Deep neural networks architecture driven by problem-specific information
NEURAL COMPUTING & APPLICATIONS(2021)
摘要
Deep learning provides a variety of neural network-based models, known as deep neural networks (DNNs), which are being successfully used in several domains to build highly accurate predictors. A key factor which usually makes DNNs to outperform traditional machine learning models is the amount of data that is nowadays accessible and available. Nevertheless, there are other factors linked to DNNs topologies that may also have influence on the predictive performance of DNN models. In particular, fully connected deep neural networks (fc-DNNs) typically struggle in achieving good performance rates when applied to small datasets. This is due to the high number of parameters which need to be learned when training this kind of models, which makes them prone to over-fitting issues. In this paper, authors propose the use of problem-specific information in order to impose constraints to network architecture so that a fc-DNN is transformed into a partially connected DNN (pc-DNN), in such a way that network topology is driven by prior knowledge. This work compares two baseline models, the elastic net and fc-DNNs, to pc-DNNs applied on three synthetic datasets with different number of samples. Synthetic data was generated to estimate the goodness of using problem-specific information to drive network architectures. Furthermore, a similar analysis is performed herein on a real-world problem dataset to show the benefits of pc-DNN models in term of predictive performance. The results of the analysis showed that pc-DNNs with built-in problem-specific information clearly outperformed the elastic net and fc-DNNs in most of the datasets used, in either synthetic or real-world problems. The pc-DNNs turned out to be a useful model, especially when it is applied to small- or medium-size datasets, on which it significantly outperformed the baseline models considered in this study. Specifically, the pc-DNNs achieved AUC and MSE improvement rates of ( 8.21% , 19.79% ) and ( 6.65% , 20.54% ) in small- and medium-size datasets for both case studies analyzed, the synthetic and real-world problem, respectively.
更多查看译文
关键词
Deep learning, Prior knowledge, Deep neural networks, Data-driven topology, Data-scarce settings, Predictive modeling, Air quality
AI 理解论文
溯源树
样例
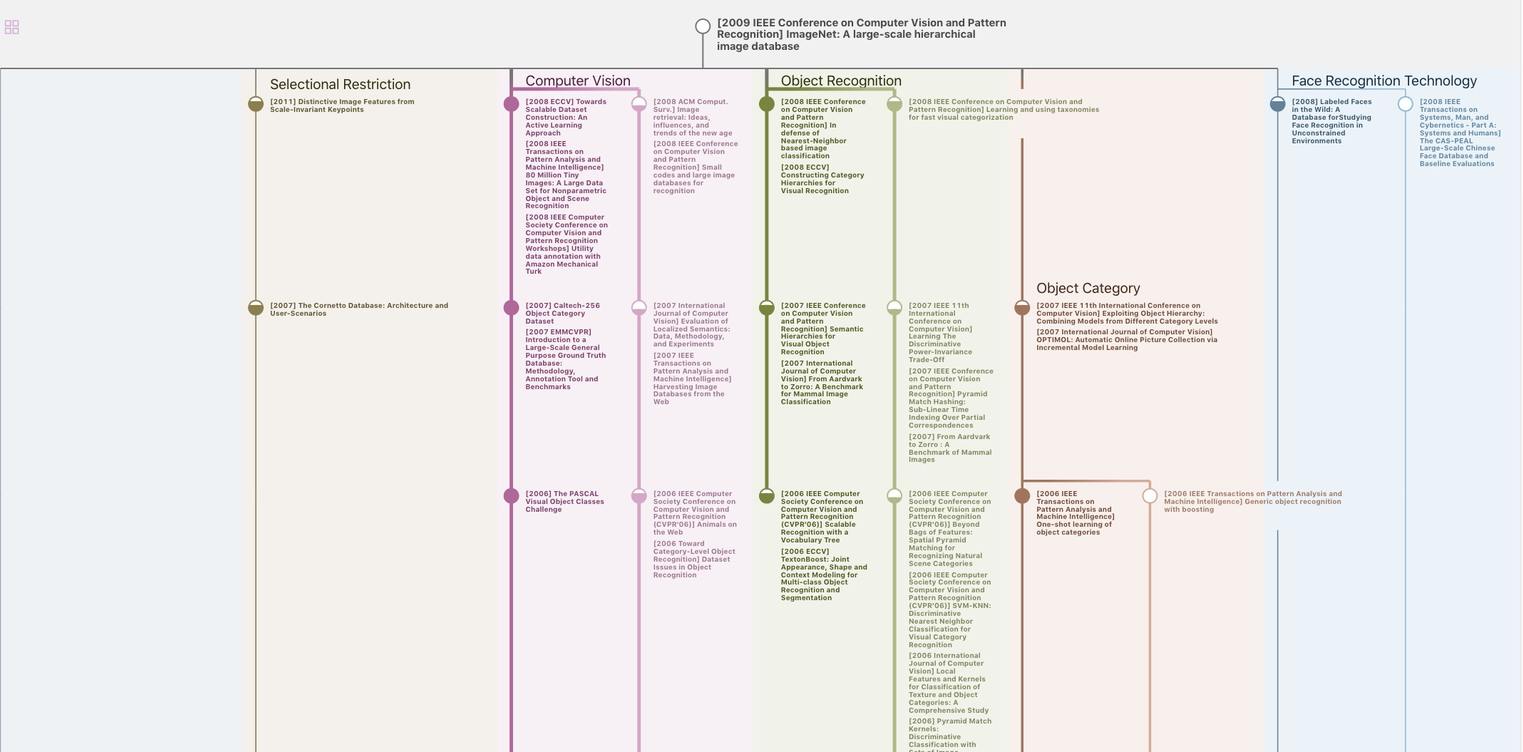
生成溯源树,研究论文发展脉络
Chat Paper
正在生成论文摘要