An attempt to analyse Iterative Data Snooping and L1-norm based on Monte Carlo simulation in the context of leveling networks
SURVEY REVIEW(2022)
摘要
The goal of this paper is to evaluate the outlier identification performance of iterative Data Snooping (IDS) and L-1-norm in levelling networks by considering the redundancy of the network, number and size of the outliers. For this purpose, several Monte-Carlo experiments were conducted into three different levelling networks configurations. In addition, a new way to compare the results of IDS based on Least Squares (LS) residuals and robust estimators such as the L-1-norm has also been developed and presented. From the perspective of analysis only according to the success rate, it is shown that L-1-norm performs better than IDS for the case of networks with low redundancy ((r) over bar < 0.5), especially for cases where more than one outlier is present in the dataset. In the relationship between false positive rate and outlier identification success rate, however, IDS performs better than L-1-norm, independently of the levelling network configuration, number and size of outliers.
更多查看译文
关键词
Data snooping, L-1-norm, Outliers, Levelling network success rate, False positive rate
AI 理解论文
溯源树
样例
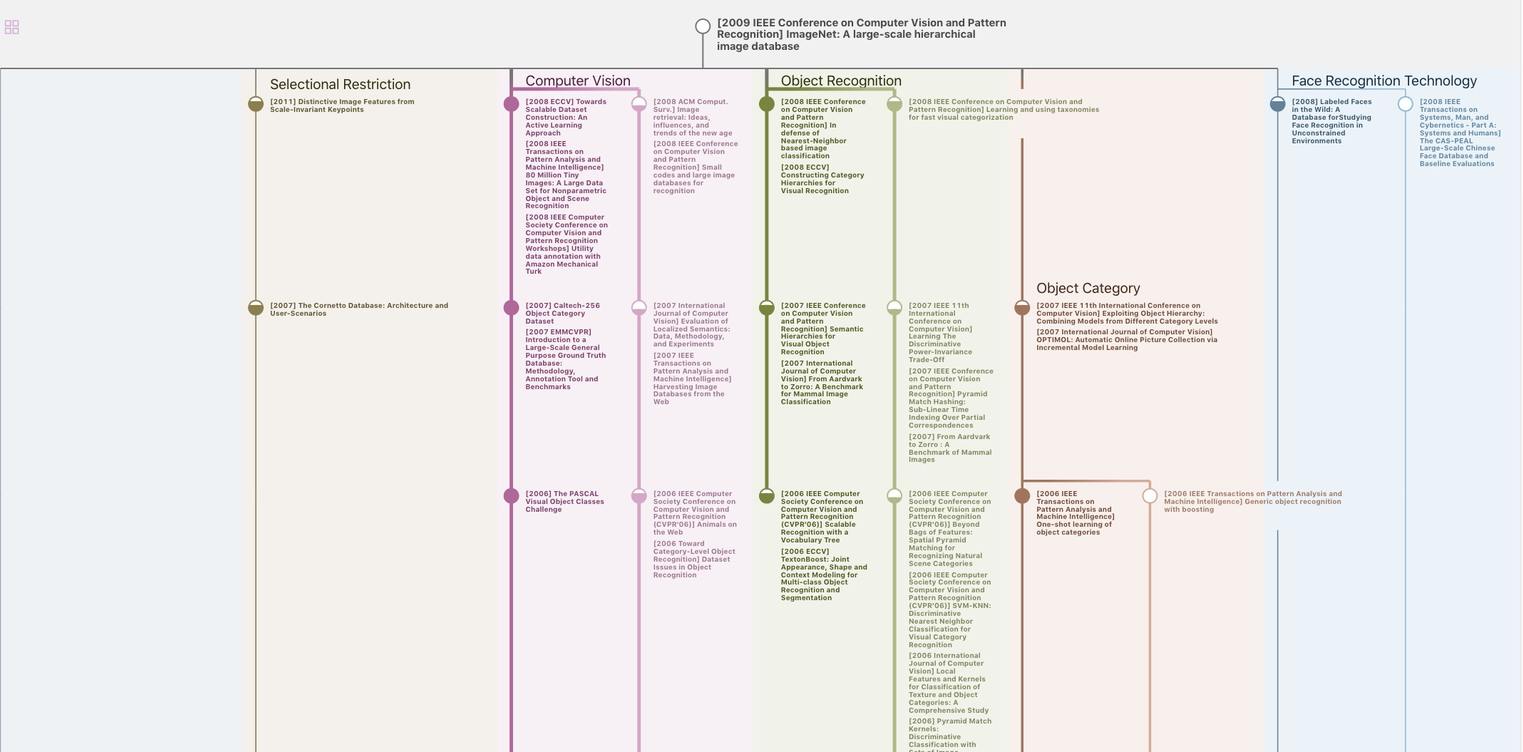
生成溯源树,研究论文发展脉络
Chat Paper
正在生成论文摘要