Active Learning Of Bayesian Probabilistic Movement Primitives
IEEE ROBOTICS AND AUTOMATION LETTERS(2021)
摘要
Learning from Demonstration permits non-expert users to easily and intuitively reprogram robots. Among approaches embracing this paradigm, probabilistic movement primitives (ProMPs) are a well-established and widely used method to learn trajectory distributions. However, providing or requesting useful demonstrations is not easy, as quantifying what constitutes a good demonstration in terms of generalization capabilities is not trivial. In this letter, we propose an active learning method for contextual ProMPs for addressing this problem. More specifically, we learn the trajectory distributions using a Bayesian Gaussian mixture model (BGMM) and then leverage the notion of epistemic uncertainties to iteratively choose new context query points for demonstrations. We show that this approach reduces the required number of human demonstrations. We demonstrate the effectiveness of the approach on a pouring task, both in simulation and on a real 7-DoF Franka Emika robot.
更多查看译文
关键词
Imitation learning, learning from demonstration, incremental learning
AI 理解论文
溯源树
样例
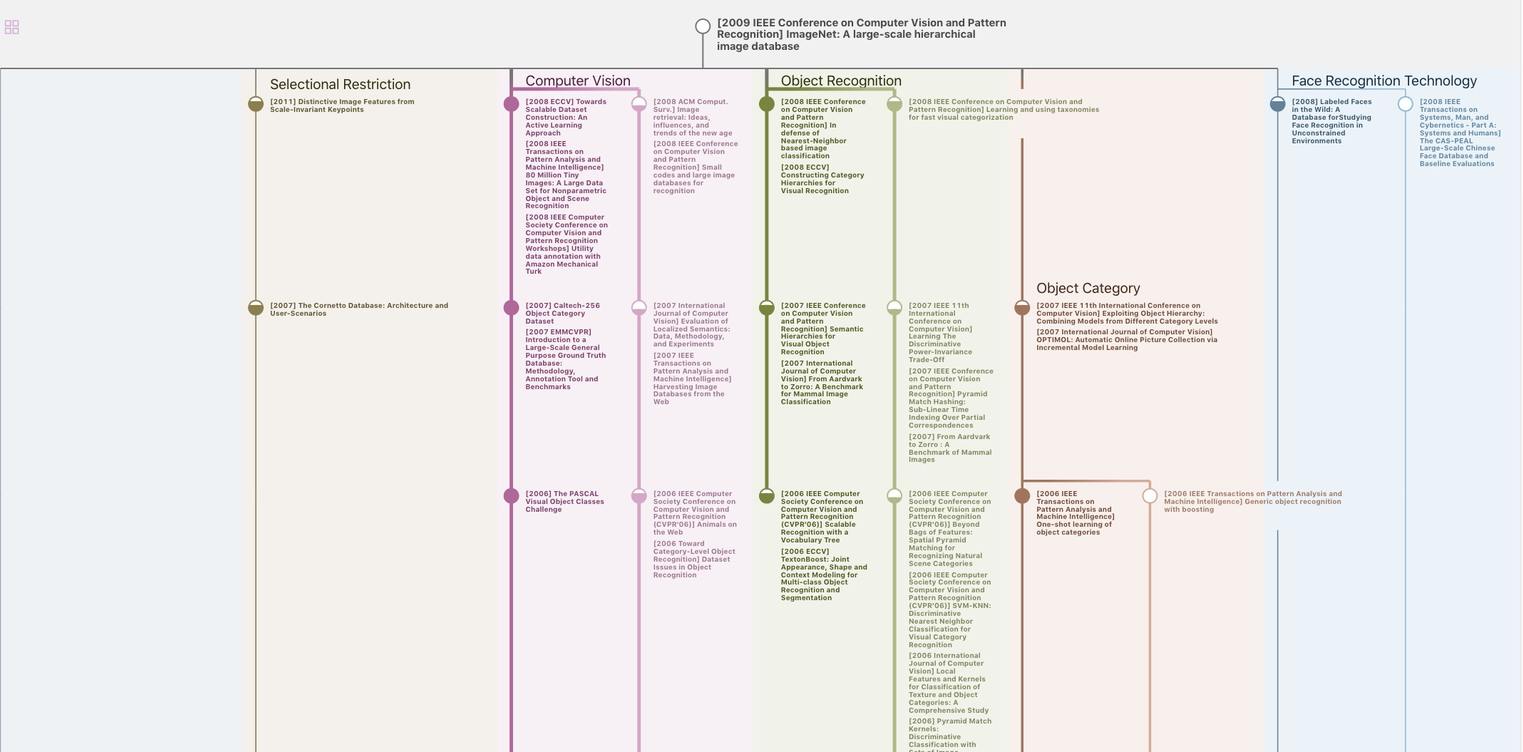
生成溯源树,研究论文发展脉络
Chat Paper
正在生成论文摘要