Novel Radar-Based Gesture Recognition System Using Optimized Cnn-Lstm Deep Neural Network For Low-Power Microcomputer Platform
ICAART: PROCEEDINGS OF THE 13TH INTERNATIONAL CONFERENCE ON AGENTS AND ARTIFICIAL INTELLIGENCE - VOL 2(2021)
摘要
The goal of the embedded hand gesture recognition based on a radar sensor is to improve a human-machine interface, while taking into consideration privacy issues of camera sensors. In addition, the system has to be deployable on a low-power microcomputer for the applicability in broadly defined IoT and smart home solutions. Currently available gesture sensing solutions are ineffective in terms of low-power consumption what prevents them from the deployment on the low-power microcomputers. Recent advances exhibit a potential of deep learning models for a gesture classification whereas they are still limited to high-performance hardware. Embedded microcomputers are constrained in terms of memory, CPU clock speed and GPU performance. These limitations imply a topology design problem that is addressed in this work. Moreover, this research project proposes an alternative signal processing approach - using the continuous wavelet transform, which enables us to see the distribution of frequencies formed by every gesture. The newly proposed neural network topology performs equally well compared to the state of the art neural networks, however it needs only 54.6% of memory and it needs 20% of time to perform inference relative to the state of the art models. This dedicated neural network architecture allows for the deployment on resource constrained microcomputers, thus enabling a human-machine interface implementation on the embedded devices. Our system achieved an overall accuracy of 95.05% on earlier unseen data.
更多查看译文
关键词
Deep Learning, Gesture Recognition, Optimization, Pruning, CNN, LSTM
AI 理解论文
溯源树
样例
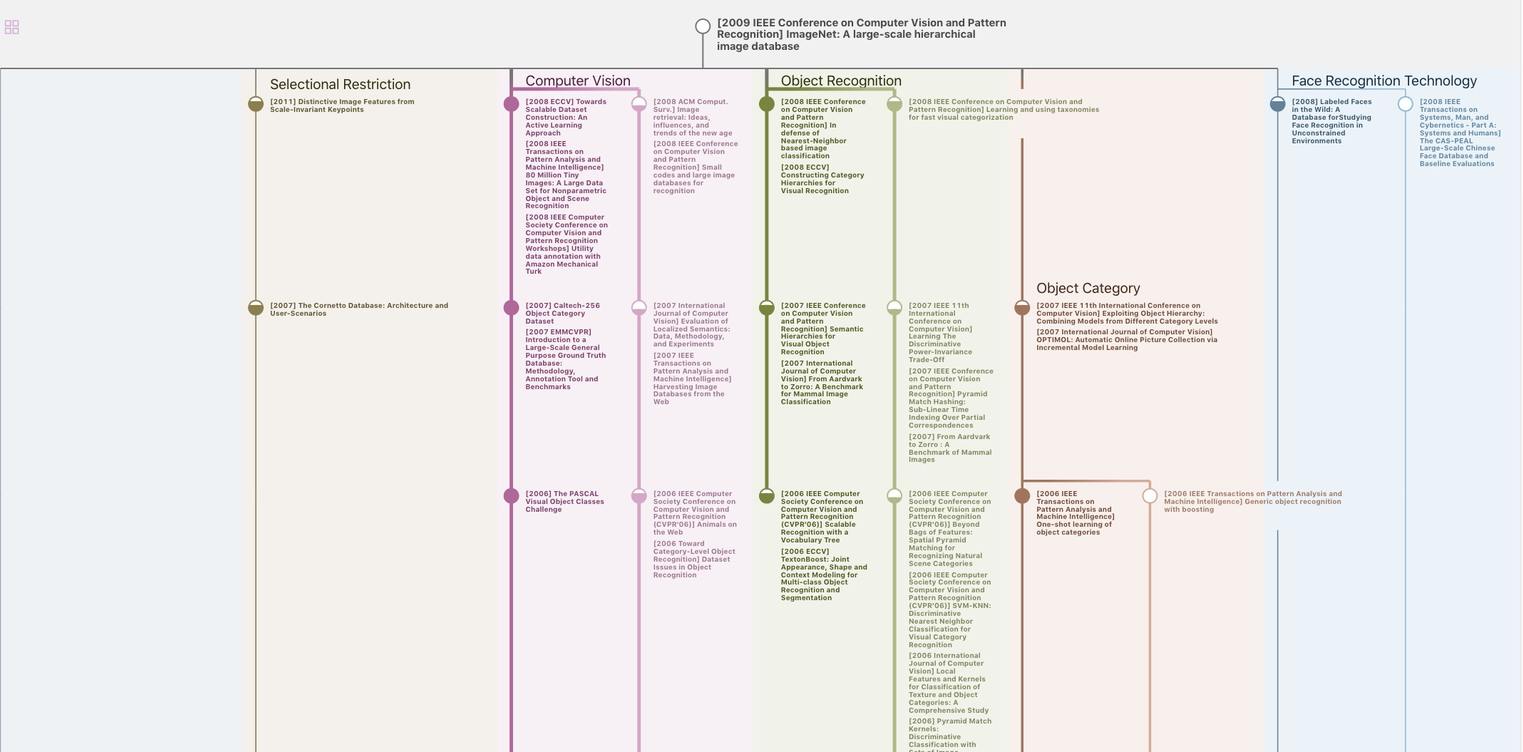
生成溯源树,研究论文发展脉络
Chat Paper
正在生成论文摘要