Three-Dimensional Mapping with Augmented Navigation Cost through Deep Learning
JOURNAL OF INTELLIGENT & ROBOTIC SYSTEMS(2021)
摘要
This work addresses the problem of mapping terrain features based on inertial and LiDAR measurements to estimate navigation cost, for an autonomous ground robot. The navigation cost quantifies the degree of how easy or difficult it is to navigate through different areas. Unlike most indoor applications, where surfaces are usually human-made, flat, and structured, external environments may be unpredictable as to the types and conditions of the travel surfaces, such as traction characteristics and inclination. Attaining full autonomy in outdoor environments requires a mobile ground robot to perform the fundamental localization and mapping tasks in unfamiliar environments, but with the added challenge of unknown terrain conditions. Autonomous motion in uneven terrain has been widely explored by the research community focusing on one or more of the several factors involved aiming at both safety and efficient displacement. A fuller representation of the environment is fundamental to increase confidence and to reduce navigation costs. To this end we propose a methodology composed of five main steps: (i) speed-invariant inertial transformation; (ii) roughness level classification; (iii) navigation cost estimation; (iv) sensor fusion through Deep Learning; and (v) estimation of navigation costs for untraveled regions. To validate the methodology, we carried out experiments using ground robots in different outdoor environments with different terrain characteristics. Results show that the inertial data transformation reduces the dispersion of signal magnitude for different speeds and scenarios. Meanwhile, the roughness level classification achieved a mean accuracy of 95.4%, for the speed of 0.6 m / s . Finally, the obtained terrain maps are a faithful representation of outdoor environments allowing accurate and reliable path planning.
更多查看译文
关键词
Outdoor terrain mapping,Navigation cost,Traversability,Convolutional neural networks,Field robotics
AI 理解论文
溯源树
样例
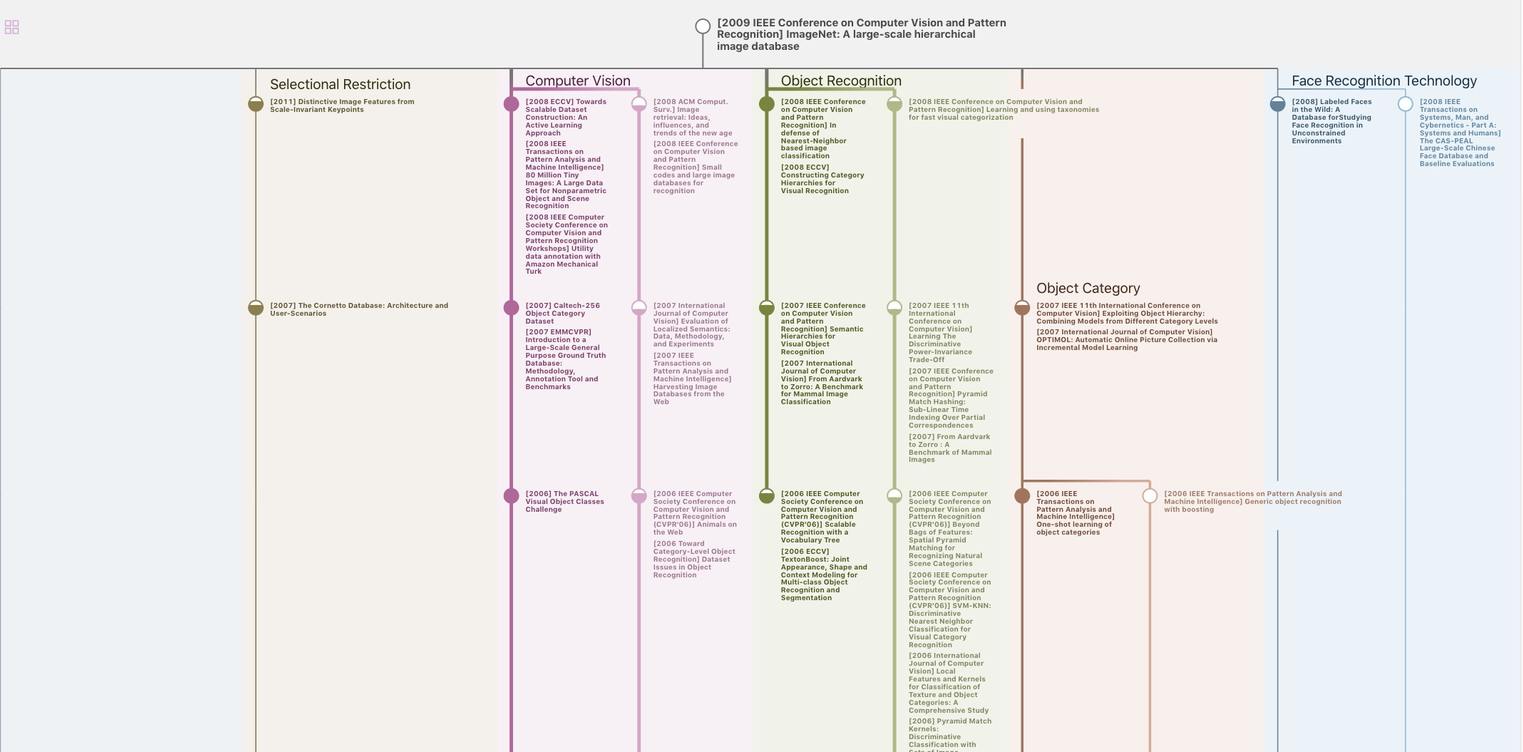
生成溯源树,研究论文发展脉络
Chat Paper
正在生成论文摘要