerably improves the accuracy of passenger flow prediction under anomalous conditions. This study can also serve as an exploration of interdisciplinary methods for transportation research.
更多查看译文
关键词
learning (artificial intelligence), transportation, complex networks, rail traffic, real-time systems, behavioural sciences, transportation, real-time data, online learning algorithms, collective behaviour, urban metros, hybrid model, anomalous conditions, passenger flow prediction, complex network models, prediction framework, anomalous large passenger flow conditions, machine learning models
AI 理解论文
溯源树
样例
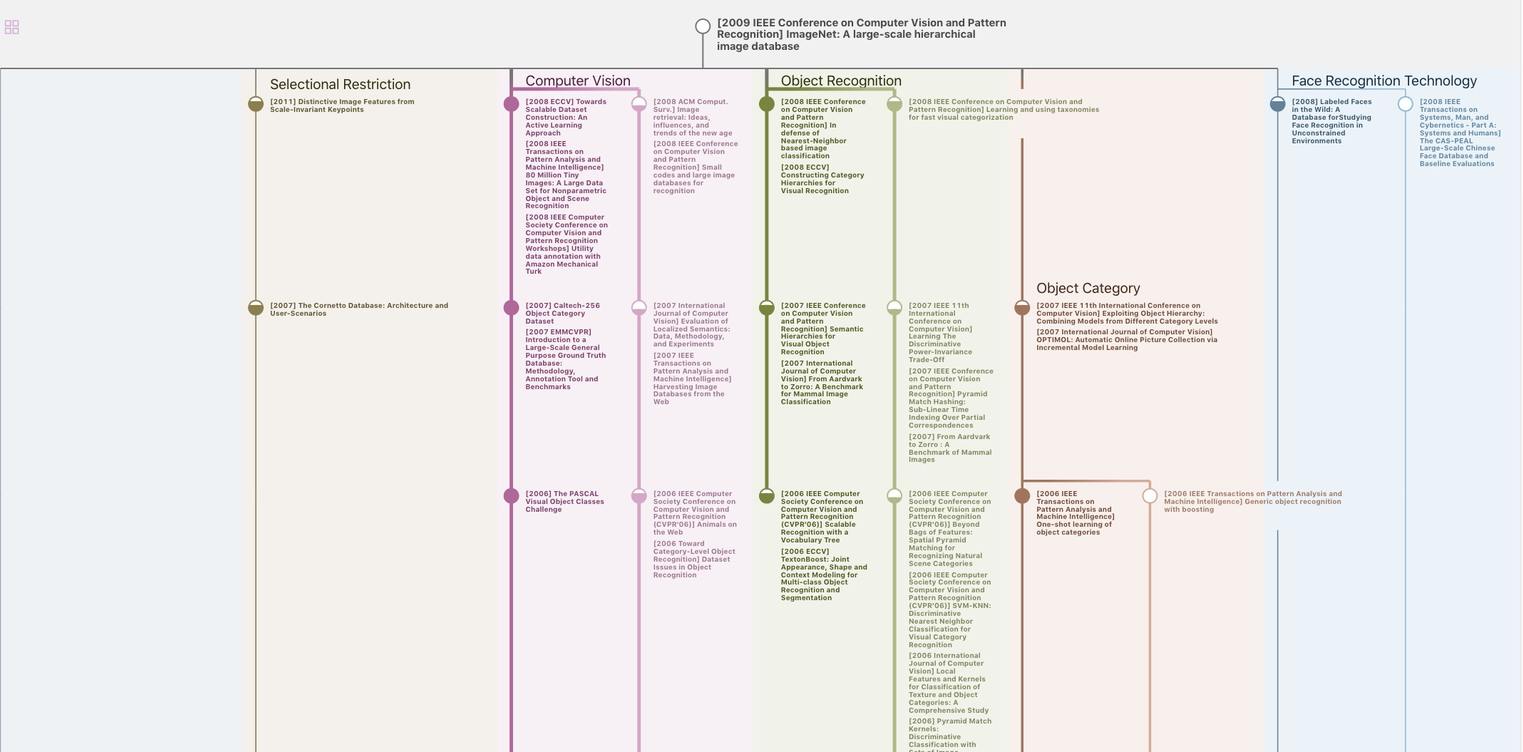
生成溯源树,研究论文发展脉络
Chat Paper
正在生成论文摘要