Towards Combined Open Set Recognition And Out-Of-Distribution Detection For Fine-Grained Classification
VISAPP: PROCEEDINGS OF THE 16TH INTERNATIONAL JOINT CONFERENCE ON COMPUTER VISION, IMAGING AND COMPUTER GRAPHICS THEORY AND APPLICATIONS - VOL. 5: VISAPP(2021)
摘要
We analyze the two very similar problems of Out-of-Distribution (OOD) Detection and Open Set Recognition (OSR) in the context of fine-grained classification. Both problems are about detecting object classes that a classifier was not trained on, but while the former aims to reject invalid inputs, the latter aims to detect valid but unknown classes. Previous works on OOD detection and OSR methods are evaluated mostly on very simple datasets or datasets with large inter-class variance and perform poorly in the fine-grained setting. In our experiments, we show that object detection works well to recognize invalid inputs and techniques from the field of fine-grained classification, like individual part detection or zooming into discriminative local regions, are helpful for fine-grained OSR.
更多查看译文
关键词
Fine-grained Classification, Out-of-Distribution Detection, Open Set Recognition
AI 理解论文
溯源树
样例
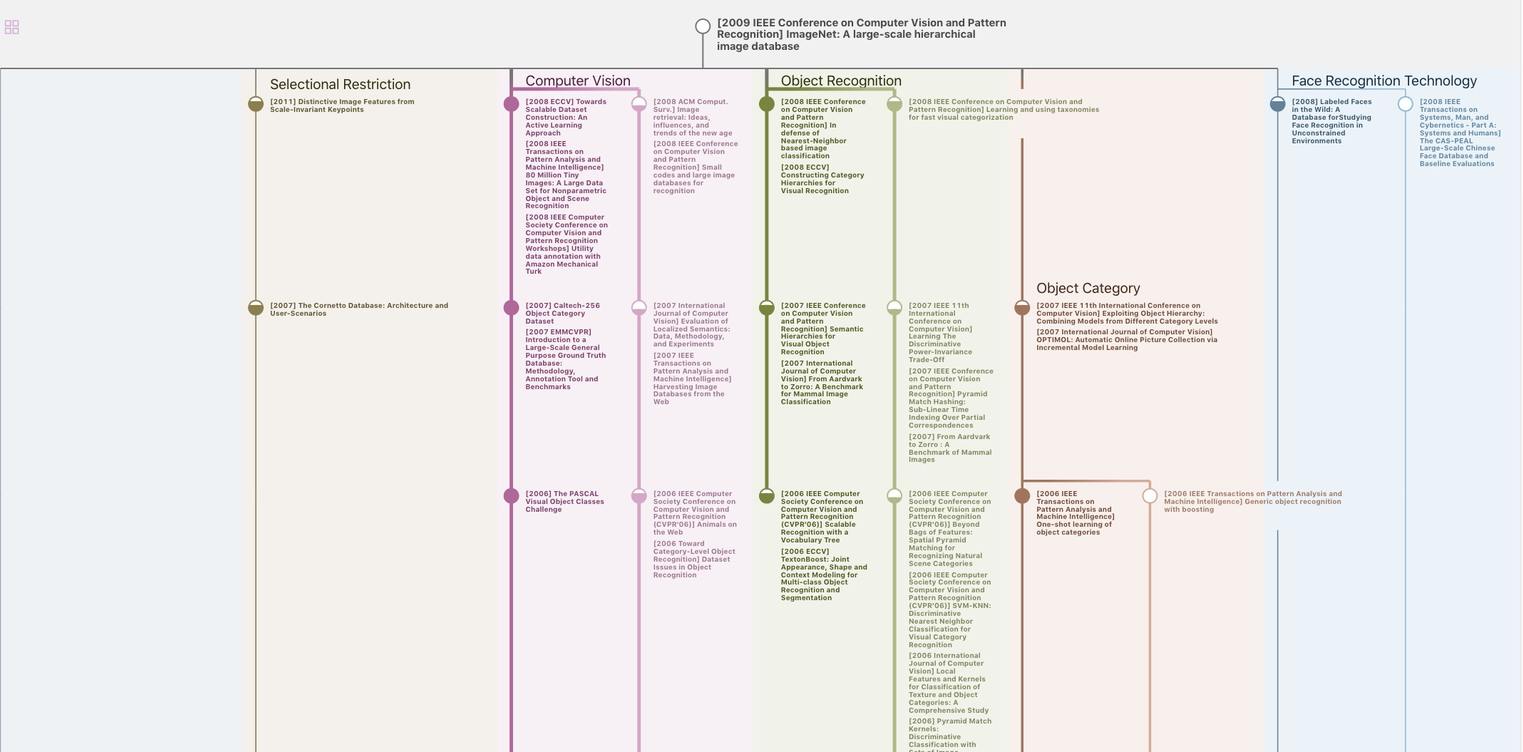
生成溯源树,研究论文发展脉络
Chat Paper
正在生成论文摘要