Degradation-Aware Remaining Useful Life Prediction With Lstm Autoencoder
IEEE TRANSACTIONS ON INSTRUMENTATION AND MEASUREMENT(2021)
摘要
The remaining useful life (RUL) prediction plays a pivotal role in the predictive maintenance of industrial manufacturing systems. However, one major problem with the existing RUL estimation algorithms is the assumption of a single health degradation trend for different machine health stages. To improve the RUL prediction accuracy with various degradation trends, this article proposes an algorithm dubbed degradation-aware long short-term memory (LSTM) autoencoder (AE) (DELTA). First, the Hilbert transform is adopted to evaluate the degradation stage and factor with the real-time sensory signal. Second, we adopt LSTM AE to predict RUL based on multisensor time-series data and the degradation factor. Distinct from the existing studies, the proposed framework is able to dynamically model the degradation factor and explore latent variables to improve RUL prediction accuracy. The performance of DELTA is evaluated with the open-source FEMTO bearing data set. Compared with the existing algorithms, DELTA achieves appreciable improvements in the RUL prediction accuracy.
更多查看译文
关键词
Industrial internet-of-things (IIoT), long short-term memory (LSTM) autoencoder (AE), prognostic technique, remaining useful life (RUL)
AI 理解论文
溯源树
样例
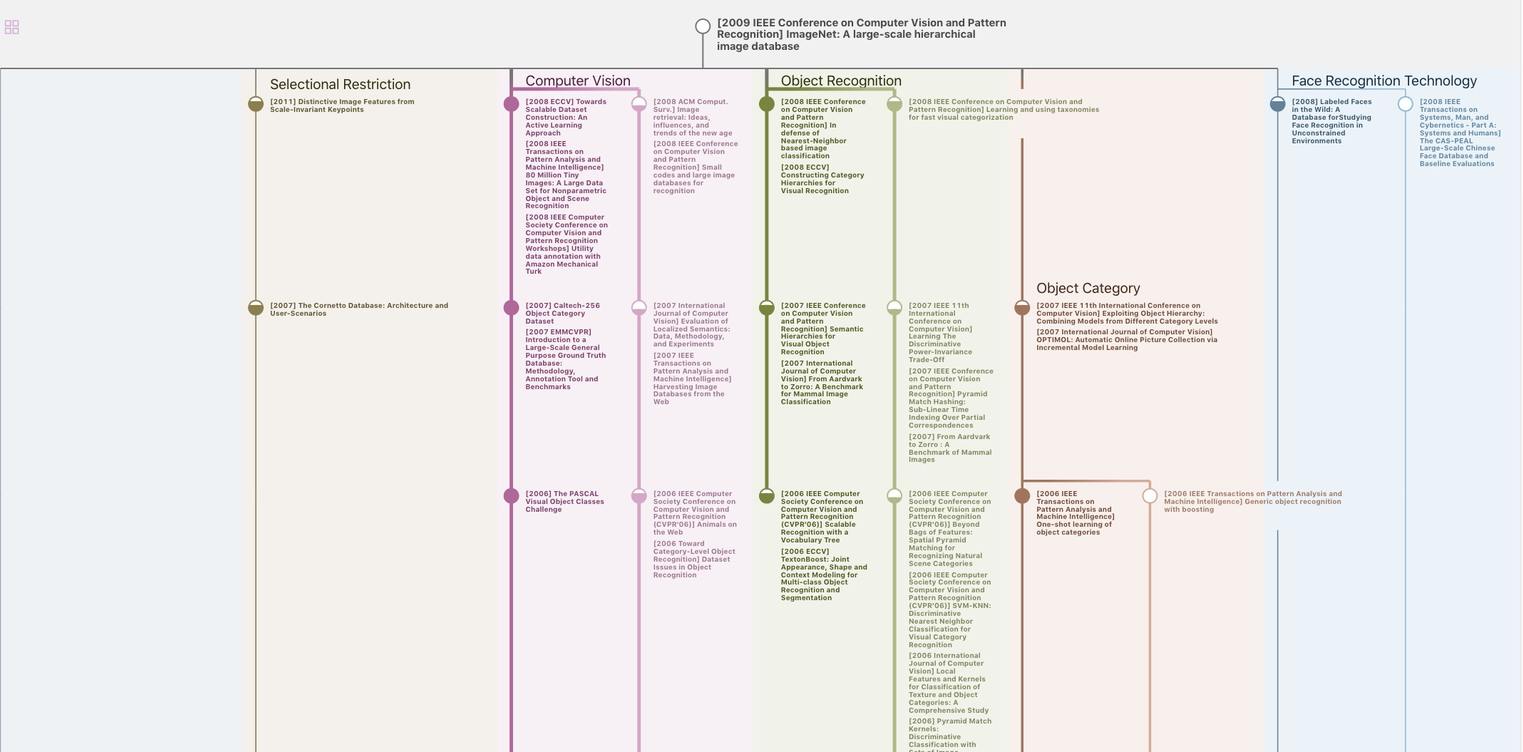
生成溯源树,研究论文发展脉络
Chat Paper
正在生成论文摘要