Improved Adversarial Attack against Black-box Machine Learning Models
chinese automation congress(2020)
摘要
The existence of adversarial samples makes the security of machine learning models in practical application questioned, especially the black-box adversarial attack, which is very close to the actual application scenario. Efficient search for black-box attack samples is helpful to train more robust models. We discuss the situation that the attacker can get nothing except the final predict label. As for this problem, the current state-of-the-art method is Boundary Attack(BA) and its variants, such as Biased Boundary Attack(BBA), however it still requires large number of queries and kills a lot of time. In this paper, we propose a novel method to solve these shortcomings. First, we improved the algorithm for generating initial adversarial samples with smaller L 2 distance. Second, we innovatively combine a swarm intelligence algorithm——Particle Swarm Optimization(PSO) with Biased Boundary Attack and propose PSO-BBA method. Finally, we experiment on ImageNet dataset, and compared our algorithm with the baseline algorithm. The results show that:(1)our improved initial point selection algorithm effectively reduces the number of queries;(2)compared with the most advanced methods, our PSO-BBA method improves the convergence speed while ensuring the attack accuracy;(3)our method has a good effect on both targeted attack and untargeted attack.
更多查看译文
关键词
adversarial samples,machine learning models,boundary attack,PSO
AI 理解论文
溯源树
样例
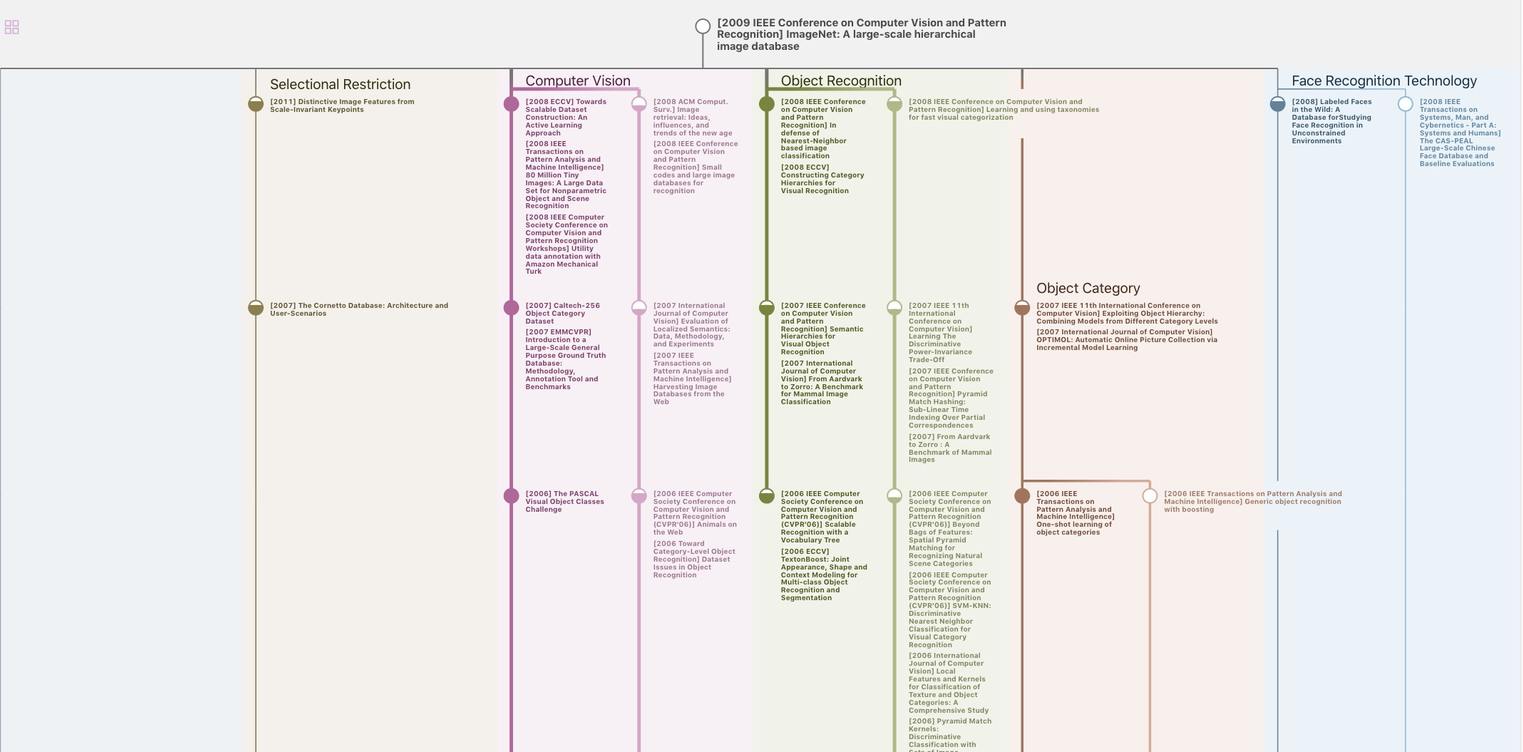
生成溯源树,研究论文发展脉络
Chat Paper
正在生成论文摘要