Semantic Segmentation of Remote-Sensing Images Based on Multiscale Feature Fusion and Attention Refinement
IEEE GEOSCIENCE AND REMOTE SENSING LETTERS(2022)
摘要
In recent years, the automatic extraction of remote-sensing image information has attracted full attention. However, the particularity of remote-sensing images and the scarcity of data sets with label information have brought new challenges to existing methods. Therefore, we develop a lightweight semantic segmentation network based onmultiscale feature fusion (MFF) and attention refinement (MFFANet). Our network relies on three crucial modules for improved performance. The multiscale attention refinement module strengthens the representation ability of feature maps extracted by the deep residual network. The MFF module aggregates the information carried by the high-level and low-level features while restoring the image resolution. Furthermore, the boundary enhancement module captures boundary details to solve the semantic ambiguity problem. We achieve 83.5% mean intersection over union (MIoU) on the Urban Semantic 3-D (US3D) data set and 69.3% MIoU on the Vaihingen data set with only 8.2M parameters.
更多查看译文
关键词
Semantics, Image segmentation, Remote sensing, Feature extraction, Image resolution, Decoding, Training, Attention, feature fusion, multiscale feature, remote-sensing images, semantic segmentation
AI 理解论文
溯源树
样例
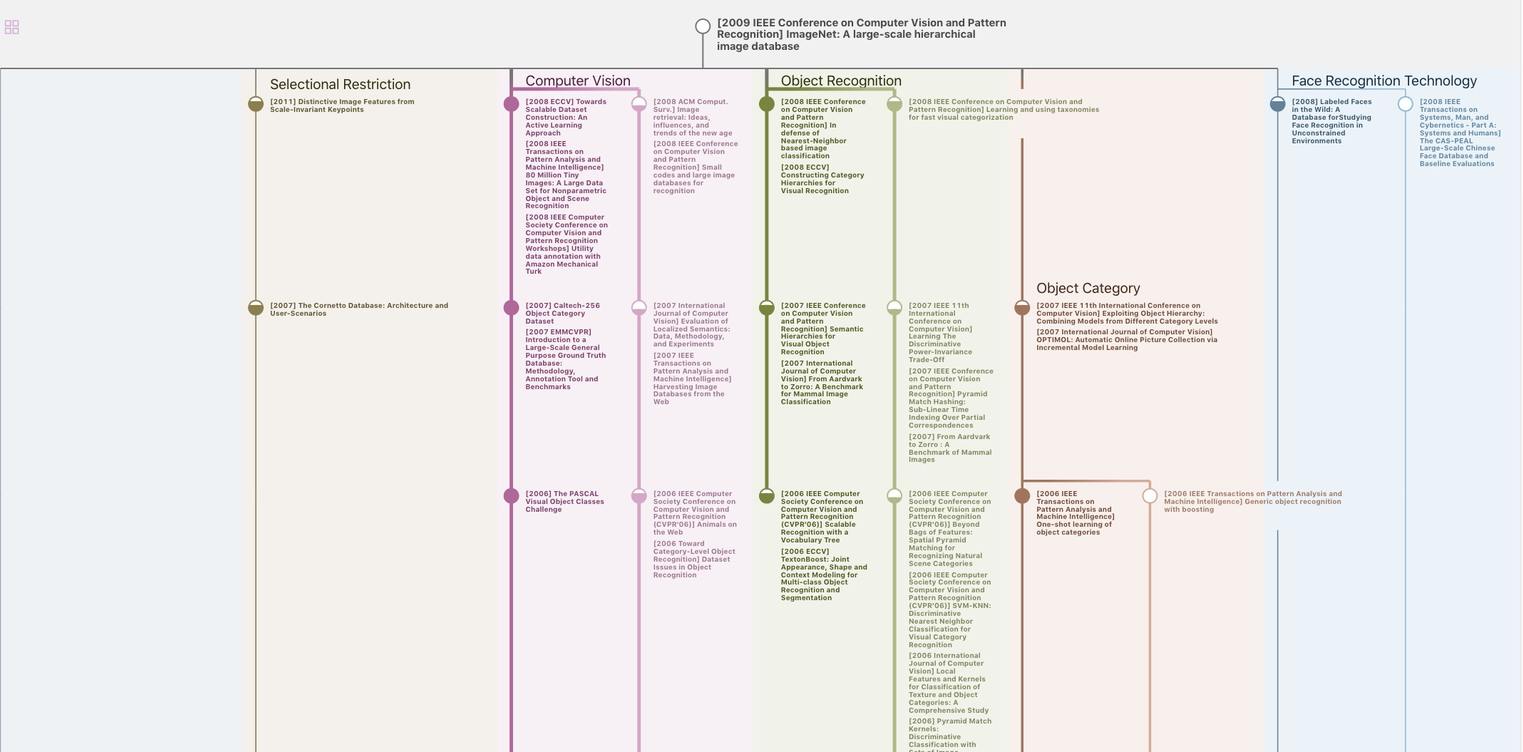
生成溯源树,研究论文发展脉络
Chat Paper
正在生成论文摘要