Mutual-information-inspired heuristics for constraint-based causal structure learning
Information Sciences(2021)
摘要
In constraint-based approaches to Bayesian network structure learning, when the assumption of orientation-faithfulness is violated, not only the correctness of edge orientation can be greatly degraded, the soaring cost of conditional independence testing also limits their applicability in learning very large causal networks. Inspired by the strong connection between the degree of mutual information shared by two variables and their conditional independence, we extend the PC-MI algorithm in two ways: (a) the Weakest Edge-First (WEF) strategy implemented in PC-MI is further integrated with Markov-chain consistency to reduce the number of independence testing and sustain the number of false positive edges in skeletal learning; (b) the Smaller Adjacency-Set (SAS) strategy is proposed and we prove that the Smaller Adjacency-Set captures sufficient information for determining whether an unshielded triple forms a v-structure. We have conducted experiments with both low-dimensional and high-dimensional data sets, and the results indicate that our MIIPC approach outperforms the state-of-the-art approaches in both the quality of learning and the execution time.
更多查看译文
关键词
Causal structure learning,Mutual information,Markov-chain consistency,d-Separation,Smaller adjacency
AI 理解论文
溯源树
样例
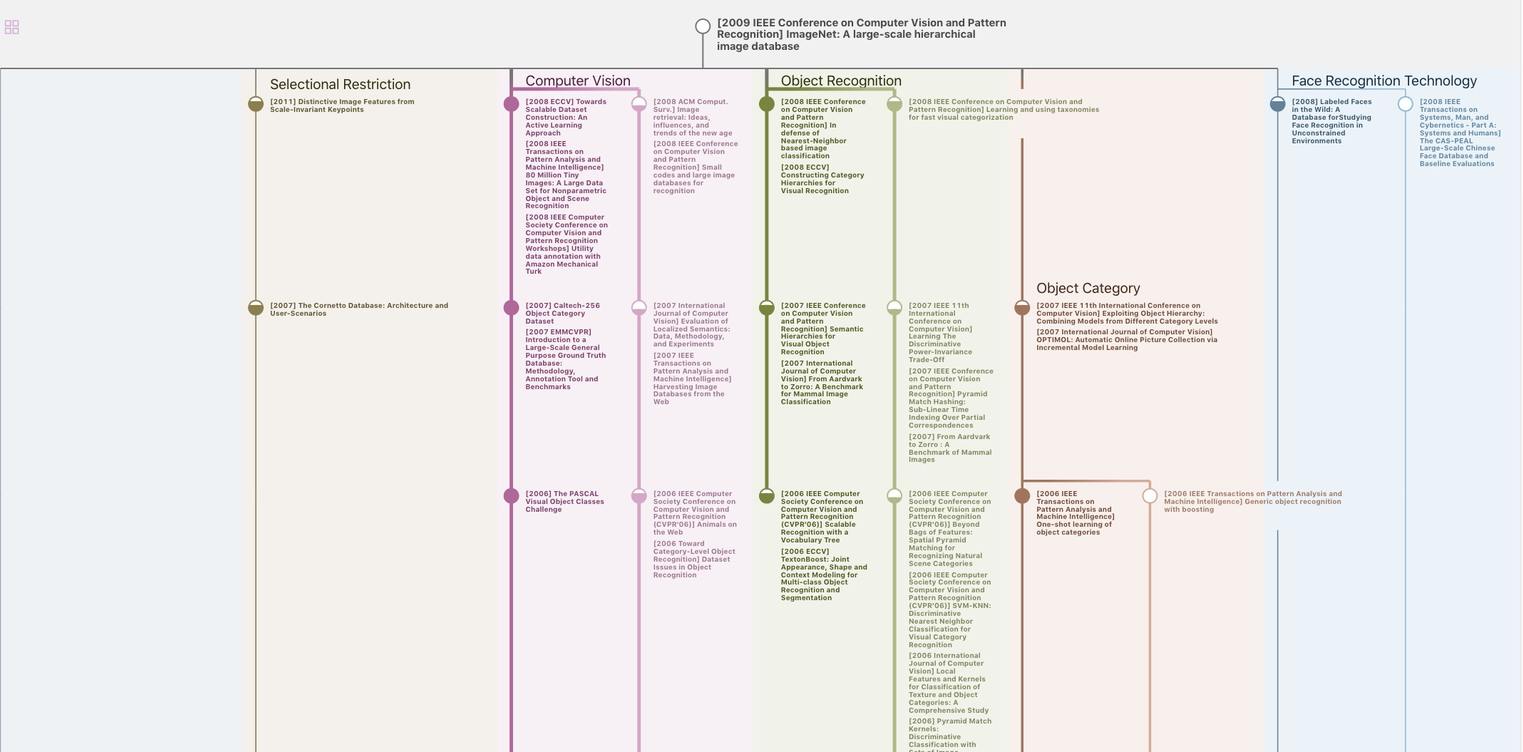
生成溯源树,研究论文发展脉络
Chat Paper
正在生成论文摘要