Deep Learning Based Automated Inspection Of Weak Microscratches In Optical Fiber Connector End-Face
IEEE TRANSACTIONS ON INSTRUMENTATION AND MEASUREMENT(2021)
摘要
Surface defects on optical fiber end-face, such as scratches, cause heavy loss and low data-transfer rate. Therefore, the inspection of optical fiber end-face defect cannot he neglected. Mast optical fiber defect detection methods ignore the striped pattern of scratches and easily cause incomplete segmentation. In this article, a novel method based on deep convolutional neural networks (DCNNs) for weak microscratches inspection in the optical fiber end-face is proposed. First, a new feature pyramid structure with criss-cross max-pooling (CCMP) block is developed to extract precise boundary features in all directions and generate feature maps. Second, these feature maps are fed into the spatial attention upsample (SAU) module to recover a full-resolution feature map and localize the defects. Finally, multiple scratches with various types can he detected in the images. This article uses many real optical fiber images as experimental images. The experimental results demonstrate that the proposed method yields good comprehensive performance with a mean pixel accuracy (mPA) of 92.70% and a mean intersection over union (mIoU) of 85.35%.
更多查看译文
关键词
Deep neural network, defect detection, optical fiber end-face, scratch inspection
AI 理解论文
溯源树
样例
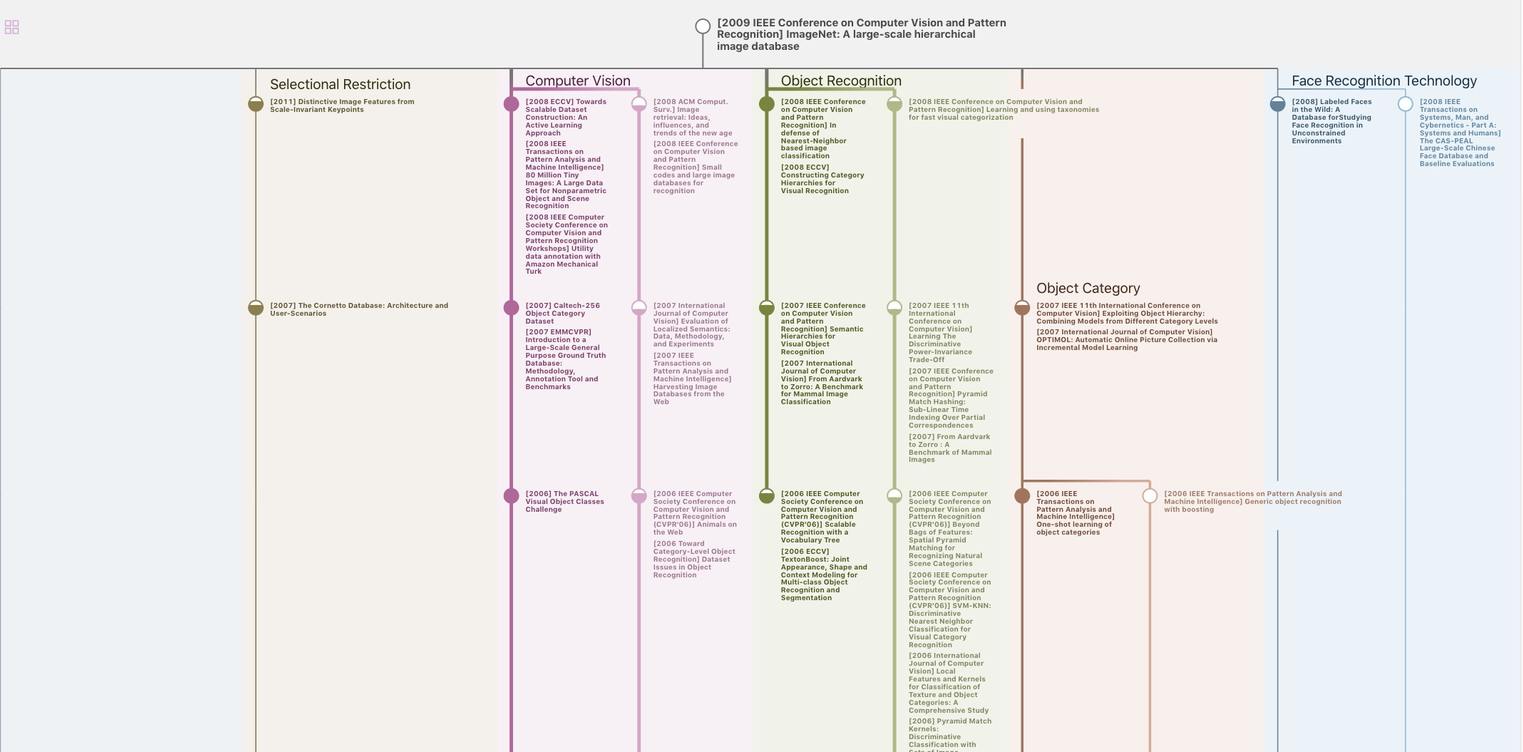
生成溯源树,研究论文发展脉络
Chat Paper
正在生成论文摘要