Segmentation Of Point Clouds Via Joint Semantic And Geometric Features For 3d Modeling Of The Built Environment
AUTOMATION IN CONSTRUCTION(2021)
摘要
Generating 3D models from point cloud data is a common Virtual Design and Construction (VDC) service. Research has focused on automating several key steps, including segmenting point clouds based on appearance and geometric attributes. These methods dominantly use Manhattan-World assumptions in their approach. Hence, in cases where building systems are close to architectural/structural elements, these methods result in over-segmentation and require significant fine-tuning by the users. To overcome these limitations, this paper presents a learning method based on Markov Random Field (MRF) that assigns semantic labels to point cloud segment. The MRF enforces coherence between the semantic (e.g., beam, column, wall, ceiling, floor, pipe) and geometric labels (e.g., horizontal, vertical, cylindrical), and it uses the neighborhood context to enhance the semantic labeling accuracy. Experimental results show an average accuracy of 90% on semantic labeling, achieving state-of-the-art performance on labeling beam, ceiling, column, floor, pipe, and wall elements.
更多查看译文
关键词
Scan-to-BIM, Point cloud, Segmentation, Semantic labeling, Geometric labeling, Semantic segmentation, Machine learning, Support vector machine (SVM), AdaBoost classifier, Conditional random field (CRF), Markov random field (MRF)
AI 理解论文
溯源树
样例
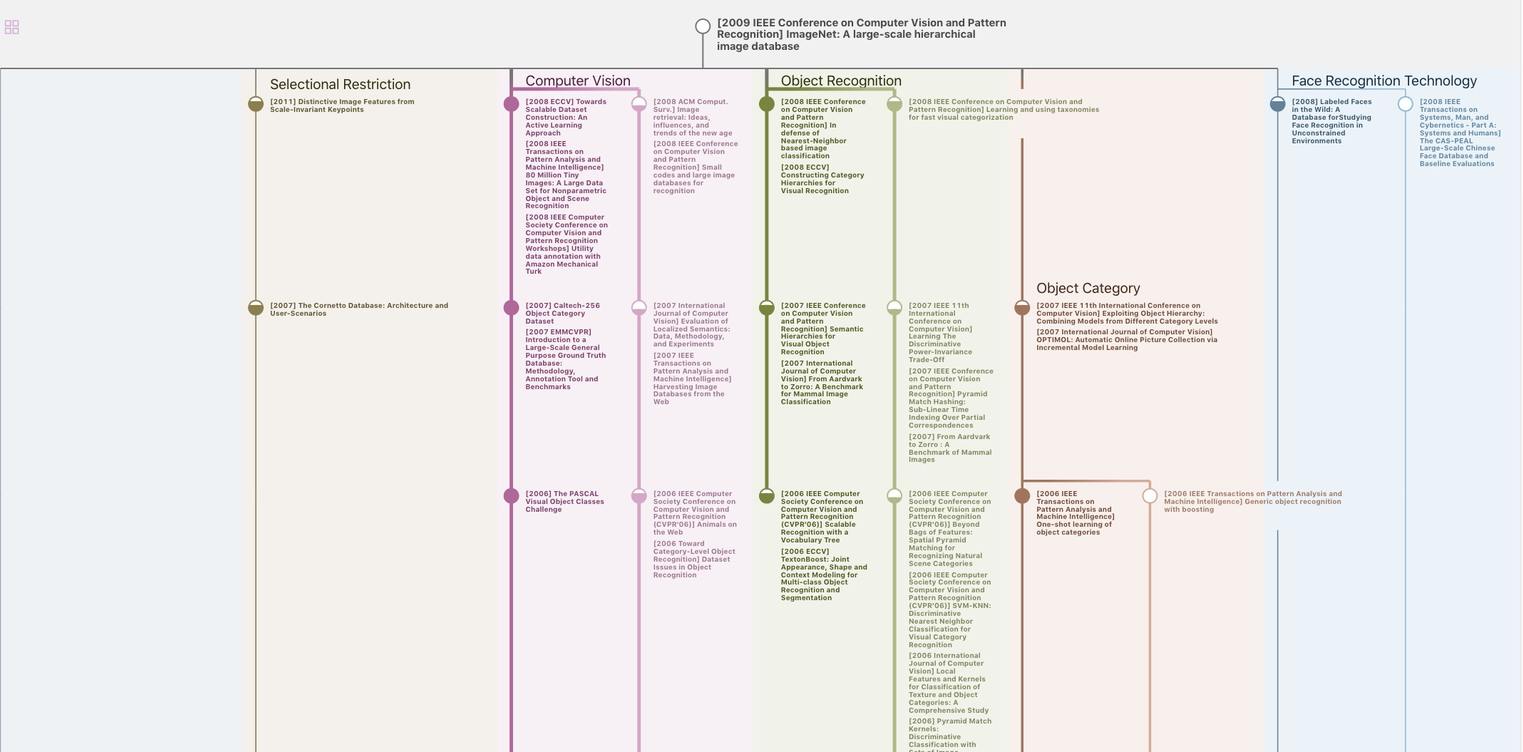
生成溯源树,研究论文发展脉络
Chat Paper
正在生成论文摘要