Morphological-Based Classifications Of Radio Galaxies Using Supervised Machine-Learning Methods Associated With Image Moments
ASTRONOMICAL JOURNAL(2021)
摘要
With the advent of new high-resolution instruments for detecting and studying radio galaxies with different morphologies, the need for the use of automatic classification methods is undeniable. Here, we focused on the morphological-based classification of radio galaxies known as Fanaroff-Riley (FR) type I and type II via supervised machine-learning approaches. Galaxy images with a resolution of 5 '' at 1.4 GHz provided by the Faint Images of the Radio Sky at Twenty centimeters (FIRST) survey are employed. The radial Zernike polynomials are exploited to extract image moments. Then, the rotation, translation, and scale-invariant moments of images are used to form a training set (65% of the radio galaxy sample) and a test set (the remaining 35%). The classes of the test set are determined by two classifiers: a support vector machine and a twin support vector machine (TWSVM). In addition the genetic algorithm is employed to optimize the length of moment series and to find the optimum values of the parameters of the classifiers. The labels of outputs are compared to identify the best performance classifier. To do this the confidence level of classifications is estimated by four different metrics: precision, recall, F1 score, and accuracy. All tests show that implementing TWSVM with the radial basis function as a kernel achieves a confidence level of more than 95% in grouping galaxies.
更多查看译文
关键词
Radio galaxies, Support vector machine, Classification, Catalogs
AI 理解论文
溯源树
样例
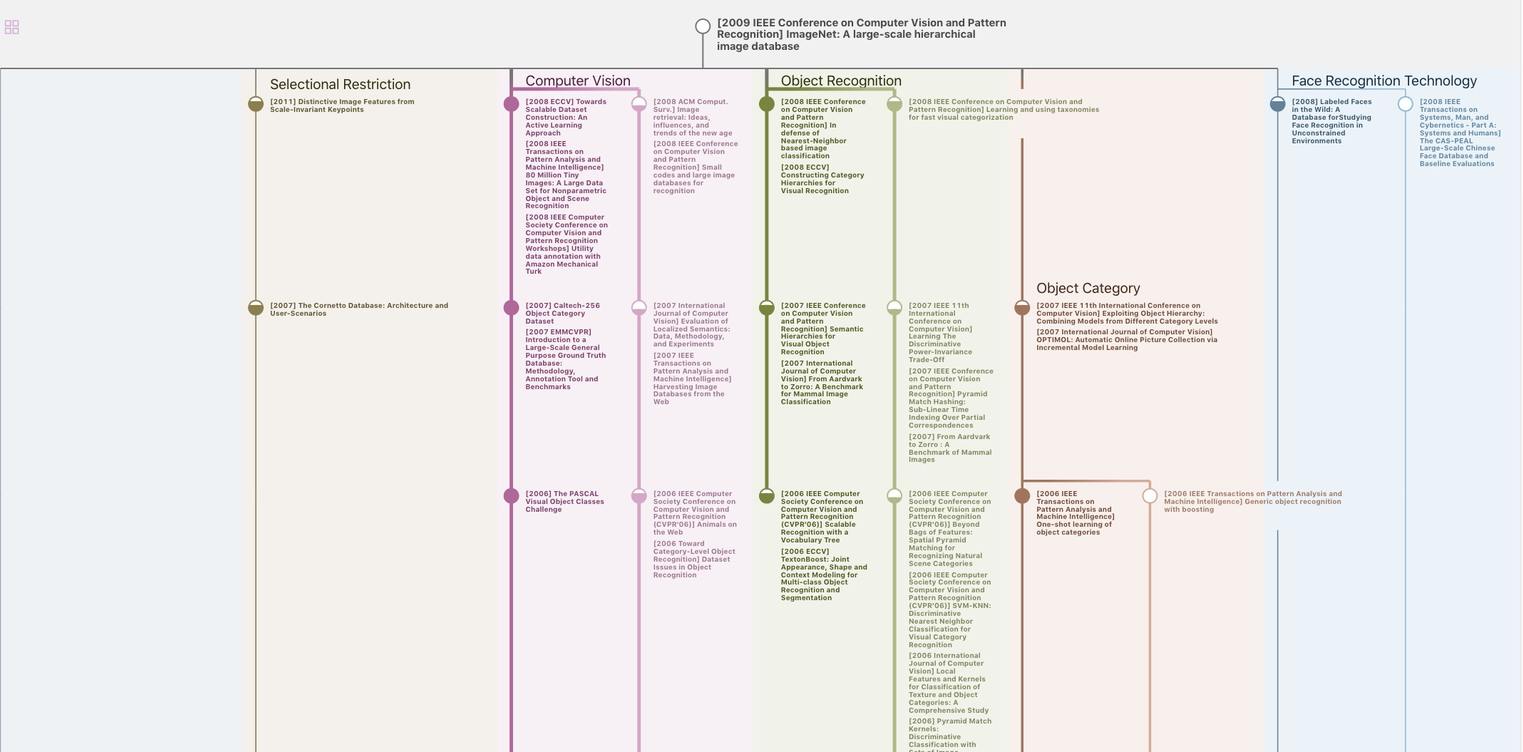
生成溯源树,研究论文发展脉络
Chat Paper
正在生成论文摘要