Social Graph Convolutional Lstm For Pedestrian Trajectory Prediction
IET INTELLIGENT TRANSPORT SYSTEMS(2021)
摘要
Understanding the movement of pedestrians and predicting their future trajectory can be very important in intelligent transportation systems because accurate pedestrian trajectory prediction will improve the level of autonomous driving technology and reduce traffic accidents. The authors address this problem with a social graph convolutional long short-term memory neural network architecture by considering the movement information of each pedestrian and its interaction with neighbours. Specifically, the authors use a graph to model pedestrian walking state where nodes denote the pedestrian movement information, and edges represent the interactions between pairwise pedestrians. An end-to-end architecture that combines a sequence-to-sequence model with a graph convolutional network to learn the movement features and interaction features is used. To capture the interaction influence on different pedestrians, an emotion gate to refine the learned features and filter out useless information is introduced. A companion loss function to increase the ability of the network to capture 'walking in groups' behaviour is further proposed. Through experiments on two public datasets (ETH and UCY), the authors prove that our method outperforms the previous methods.
更多查看译文
关键词
Computer vision and image processing techniques,Traffic engineering computing,Other topics in statistics,Combinatorial mathematics
AI 理解论文
溯源树
样例
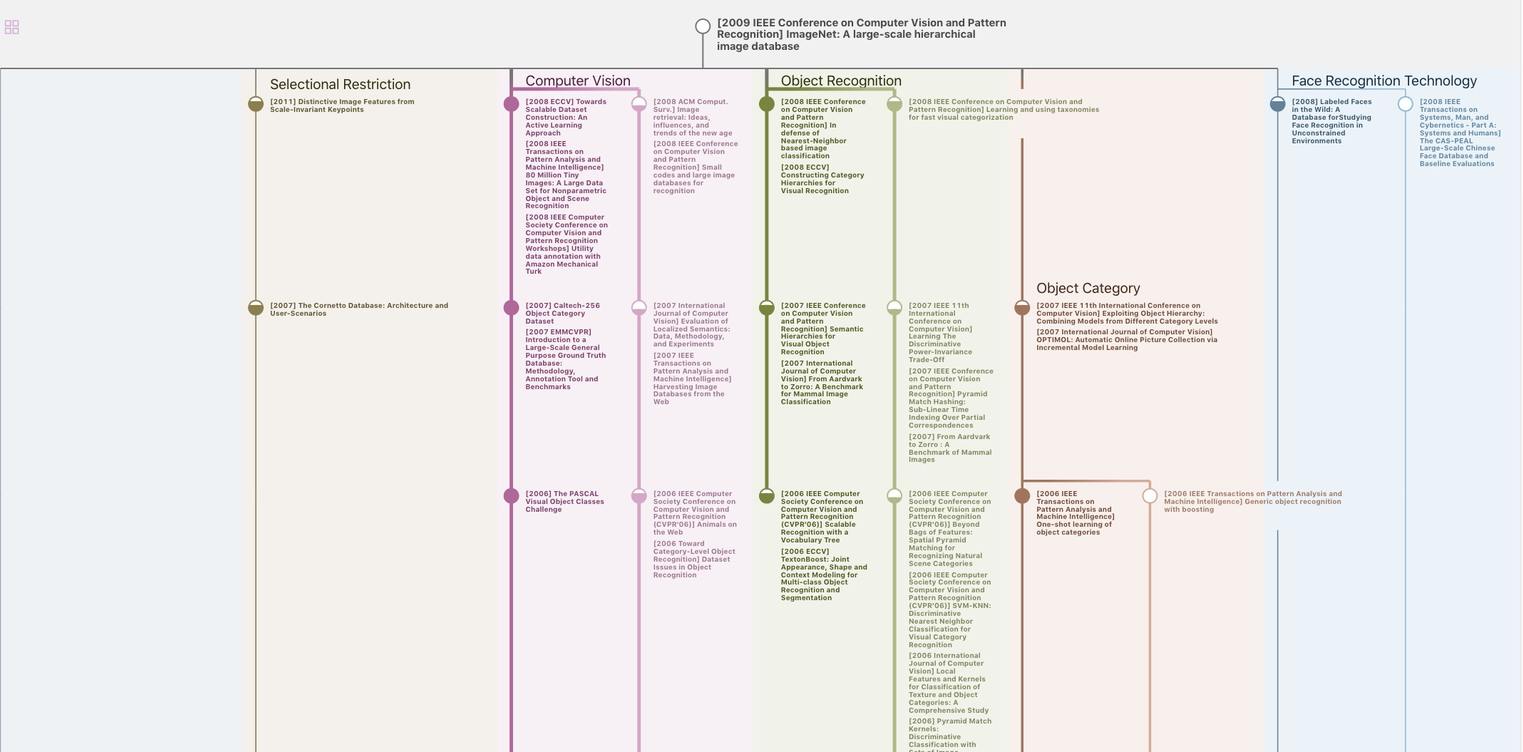
生成溯源树,研究论文发展脉络
Chat Paper
正在生成论文摘要