Material Removal Rate Prediction using the Classification-Regression Approach
2020 IEEE 22nd Electronics Packaging Technology Conference (EPTC)(2020)
摘要
Chemical Mechanical Polishing (CMP) is one of the most critical process step in the fabrication of advanced packages, such as Fanout Wafer Level Packaging (FOWLP). CMP process requires tight and dynamic control of process parameters to achieve polarization, high quality and reliability of organic or in-organic redistribution layer (RDL) surface morphology. Typically, physics based or data driven approaches are implied to predict material removal rate (MRR) and run time control. The former models a closed-form expression between domain knowledge and MRR. Often, the domain knowledge are based on kinetics and contact interaction between the wafer, and the polishing tool. While the latter use time series based training data and machine learning to predict MRR. In this paper, we demonstrate to incorporate wear knowledge as classification and show its effectiveness in predicting MRR. Our experiments shows better overall accuracy being achieved through the proposed classification and regression framework.
更多查看译文
关键词
material removal rate prediction,classification-regression approach,critical process step,advanced packages,fanout wafer level packaging,FOWLP,CMP process,dynamic control,process parameters,reliability,in-organic redistribution layer surface morphology,data driven approaches,MRR,closed-form expression,domain knowledge,contact interaction,polishing tool,use time series based training data,wear knowledge,regression framework,FOWLP
AI 理解论文
溯源树
样例
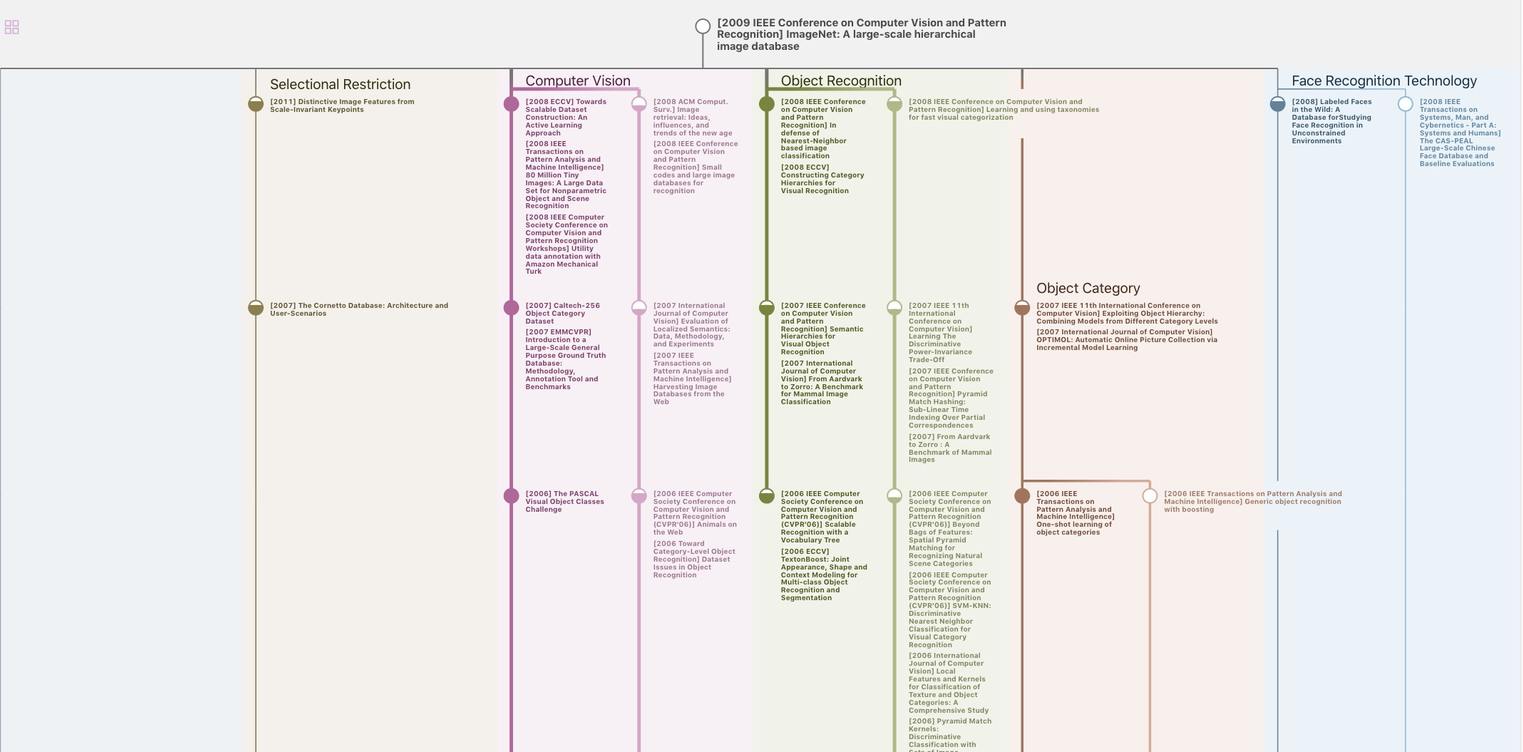
生成溯源树,研究论文发展脉络
Chat Paper
正在生成论文摘要