An improved approach of task-parameterized learning from demonstrations for cobots in dynamic manufacturing
Journal of Intelligent Manufacturing(2021)
摘要
Task-Parameterized Learning from Demonstrations (TP-LfD) is an intelligent intuitive approach to support collaborative robots (cobots) for various industrial applications. Using TP-LfD, human’s demonstrated paths can be learnt by a cobot for reproducing new paths for the cobot to move along in dynamic situations intelligently. One of the challenges to applying TP-LfD in industrial scenarios is how to identify and optimize critical task parameters of TP-LfD, i.e., frames in demonstrations. To overcome the challenge and enhance the performance of TP-LfD in complex manufacturing applications, in this paper, an improved TP-LfD approach is presented. In the approach, frames in demonstrations are autonomously chosen from a pool of generic visual features. To strengthen computational convergence, a statistical algorithm and a reinforcement learning algorithm are designed to eliminate redundant frames and irrelevant frames respectively. Meanwhile, a B-Spline cut-in algorithm is integrated in the improved TP-LfD approach to enhance the path reproducing process in dynamic manufacturing situations. Case studies were conducted to validate the improved TP-LfD approach and to showcase the advantage of the approach. Owing to the robust and generic capabilities, the improved TP-LfD approach enables teaching a cobot to behavior in a more intuitive and intelligent means to support dynamic manufacturing applications.
更多查看译文
关键词
Learning from demonstration, Reinforcement learning, Collaborative robots
AI 理解论文
溯源树
样例
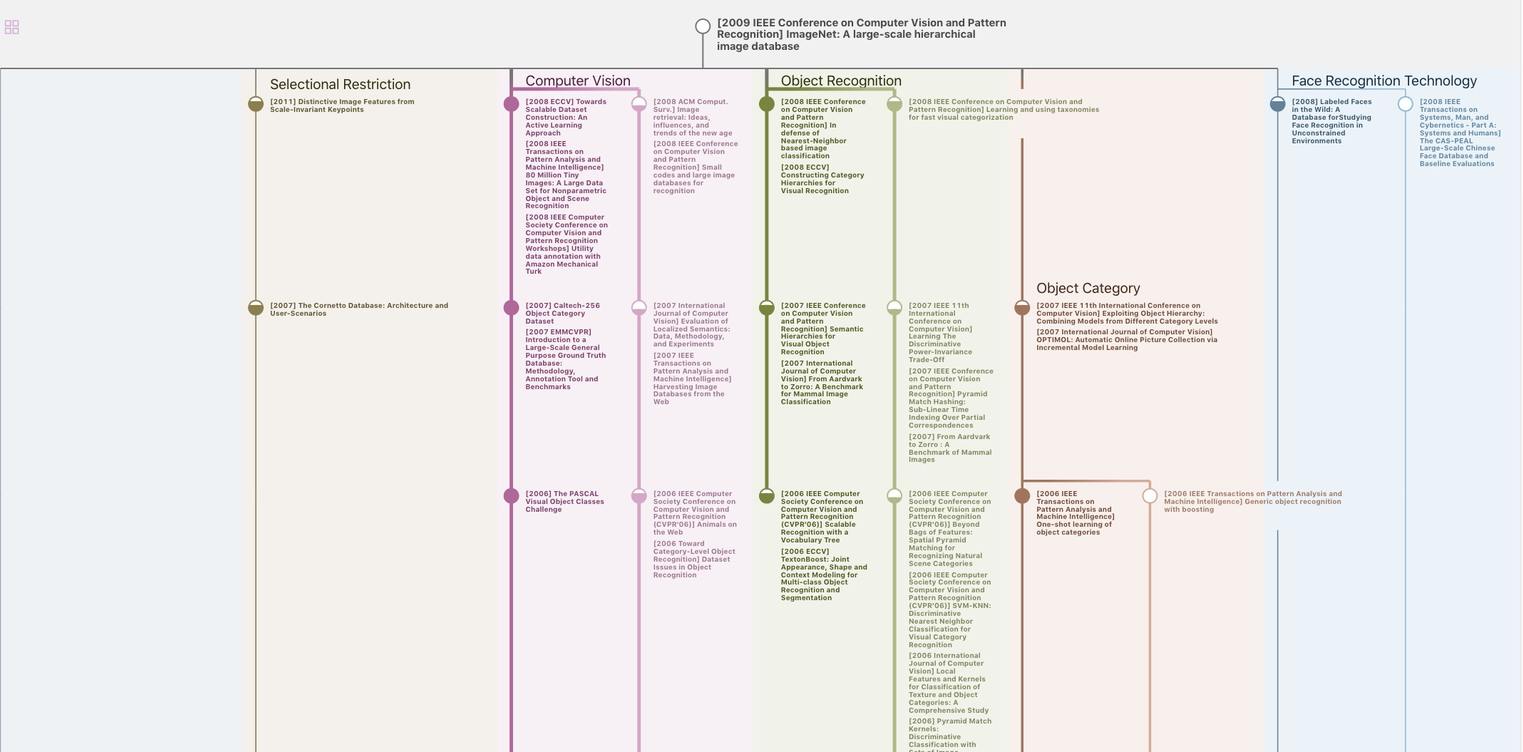
生成溯源树,研究论文发展脉络
Chat Paper
正在生成论文摘要