Estimating Pasture Biomass Using Sentinel-2 Imagery And Machine Learning
REMOTE SENSING(2021)
摘要
Effective dairy farm management requires the regular estimation and prediction of pasture biomass. This study explored the suitability of high spatio-temporal resolution Sentinel-2 imagery and the applicability of advanced machine learning techniques for estimating aboveground biomass at the paddock level in five dairy farms across northern Tasmania, Australia. A sequential neural network model was developed by integrating Sentinel-2 time-series data, weekly field biomass observations and daily climate variables from 2017 to 2018. Linear least-squares regression was employed for evaluating the results for model calibration and validation. Optimal model performance was realised with an R-2 of approximate to 0.6, a root-mean-square error (RMSE) of approximate to 356 kg dry matter (DM)/ha and a mean absolute error (MAE) of 262 kg DM/ha. These performance markers indicated the results were within the variability of the pasture biomass measured in the field, and therefore represent a relatively high prediction accuracy. Sensitivity analysis further revealed what impact each farm's in situ measurement, pasture management and grazing practices have on the model's predictions. The study demonstrated the potential benefits and feasibility of improving biomass estimation in a cheap and rapid manner over traditional field measurement and commonly used remote-sensing methods. The proposed approach will help farmers and policymakers to estimate the amount of pasture present for optimising grazing management and improving decision-making regarding dairy farming.
更多查看译文
关键词
remote sensing, deep learning, digital agriculture, dairy farming, grazing, grassland biomass
AI 理解论文
溯源树
样例
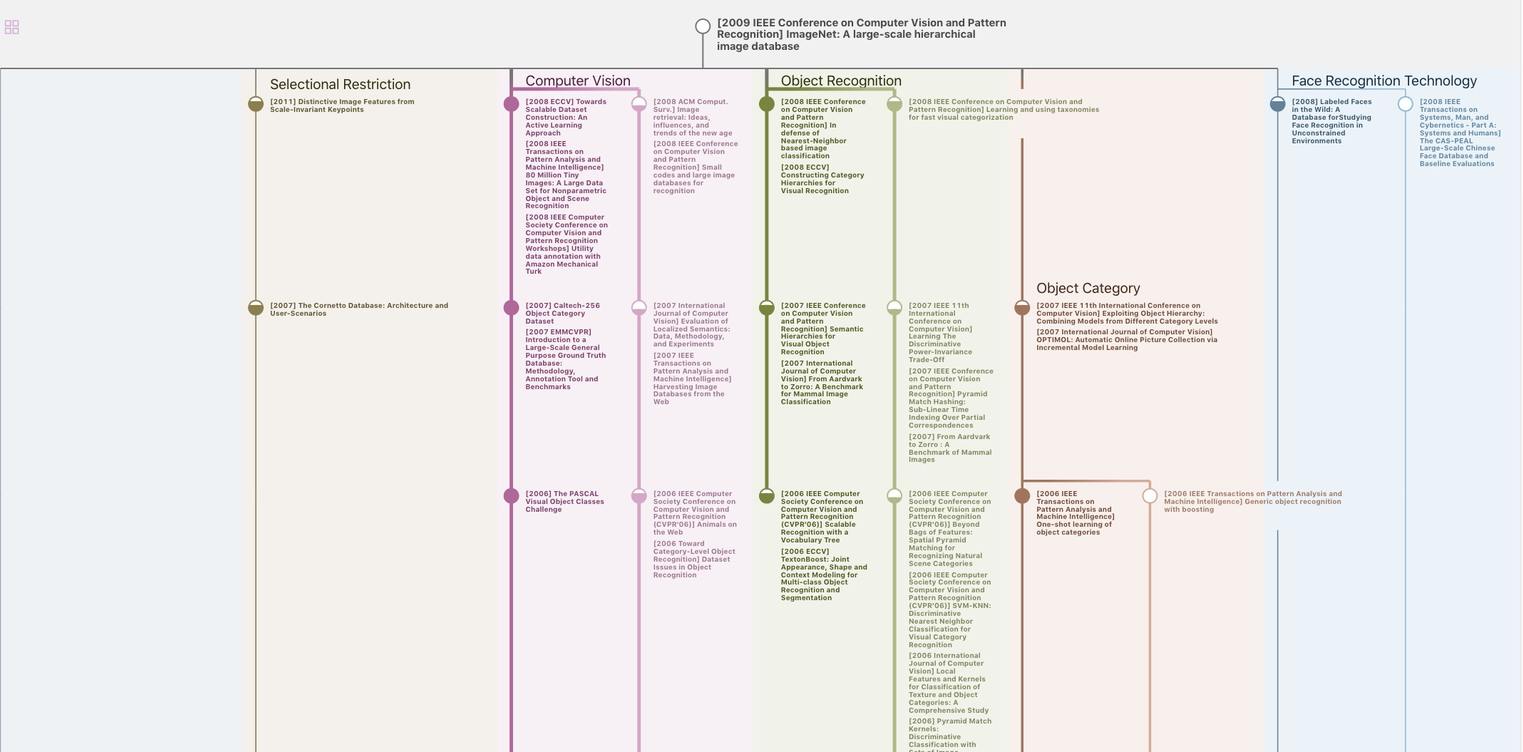
生成溯源树,研究论文发展脉络
Chat Paper
正在生成论文摘要