A Novel Multitask Adversarial Network Via Redundant Lifting For Multicomponent Intelligent Fault Detection Under Sharp Speed Variation
IEEE TRANSACTIONS ON INSTRUMENTATION AND MEASUREMENT(2021)
摘要
Playing a vital role in the safe operation of equipment, intelligent fault detection has been extensively applied in mechanical systems. However, the complicated mapping of signal symptoms and multicomponent failure modes under sharp speed variation makes it hard. The classification error is large due to insufficient samples in the fault condition. Aiming at these problems, a novel multitask redundant lifting adversarial network (MRLAN) is proposed. First, inspired by redundant second-generation wavelet transform, a reconstruction network is established to generate high-quality time-frequency representation of vibration signal, thus eliminating speed fluctuations and expanding training samples. The reconstruction network consists of transposed convolution layer, resume update layer, resume predict layer, and merge layer. Then, multitask category discriminator, which forms dual discriminator together with distance discriminator, is constructed to separate the features of multiple components, thereby realizing multiple tasks (multicomponent fault location and single-component health assessment). Finally, the model is alternately optimized through adversarial process between the generation network and the double discriminators. The proposed method is validated by two cases and other methods are also established to show its superiority. The results show satisfactory performance of MRLAN in multicomponent fault diagnosis under sharp speed fluctuation and little training data.
更多查看译文
关键词
Convolutional neural network (CNN), deep learning, intelligent fault diagnosis, rolling element bearing
AI 理解论文
溯源树
样例
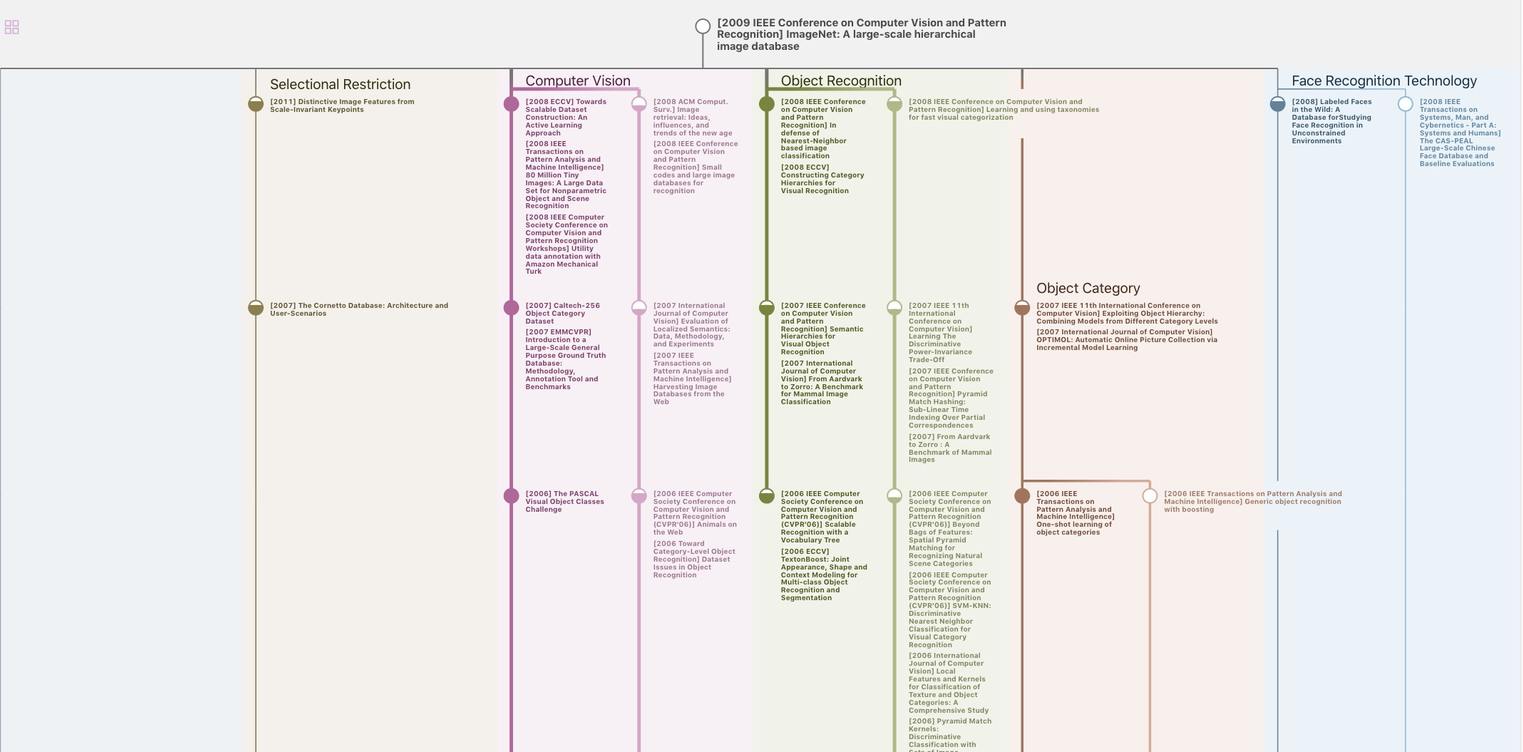
生成溯源树,研究论文发展脉络
Chat Paper
正在生成论文摘要