Global-Local Label Correlation for Partial Multi-Label Learning
IEEE Transactions on Multimedia(2022)
摘要
Partial Multi-label Learning (PML) addresses the scenario where each instance is assigned with multiple candidate labels, while only a subset of the labels are relevant. This task is very challenging because the training procedure can be misguided by the noisy (irrelevant) labels. Exploiting label correlations is useful for partial multi-label learning. However, the existing PML methods often ignore to explicitly and sufficiently leverage the label correlation information for handling the noisy labels. To this end, in this paper, we propose a novel
Global-Local Label Correlation (GLC) approach for partial multi-label learning
. On one hand, we introduce a label coefficient matrix to explicitly exploit the global structure information of labels from multiple subspaces. On the other hand, we present a new label manifold regularizer to capture the local label correlations to further improve the performance of our method. By jointly taking advantage of the global and local label correlations, our proposed approach achieves superior performance on both the synthetic and real-world data sets from diverse domains.
更多查看译文
关键词
Partial multi-label learning,label correlations,label coefficient matrix,label manifold
AI 理解论文
溯源树
样例
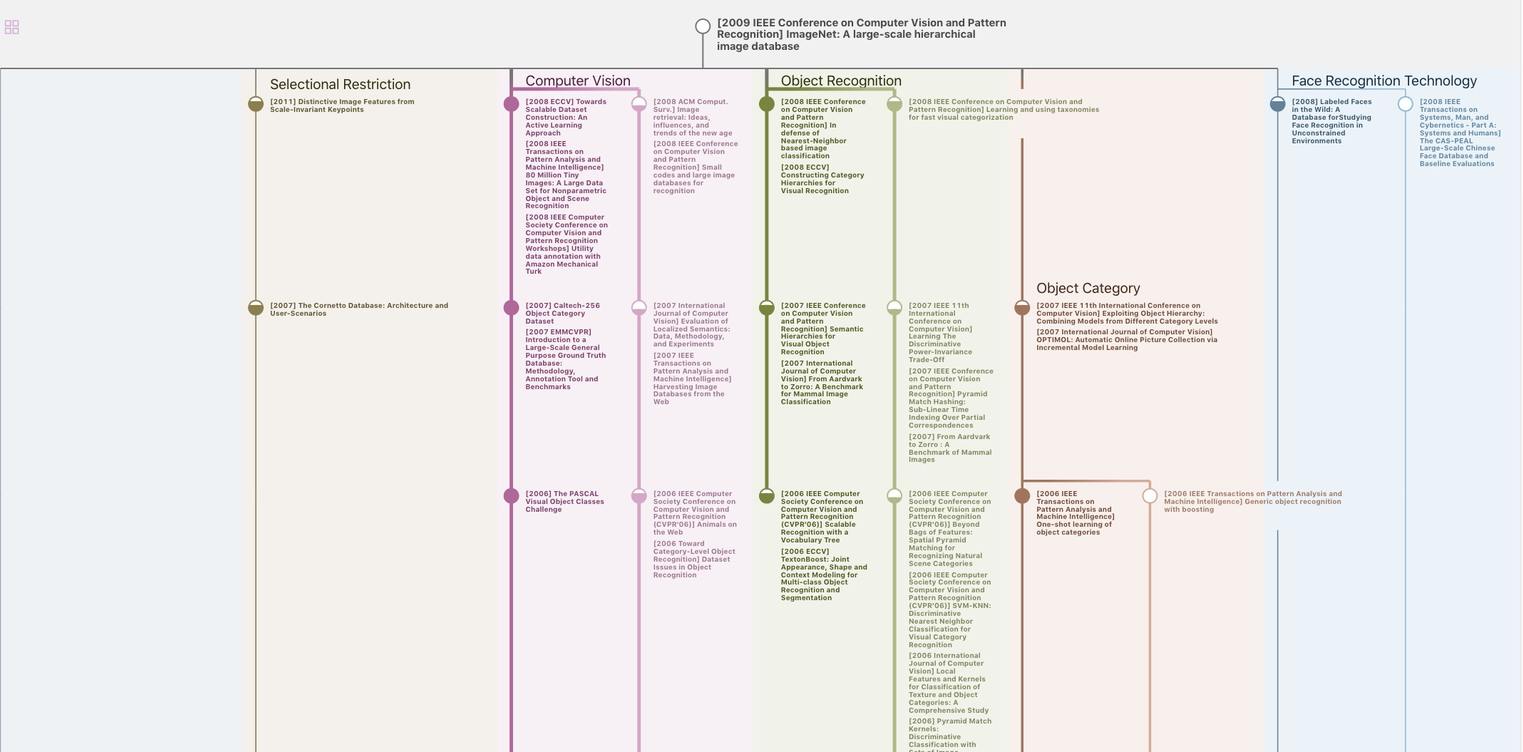
生成溯源树,研究论文发展脉络
Chat Paper
正在生成论文摘要