Neurosymbolic Spike Concept Learner Towards Neuromorphic General Intelligence
ICAART: PROCEEDINGS OF THE 13TH INTERNATIONAL CONFERENCE ON AGENTS AND ARTIFICIAL INTELLIGENCE - VOL 2(2021)
摘要
Current research in the area of concept learning makes use of deep learning and ensembles methods to learn concepts. Concept learning allows us to combine heterogeneous entities in data which could collectively identify as individual concepts. Heterogeneity and compositionality are crucial areas to explore in machine learning as it has the potential to contribute profoundly to artificial general intelligence. We investigate the use of spiking neural networks for concept learning. Spiking neurones inclusively model the temporal properties as observed in biological neurones. A benefit of spike-based neurones allows for localised learning rules that only adapts connections between relevant neurones. In this position paper, we propose a technique allowing dynamic formation of synapse (connections) in spiking neural networks, the basis of structural plasticity. Achieving dynamic formation of synapse allows for a unique approach to concept learning with a malleable neural structure. We call this technique Neurosymbolic Spike-Concept Learner (NS-SCL). The limitations of NS-SCL can be overcome with the neuromorphic computing paradigm. Furthermore, introducing NS-SCL as a technique on neuromorphic platforms should motivate a new direction of research towards Neuromorphic General Intelligence (NGI), a term we define to some extent.
更多查看译文
关键词
Neuromorphic General Intelligence, Spiking Neural Networks, Functional Plasticity, Structural Plasticity, Neurosymbolic, Representation Learning, Concept Learning
AI 理解论文
溯源树
样例
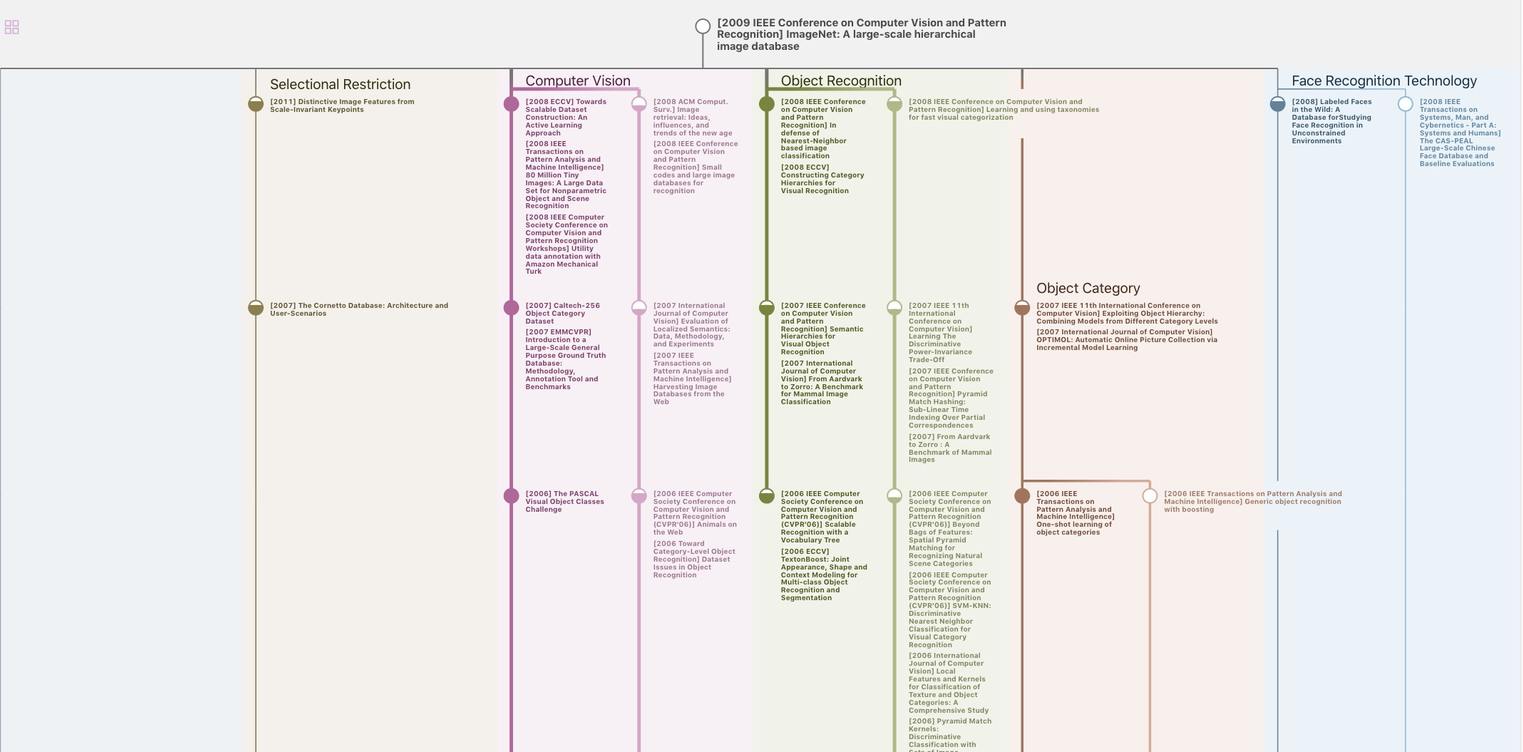
生成溯源树,研究论文发展脉络
Chat Paper
正在生成论文摘要