Leveraging Network Science for Social Distancing to Curb Pandemic Spread
IEEE ACCESS(2021)
摘要
COVID-19 has irreversibly upended the course of human life and compelled countries to invoke national emergencies and strict public guidelines. As the scientific community is in the early stages of rigorous clinical testing to come up with effective vaccination measures, the world is still heavily reliant on social distancing to curb the rapid spread and mortality rates. In this work, we present three optimization strategies to guide human mobility and restrict contact of susceptible and infective individuals. The proposed strategies rely on well-studied concepts of network science, such as clustering and homophily, as well as two different scenarios of the SEIRD epidemic model. We also propose a new metric, called contagion potential, to gauge the infectivity of individuals in a social setting. Our extensive simulation experiments show that the recommended mobility approaches slow down spread considerably when compared against several standard human mobility models. Finally, as a case study of the mobility strategies, we introduce a mobile application, MyCovid, that provides periodic location recommendations to the registered app users.
更多查看译文
关键词
Optimization,COVID-19,Social factors,Human factors,Analytical models,Testing,Statistics,Social distancing,network science,clustering,optimization,homophily
AI 理解论文
溯源树
样例
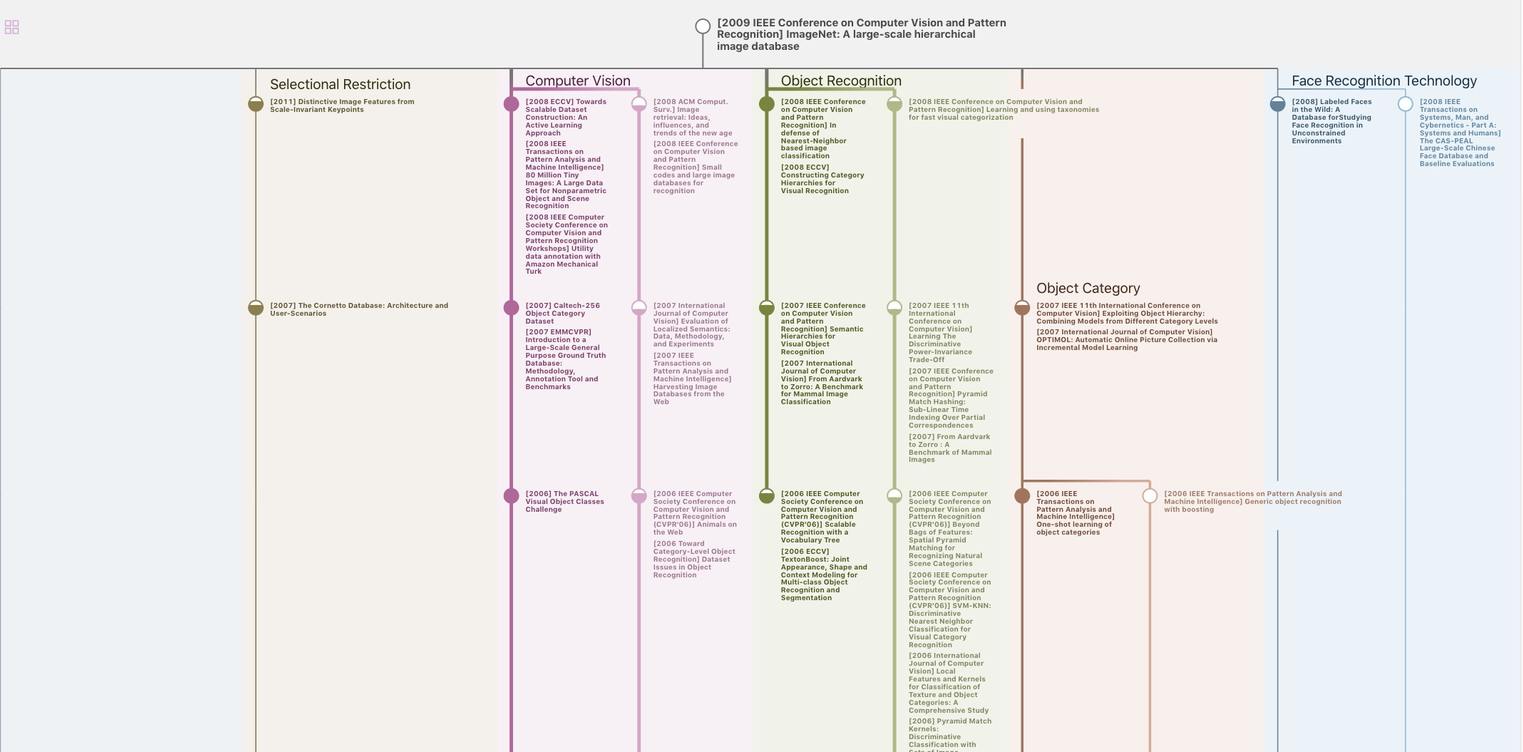
生成溯源树,研究论文发展脉络
Chat Paper
正在生成论文摘要