Real-time foreground object segmentation networks using long and short skip connections
Information Sciences(2021)
摘要
Foreground object segmentation is an important task with various applications in outdoor surveillance and navigation. Most existing methods focus on accuracy and therefore, are computationally expensive and low in speed, making them difficult to use in actual applications. In this study, we aim to address the issue of accuracy and efficiency trade-off. In particular, in contrast with existing methods that use fine-tuning routine on heavyweight pretrained models and/or optimization techniques to enhance results, we propose a lightweight end-to-end network that can be trained from scratch effectively and efficiently. First, long and short skip connections are used among convolutional blocks and within the bottleneck block. By doing so, information flow within the networks is enhanced during the training stage, and thus, the use rate of parameters in the model is increased, allowing a more compact and efficient network design. Second, we use feature fusions based on element-wise summing before each up-sampling layer to reduce the size of the decoder, accelerate the up-sampling process, and stabilize training convergence. Our proposed method is tested rigorously. In particular, we achieved 1000 times higher speed compared with state-of-the-art methods on CD2014 and SBI2015 datasets with comparable accuracy.
更多查看译文
关键词
Foreground segmentation,Lightweight model,Real-time inference,Surveillance
AI 理解论文
溯源树
样例
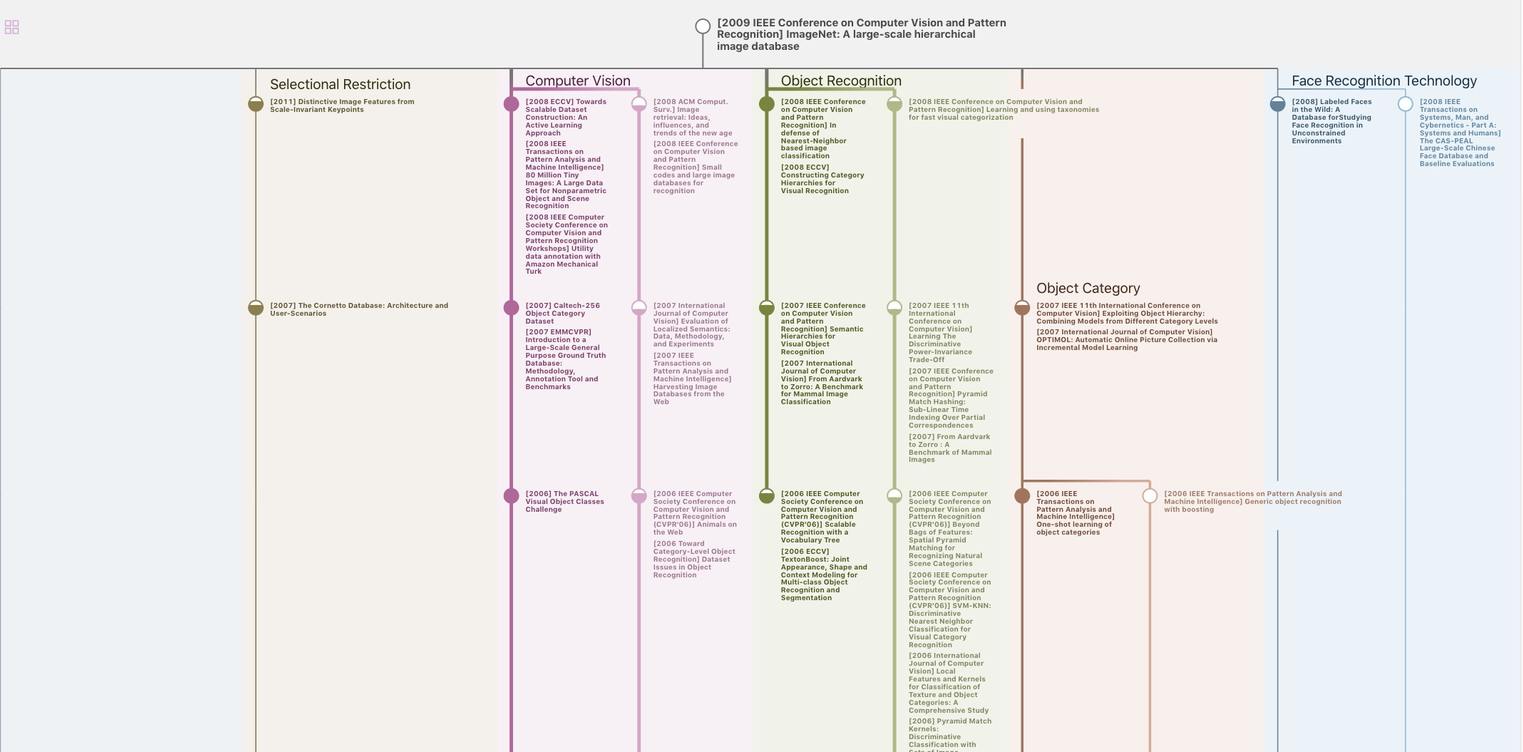
生成溯源树,研究论文发展脉络
Chat Paper
正在生成论文摘要