Real-Time Video Super Resolution Network Using Recurrent Multi-Branch Dilated Convolutions
SIGNAL PROCESSING-IMAGE COMMUNICATION(2021)
摘要
Recent developments of video super-resolution reconstruction often exploit spatial and temporal contexts from input frame sequence by making use of explicit motion estimation, e.g., optical flow, which may introduce accumulated errors and requires huge computations to obtain an accurate estimation. In this paper, we propose a novel multi-branch dilated convolution module for real-time frame alignment without explicit motion estimation, which is incorporated with the depthwise separable up-sampling module to formulate a sophisticated real-time video super-resolution network. Specifically, the proposed video super-resolution framework can efficiently acquire a larger receptive field and learn spatial-temporal features of multiple scales with minimal computational operations and memory requirements. Extensive experiments show that the proposed super-resolution network outperforms current state-of-the-art real-time video super-resolution networks, e.g., VESPCN and 3DVSRnet, in terms of PSNR values (0.49 dB and 0.17 dB) on average in various datasets, but requires less multiplication operations.
更多查看译文
AI 理解论文
溯源树
样例
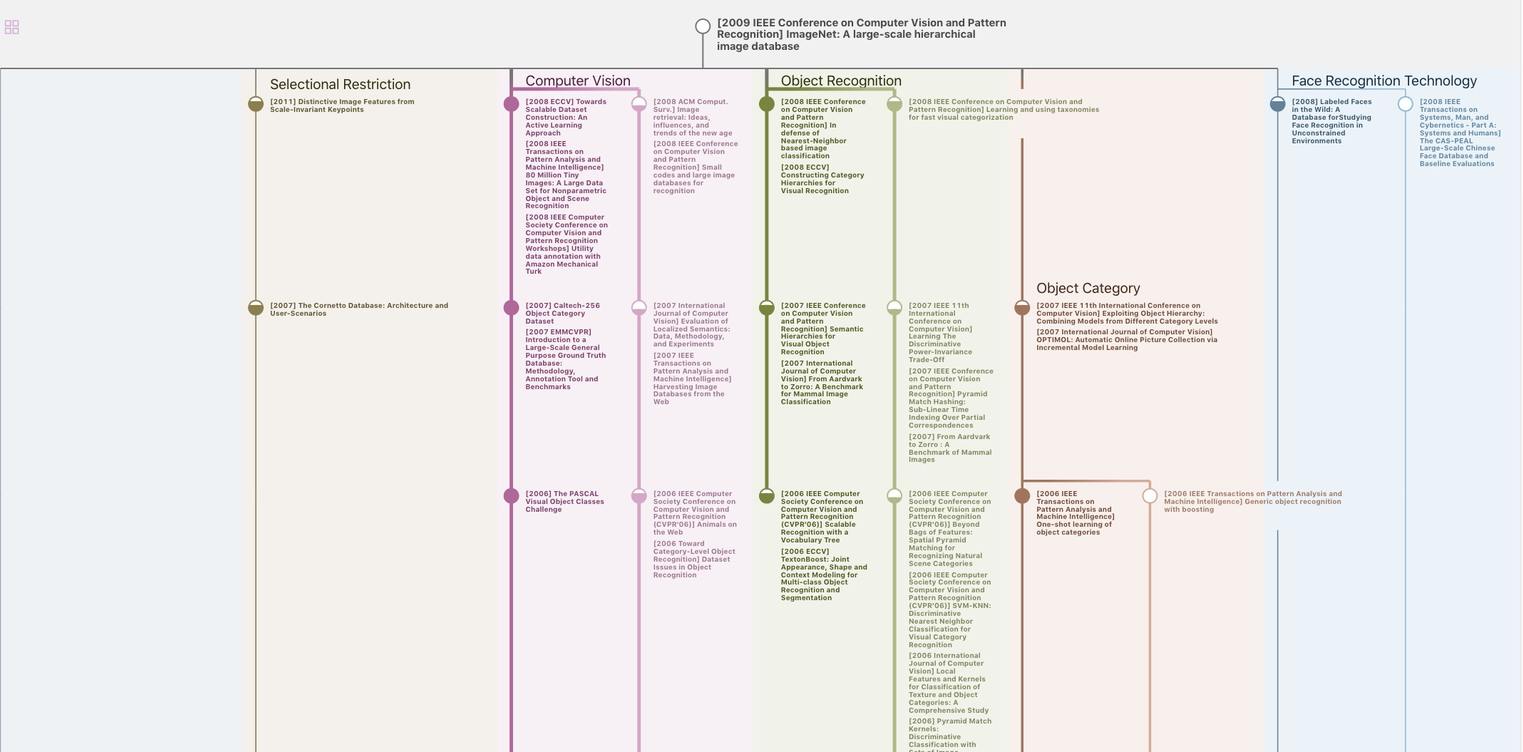
生成溯源树,研究论文发展脉络
Chat Paper
正在生成论文摘要