MLS: An MAE-Aware LiDAR Sampling Framework for On-Road Environments Using Spatio-Temporal Information
IEEE Sensors Journal(2021)
摘要
In recent years, light detection and ranging (LiDAR) sensors have been widely utilized in various applications, including robotics and autonomous driving. However, LiDAR sensors have relatively low resolutions, take considerable time to acquire laser range measurements, and require significant resources to process and store large-scale point clouds. To tackle these issues, many depth image sampling algorithms have been proposed, but their performances are unsatisfactory in complex on-road environments, especially when the sampling rate of measuring equipment is relatively low. Although region-of-interest (ROI)-based sampling has achieved some promising results for LiDAR sampling in on-road environments, the rate of ROI sampling has not been thoroughly investigated, which has limited reconstruction performance. To address this problem, this article proposes a solution to the budget distribution optimization problem to find optimal sampling rates according to the characteristics of each region. A simple yet effective mean absolute error (MAE)-aware model of reconstruction errors was developed and employed to analytically derive optimal sampling rates. In addition, a practical LiDAR sampling framework for autonomous driving was developed. Experimental results demonstrate that the proposed method outperforms all previous approaches in terms of both the object and overall scene reconstruction performances.
更多查看译文
关键词
Autonomous driving,LiDAR sampling,on-road environment,ROI-based sampling
AI 理解论文
溯源树
样例
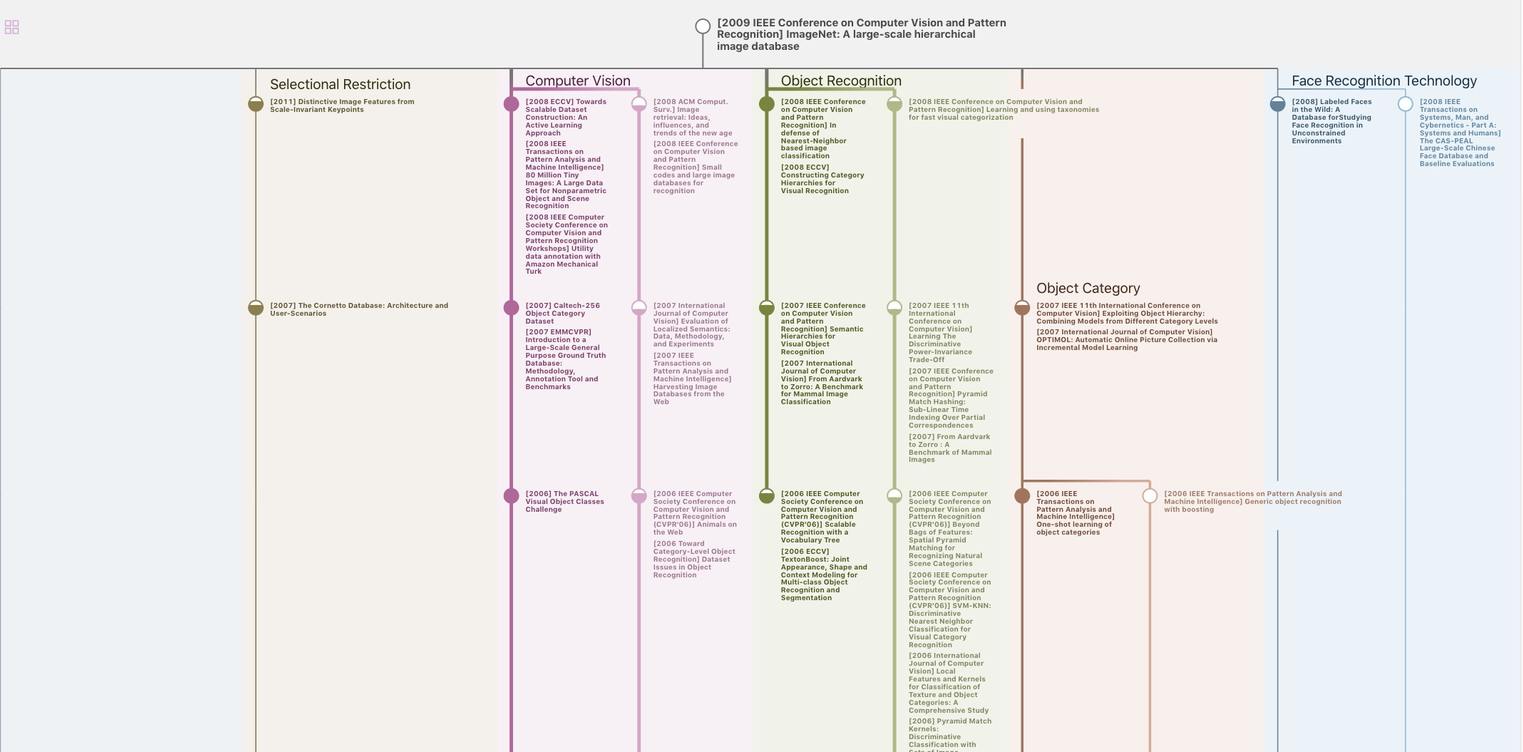
生成溯源树,研究论文发展脉络
Chat Paper
正在生成论文摘要