A blocking scheme for dimension-robust Gibbs sampling in large-scale image deblurring
INVERSE PROBLEMS IN SCIENCE AND ENGINEERING(2021)
摘要
Among the most significant challenges with using Markov chain Monte Carlo (MCMC) methods for sampling from the posterior distributions of Bayesian inverse problems is the rate at which the sampling becomes computationally intractable, as a function of the number of estimated parameters. In image deblurring, there are many MCMC algorithms in the literature, but few attempt reconstructions for images larger than 512 x 512 pixels (10(5) parameters). In quantitative X-ray radiography, used to diagnose dynamic materials experiments, the images can be much larger, leading to problems with millions of parameters. We address this issue and construct a Gibbs sampler via a blocking scheme that leads to a sparse and highly structured posterior precision matrix. The Gibbs sampler naturally exploits the special matrix structure during sampling, making it `dimension-robust', so that its mixing properties are nearly independent of the image size, and generating one sample is computationally feasible. The dimension-robustness enables the characterization of posteriors for large-scale image deblurring problems on modest computational platforms. We demonstrate applicability of this approach by deblurring radiographs of size 4096 x 4096 pixels (10(7) parameters) taken at the Cygnus Dual Beam X-ray Radiography Facility at the U.S. Department of Energy's Nevada National Security Site.
更多查看译文
关键词
Deconvolution, Markov chain Monte Carlo, Gibbs sampling
AI 理解论文
溯源树
样例
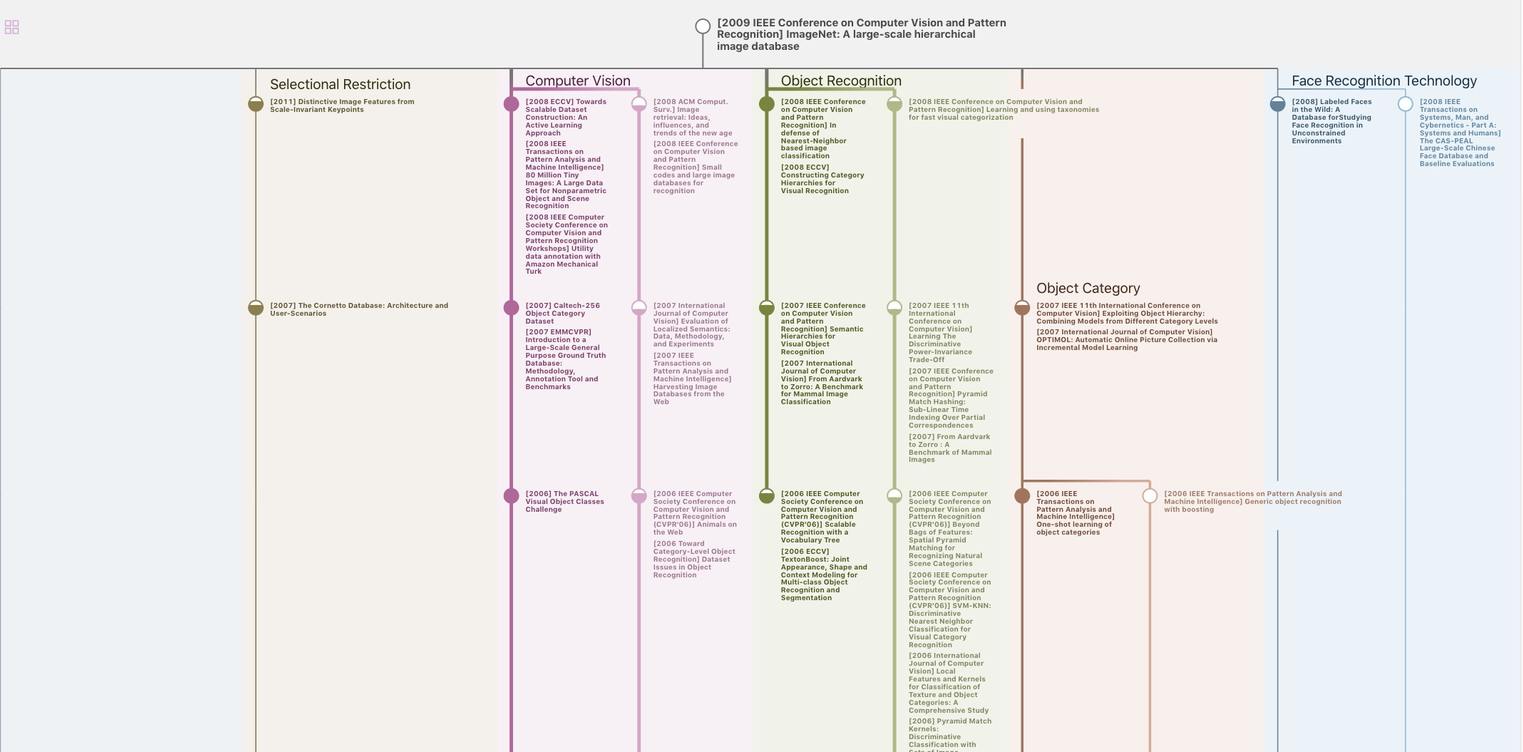
生成溯源树,研究论文发展脉络
Chat Paper
正在生成论文摘要