Explainable AI as a Social Microscope: A Case Study on Academic Performance.
LOD (1)(2020)
摘要
Academic performance is perceived as a product of complex interactions between students’ overall experience, personal characteristics and upbringing. Data science techniques, most commonly involving regression analysis and related approaches, serve as a viable means to explore this interplay. However, these tend to extract factors with wide-ranging impact, while overlooking variations specific to individual students . Focusing on each student’s peculiarities is generally impossible with thousands or even hundreds of subjects, yet data mining methods might prove effective in devising more targeted approaches. For instance, subjects with shared characteristics can be assigned to clusters, which can then be examined separately with machine learning algorithms, thereby providing a more nuanced view of the factors affecting individuals in a particular group. In this context, we introduce a data science workflow allowing for fine-grained analysis of academic performance correlates that captures the subtle differences in students’ sensitivities to these factors . Leveraging the Local Interpretable Model-Agnostic Explanations (LIME) algorithm from the toolbox of Explainable Artificial Intelligence (XAI) techniques, the proposed pipeline yields groups of students having similar academic attainment indicators , rather than similar features (e.g. familial background) as typically practiced in prior studies. As a proof-of-concept case study, a rich longitudinal dataset is selected to evaluate the effectiveness of the proposed approach versus a standard regression model.
更多查看译文
关键词
Explainable AI, LIME, Data science, Machine learning, Computational social science, Academic performance, GPA prediction
AI 理解论文
溯源树
样例
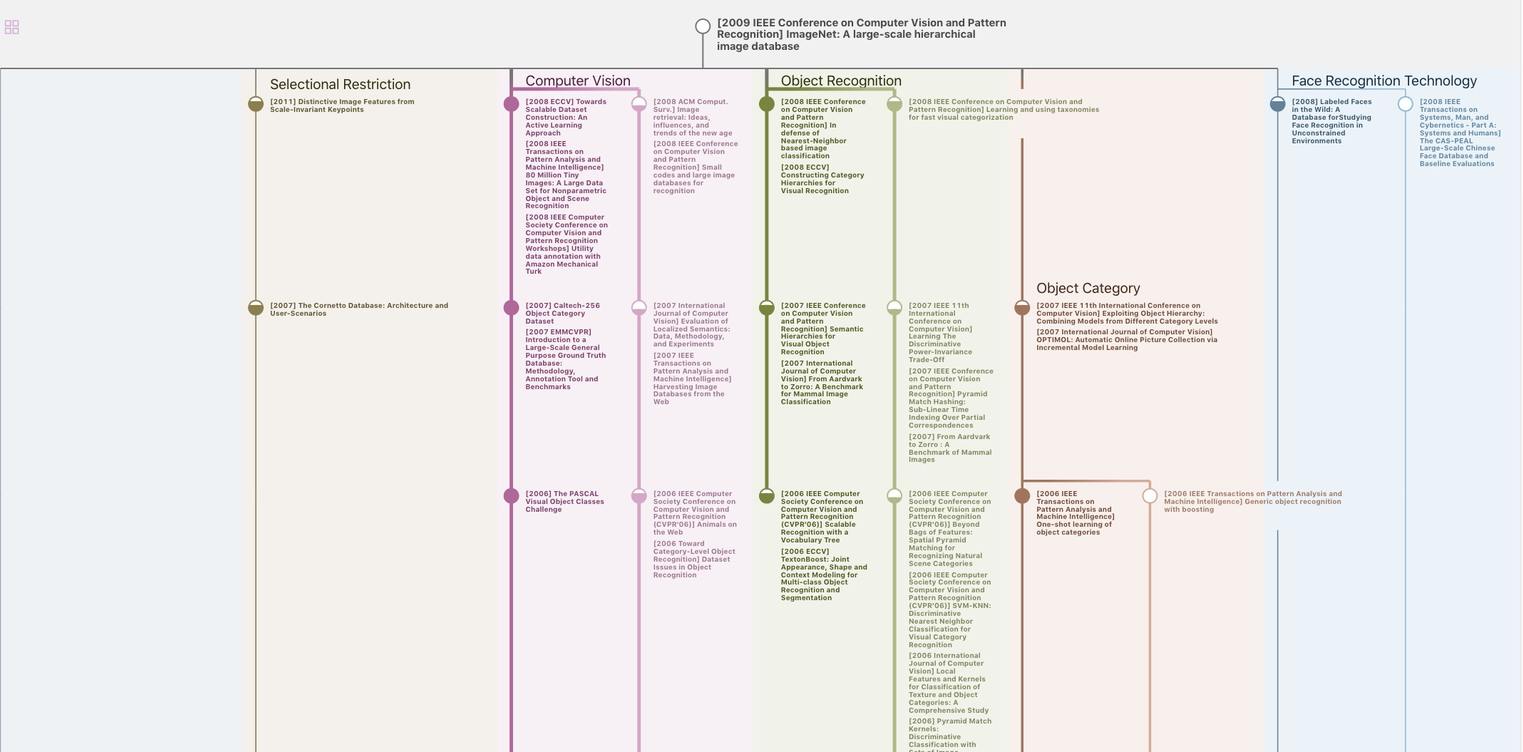
生成溯源树,研究论文发展脉络
Chat Paper
正在生成论文摘要