ITERATIVELY REWEIGHTED FGMRES AND FLSQR FOR SPARSE RECONSTRUCTION
SIAM JOURNAL ON SCIENTIFIC COMPUTING(2021)
摘要
This paper presents two new algorithms to compute sparse solutions of large-scale linear discrete ill-posed problems. The proposed approach consists in constructing a sequence of quadratic problems approximating an l(2)-l(1) regularization scheme (with additional smoothing to ensure differentiability at the origin) and partially solving each problem in the sequence using flexible Krylov-Tikhonov methods. These algorithms are built upon a new solid theoretical justification that guarantees that the sequence of approximate solutions to each problem in the sequence converges to the solution of the considered modified version of the l(2)-l(1) problem. Compared to other traditional methods, the new algorithms have the advantage of building a single (flexible) approximation (Krylov) subspace that encodes regularization through variable "preconditioning" and that is expanded as soon as a new problem in the sequence is defined. Links between the new solvers and other well-established solvers based on augmenting Krylov subspaces are also established. The performance of these algorithms is shown through a variety of numerical examples modeling image deblurring and computed tomography.
更多查看译文
关键词
Krylov methods, flexible Krylov methods, augmented Krylov methods, sparse reconstruction, inverse problems, imaging problems
AI 理解论文
溯源树
样例
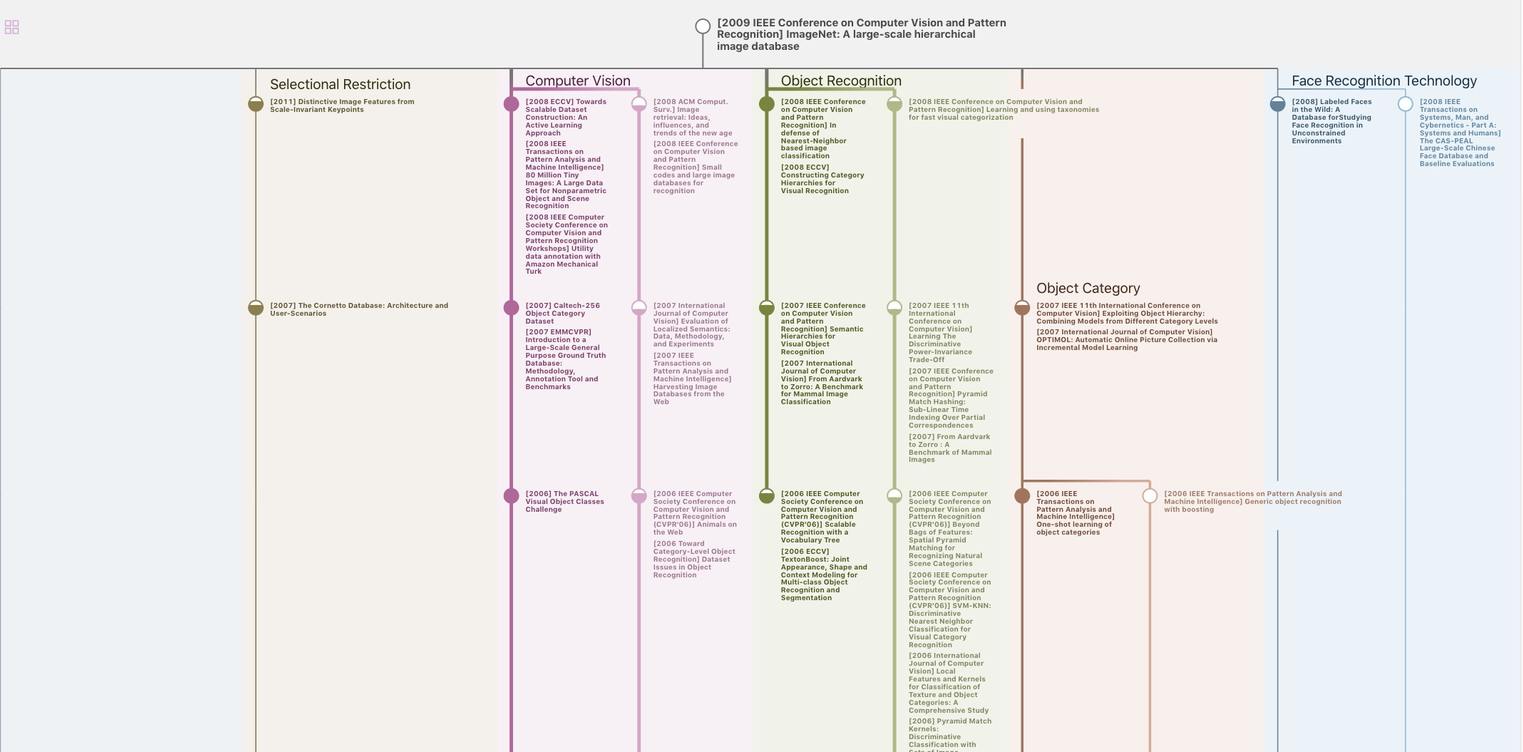
生成溯源树,研究论文发展脉络
Chat Paper
正在生成论文摘要