A Risk-Averse Optimization Approach To Human-Robot Collaboration In Robotic Fruit Harvesting
COMPUTERS AND ELECTRONICS IN AGRICULTURE(2021)
摘要
The marketability and adoption of robotic systems in agriculture is largely limited by economic and technology barriers that prevent highly efficient autonomous operations at a cost that justifies the generally low commodity values. From the technology perspective, autonomous systems exhibit brittleness in uncontrolled, unforeseen, and unlearned situations, prevalent in complex agricultural environments, which leads to inefficient operations and production losses. In this setting, human workers can complement autonomous systems through cooperation to not only minimize the total production cost by reducing the burden on autonomy, but also improve productivity by overcoming the shortfalls of autonomous systems. To this end, the focus of this work is on developing a systematic approach for human-robot collaboration in robotic fruit harvesting. Specifically, the main contribution of this work is in the development of stochastic optimization models for human-robot collaboration using a risk-averse framework to identify optimal harvester servicing policies that minimize the risk of economic losses all while guaranteeing a desired level of financial return. The solution of the models provide optimal policies for a human collaborator to service various sub-systems of a robotic harvester to improve performance in light of any operational inefficiencies in the sub-systems. The developed risk-averse optimization solution is validated in a simulated grove environment using data for various citrus varieties. Economic gains through human collaboration are confirmed through increase in the value of production and the net profit. The simulation results also provide insights into the temporal variation in the required human collaboration during the length of harvesting operation, which can benefit agricultural operations management in effective labor allocation. Furthermore, the intrinsic self-diagnosing ability of the developed model identifies component-level inefficiencies to provide an optimal servicing policy, thereby a human collaborator is not required to maintain situational awareness of the harvesting operation. Finally, it is demonstrated that the models can be solved to optimality in a relatively short time (?2s) using standard commercial optimization packages.
更多查看译文
关键词
Co-robotics, Risk-reward optimization, Stochastic optimization
AI 理解论文
溯源树
样例
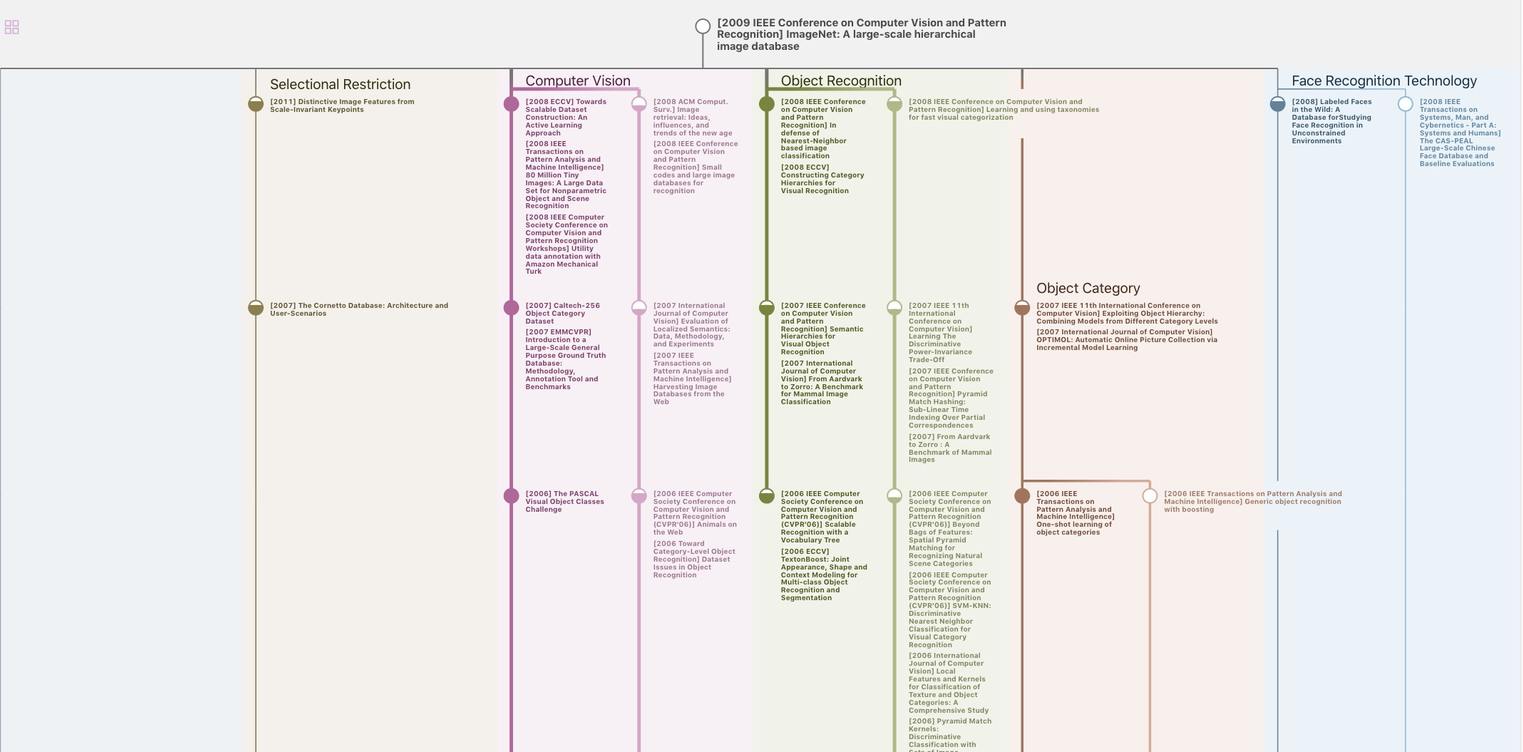
生成溯源树,研究论文发展脉络
Chat Paper
正在生成论文摘要