Salient Features, Data And Algorithms For Microrna Screening From Plants: A Review On The Gains And Pitfalls Of Machine Learning Techniques
CURRENT BIOINFORMATICS(2020)
摘要
The era of big data and high-throughput genomic technology has enabled scientists to have a clear view of plant genomic profiles. However, it has also led to a massive need for computational tools and strategies to interpret this data. In this scenario of huge data inflow, machine learning (ML) approaches are emerging to be the most promising for analysing heterogeneous and unstructured biological datasets. Extending its application to healthcare and agriculture, ML approaches are being useful for microRNA (miRNA) screening as well. Identification of miRNAs is a crucial step towards understanding post-transcriptional gene regulation and miRNA-related pathology. The use of ML tools is becoming indispensable in analysing such data and identifying species-specific, non-conserved miRNA. However, these techniques have their own benefits and lacunas. In this review, we will discuss the current scenario and pitfalls of ML-based tools for plant miRNA identification and provide some insights into the important features, the need for deep learning models and direction in which studies are needed.
更多查看译文
关键词
microRNA, dataset, algorithm, feature selection, plant genomics, machine learning
AI 理解论文
溯源树
样例
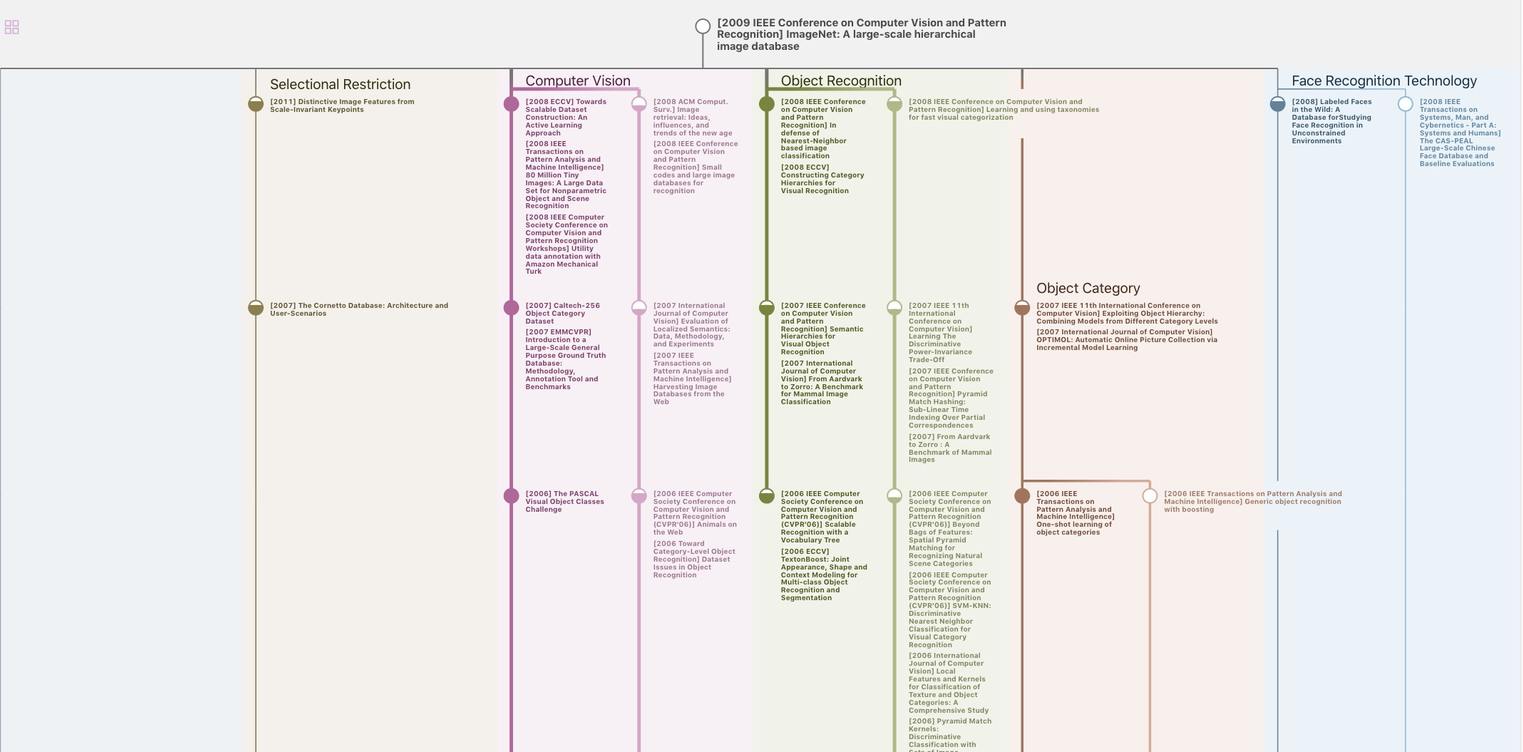
生成溯源树,研究论文发展脉络
Chat Paper
正在生成论文摘要