3D UNSUPERVISED KIDNEY GRAFT SEGMENTATION BASED ON DEEP LEARNING AND MULTI-SEQUENCE MRI
2021 IEEE 18TH INTERNATIONAL SYMPOSIUM ON BIOMEDICAL IMAGING (ISBI)(2021)
摘要
Image segmentation is one of the most popular problems in medical image analysis. Recently, with the success of deep neural networks, these powerful methods provide state of the art performance on various segmentation tasks. However, one of the main challenges relies on the high number of annotations that they need to be trained, which is crucial in medical applications. In this paper, we propose an unsupervised method based on deep learning for the segmentation of kidney grafts. Our method is composed of two different stages, the detection of the area of interest and the segmentation model that is able, through an iterative process, to provide accurate kidney draft segmentation without the need for annotations. The proposed framework works in the 3D space to explore all the available information and extract meaningful representations from Dynamic Contrast-Enhanced and T2 MRI sequences. Our method reports a dice of 89.8 +/- 3.1%, Hausdorff distance at percentile 95% of 5.8 +/- 0.41rnm and percentage of kidney volume difference of 5.9 +/- 5.7% on a test dataset of 29 patients subject to a kidney transplant.
更多查看译文
关键词
Unsupervised segmentation, kidney graft, multi-sequence MRI, Deep Learning, CNN
AI 理解论文
溯源树
样例
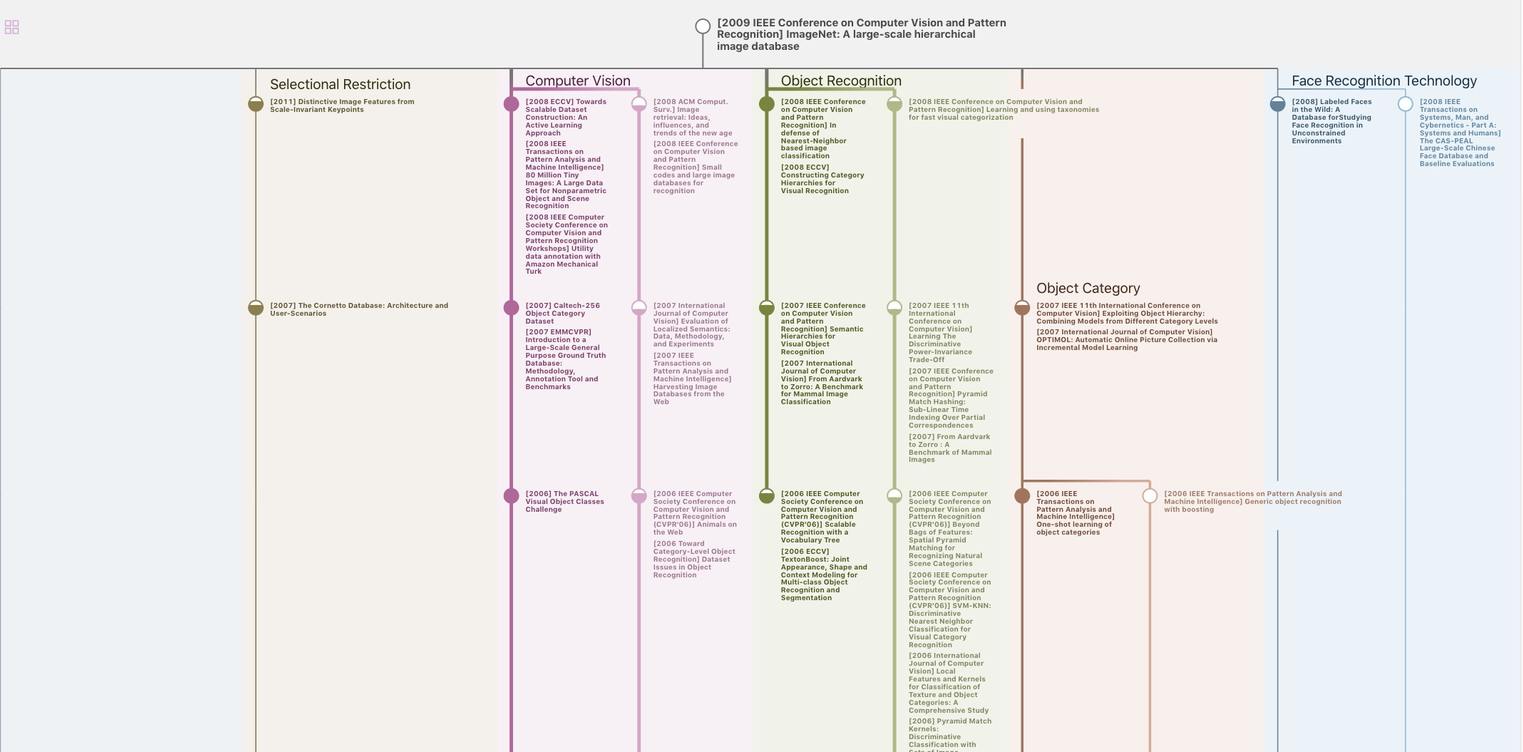
生成溯源树,研究论文发展脉络
Chat Paper
正在生成论文摘要