A Deep Learning-Based Power Control And Consensus Performance Of Spectrum Sharing In The Cr Network
WIRELESS COMMUNICATIONS & MOBILE COMPUTING(2021)
摘要
The cognitive radio network (CRN) is aimed at strengthening the system through learning and adjusting by observing and measuring the available resources. Due to spectrum sensing capability in CRN, it should be feasible and fast. The capability to observe and reconfigure is the key feature of CRN, while current machine learning techniques work great when incorporated with system adaption algorithms. This paper describes the consensus performance and power control of spectrum sharing in CRN. (1) CRN users are considered noncooperative users such that the power control policy of a primary user (PU) is predefined keeping the secondary user (SU) unaware of PU's power control policy. For a more efficient spectrum sharing performance, a deep learning power control strategy has been developed. This algorithm is based on the received signal strength at CRN nodes. (2) An agent-based approach is introduced for the CR user's consensus performance. (3) All agents reached their steady-state value after nearly 100 seconds. However, the settling time is large. Sensing delay of 0.4 second inside whole operation is identical. The assumed method is enough for the representation of large-scale sensing delay in the CR network.
更多查看译文
AI 理解论文
溯源树
样例
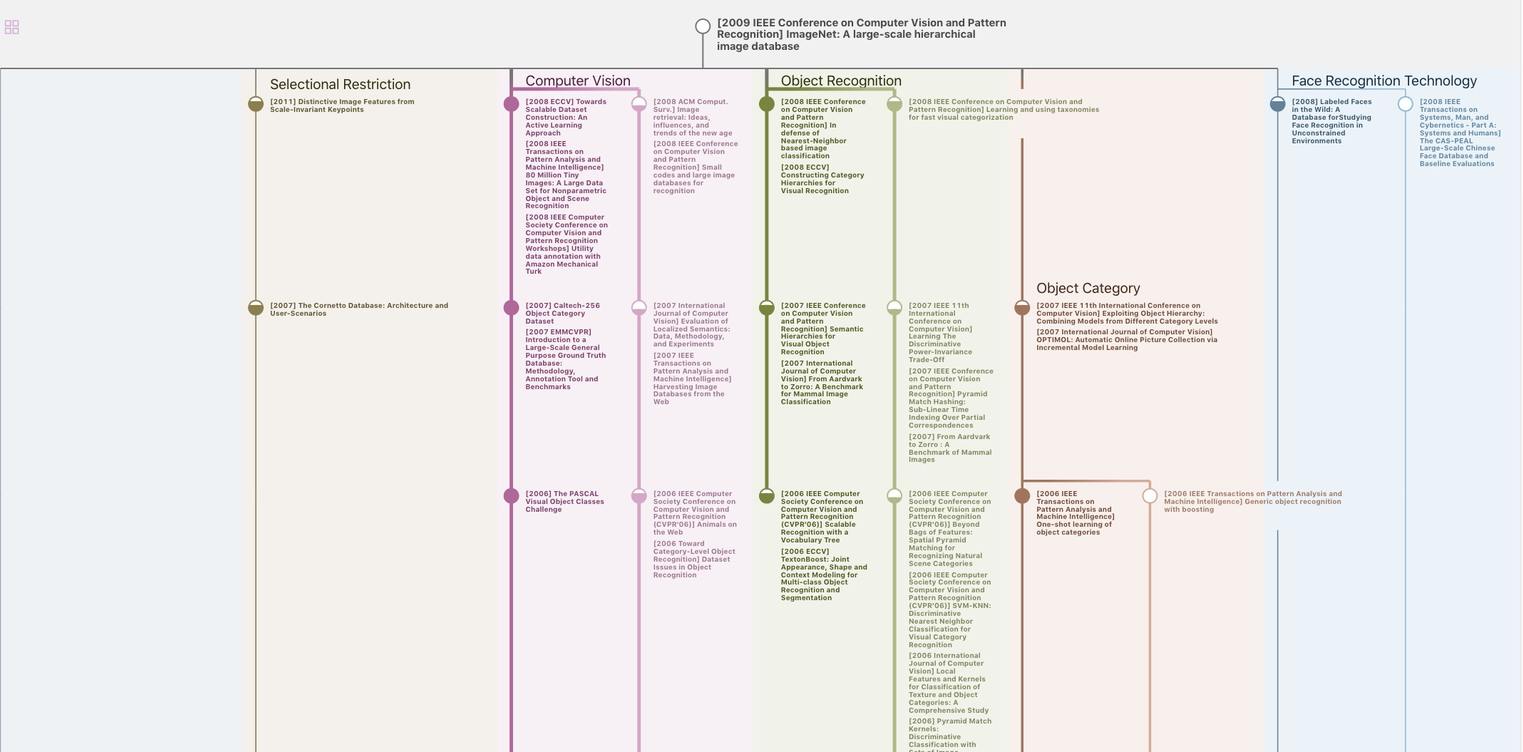
生成溯源树,研究论文发展脉络
Chat Paper
正在生成论文摘要