Experimental Determination For Viscosity Of Fly Ash Nanofluid And Fly Ash-Cu Hybrid Nanofluid:Prediction And Optimization Using Artificial Intelligent Techniques
Energy Sources Part A-recovery Utilization and Environmental Effects(2021)
摘要
The advanced heat transfer applications of nanofluids make it important to investigate their viscous properties. For various engineering applications, accurate measurement of dynamic viscosity of nanofluid plays a major role. This research aims to provide accurate and reliable soft computational algorithms to predict the viscosity of fly ash nanofluids and fly ash-Cu hybrid nanofluids accurately using measured data. In this work, the dynamic viscosity of water-based stable fly ash nanofluid and fly ash-Cu (80:20% by volume) hybrid nanofluid for the concentration range of 0-4 vol.% determined in the temperature range of 30-60 degrees C experimentally. The nanoparticles are characterized by SEM, TEM, and DLS techniques. The stability of the nanofluids assessed by Zeta potential measurement. Outcomes show that the viscosity of hybrid nanofluid is found to be higher than fly ash nanofluid. Correlations are suggested using multiple linear regression analysis to predict the nanofluid viscosity based on the obtained results. We have adopted a novel soft computing technique, namely ANN and MGGP to optimize the dynamic viscosity data of nanofluids obtained experimentally. The MGGP model predicts the viscosity values for fly ash nanofluid by (R = 0.99988, RMSE = 0.0019, and MAPE = 0.25%). In addition, statical analysis reveals that the MGGP model has excellent performance for fly ash-Cu/Water hybrid nanofluid viscosity (R = 0.9975, RMSE = 0.0063, and MAPE = 0.664%). In contrast to the ANN method and multiple linear regression analysis, the MGGP approach exhibits better results in estimating nanofluid viscosity.
更多查看译文
关键词
Fly ash nanofluid, hybrid nanofluid, ann, MGGP, viscosity, regression analysis
AI 理解论文
溯源树
样例
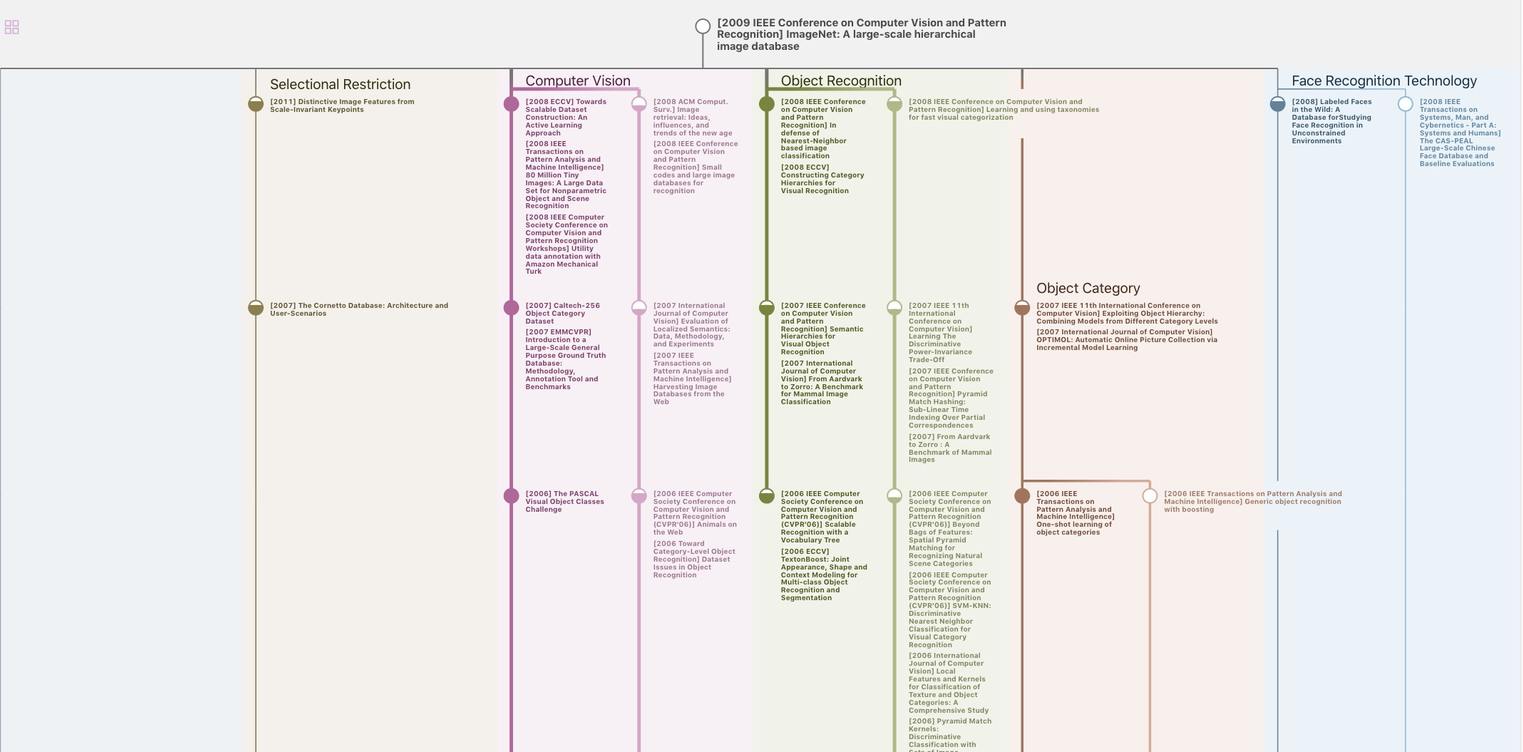
生成溯源树,研究论文发展脉络
Chat Paper
正在生成论文摘要