Underwater Image Enhancement With The Low-Rank Nonnegative Matrix Factorization Method
INTERNATIONAL JOURNAL OF PATTERN RECOGNITION AND ARTIFICIAL INTELLIGENCE(2021)
摘要
Due to the scattering and absorption effects in the undersea environment, underwater image enhancement is a challenging problem. To obtain the ground-truth data for training is also an open problem. So, the learning process is unavailable. In this paper, we propose a Low-Rank Nonnegative Matrix Factorization (LR-NMF) method, which only uses the degraded underwater image as input to generate the more clear and realistic image. According to the underwater image formation model, the degraded underwater image could be separated into three parts, the directed component, the back and forward scattering components. The latter two parts can be considered as scattering. The directed component is constrained to have a low rank. After that, the restored underwater image is obtained. The quantitative and qualitative analyses illustrate that the proposed method performed equivalent or better than the state-of-the-art methods. Yet, it's simple to implement without the training process.
更多查看译文
关键词
Image enhancement, low-rank constraint, matrix factorization
AI 理解论文
溯源树
样例
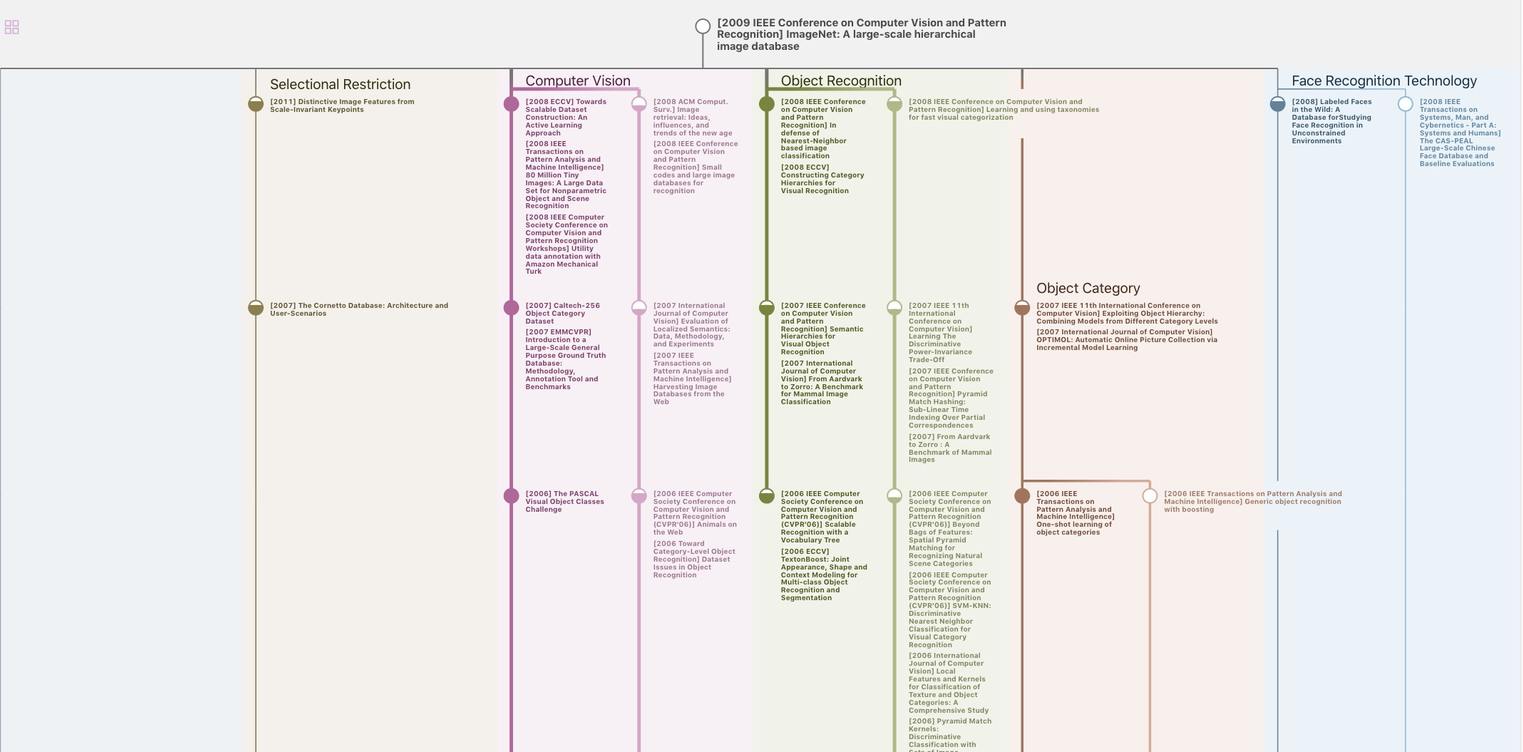
生成溯源树,研究论文发展脉络
Chat Paper
正在生成论文摘要