Sparse Non-Negative Transition Subspace Learning For Image Classification
SIGNAL PROCESSING(2021)
摘要
Recently many variations of least squares regression (LSR) have been developed to address the problem of over-fitting that widely exists in the task of image classification. Among these methods, the most prevalent two means, such as label relaxation and graph manifold embedding, have been demonstrated to be highly effective. In this paper, we present a new strategy named sparse non-negative transition subspace learning (SN-TSL) based least squares regression algorithm which aims to avoid over-fitting by learning a transition subspace between the multifarious high-dimensional inputs and low-dimensional binary labels. Moreover, considering the final regression targets are sparse binary positive matrices, we use the l(1)-norm and the non-negativity constraint to enforce the transition subspace to be sparse and non-negative. The resulting subspace features can be viewed as intermediate representations between the inputs and labels. Because SN-TSL can simultaneously learn two projection matrices in one regression model and the dimensionality of the transition subspace can be set to any integer, SN-TSL has the potential to obtain more distinct projections for classification. It is also suitable for classification problems involving a small number of classes. Extensive experiments on public datasets have shown the proposed SN-TSL outperforms other state-of-the-art LSR based image classification methods. (C) 2021 Published by Elsevier B.V.
更多查看译文
关键词
Transition subspace learning, Over-fitting, Least squares regression, Image classification
AI 理解论文
溯源树
样例
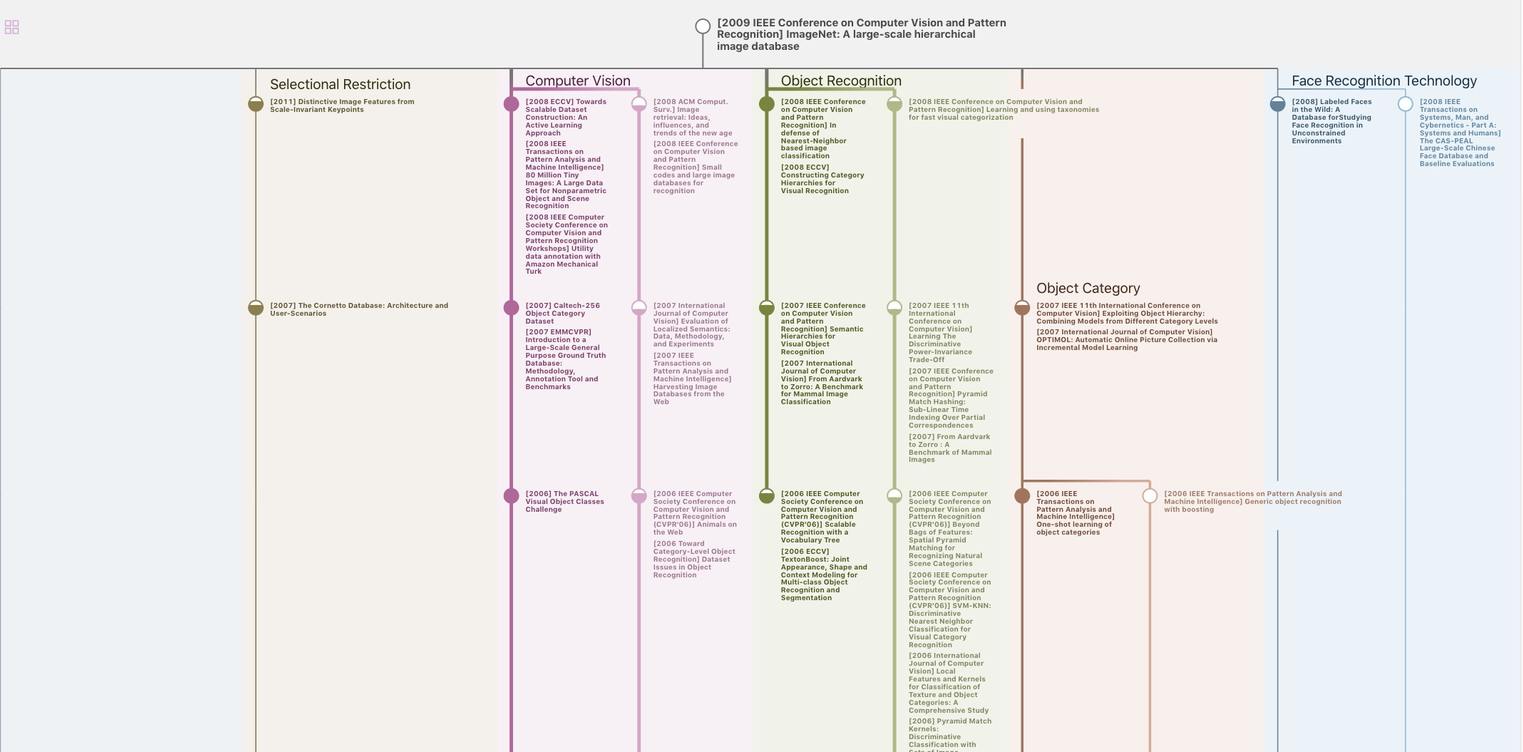
生成溯源树,研究论文发展脉络
Chat Paper
正在生成论文摘要