A Multi-Dimensional Parametric Study Of Variability In Multi-Phase Flow Dynamics During Geologic Co2 Sequestration Accelerated With Machine Learning
APPLIED ENERGY(2021)
摘要
Successful geologic CO2 storage projects depend on numerical simulations to predict reservoir performance during site selection, injection verification, and post-injection monitoring phases of the project. These numerical simulations solve non-linear sets of coupled partial differential equations, while accounting for multi-phase fluid dynamics on the basis of constitutive equations that are embedded into the solution scheme. As a consequence, individual simulations often require tens to hundreds of hours to complete on high-performance computing clusters. Moreover, laboratory experiments reveal that parametric functions for capillary pressure and relative permeability exhibit substantial variability, even within the same rock type. This combination of computational expense and wide-ranging parametric variability means that there remains substantial uncertainty in the behavior of multi-phase CO2-water systems, particularly in the context of feedbacks between relative permeability and capillary pressure. To bridge this knowledge gap, we develop a novel workflow that utilizes physics-based numerical simulation to train an artificial neural network (ANN) emulator for interrogating the multivariate parameter space that governs both capillary pressure and relative permeability. With this approach, the ANN is trained to emulate both fluid pressure distribution and CO2 saturation, which are then interrogated quantitatively to generate parametric response surface mappings with high-fidelity resolution. Results from this study initially show that capillary entry pressure is the dominant control on both CO2 plume geometry and fluid pressure propagation when considering the combined effects of capillary pressure and relative permeability, particularly when phase interference is low and residual CO2 saturation is high. Moreover, the ANN emulator provides tremendous computational speed-up by computing 2691 individual simulations in several minutes; whereas, the same simulation ensemble would have required similar to 3 years of simulation time using only physics-based simulation methods (25,000 times speed up).
更多查看译文
关键词
CO2 sequestration, Machine learning, Capillary pressure, Relative permeability, Parametric analysis
AI 理解论文
溯源树
样例
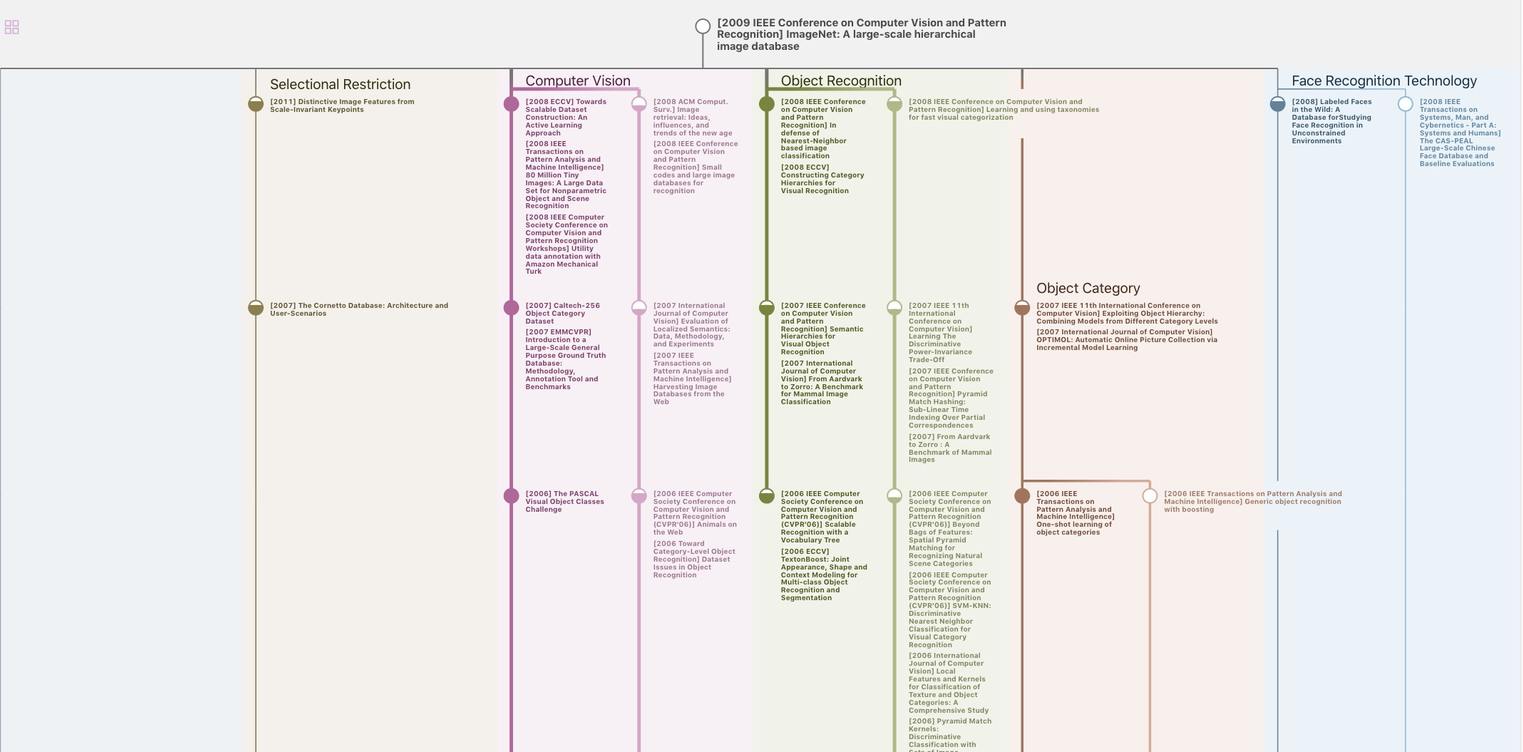
生成溯源树,研究论文发展脉络
Chat Paper
正在生成论文摘要