Optimizing a polynomial function on a quantum processor
NPJ QUANTUM INFORMATION(2021)
摘要
The gradient descent method is central to numerical optimization and is the key ingredient in many machine learning algorithms. It promises to find a local minimum of a function by iteratively moving along the direction of the steepest descent. Since for high-dimensional problems the required computational resources can be prohibitive, it is desirable to investigate quantum versions of the gradient descent, such as the recently proposed (Rebentrost et al. 1 ). Here, we develop this protocol and implement it on a quantum processor with limited resources. A prototypical experiment is shown with a four-qubit nuclear magnetic resonance quantum processor, which demonstrates the iterative optimization process. Experimentally, the final point converged to the local minimum with a fidelity >94%, quantified via full-state tomography. Moreover, our method can be employed to a multidimensional scaling problem, showing the potential to outperform its classical counterparts. Considering the ongoing efforts in quantum information and data science, our work may provide a faster approach to solving high-dimensional optimization problems and a subroutine for future practical quantum computers.
更多查看译文
关键词
Quantum information,Quantum simulation,Physics,general,Quantum Physics,Quantum Information Technology,Spintronics,Quantum Computing,Quantum Field Theories,String Theory,Classical and Quantum Gravitation,Relativity Theory
AI 理解论文
溯源树
样例
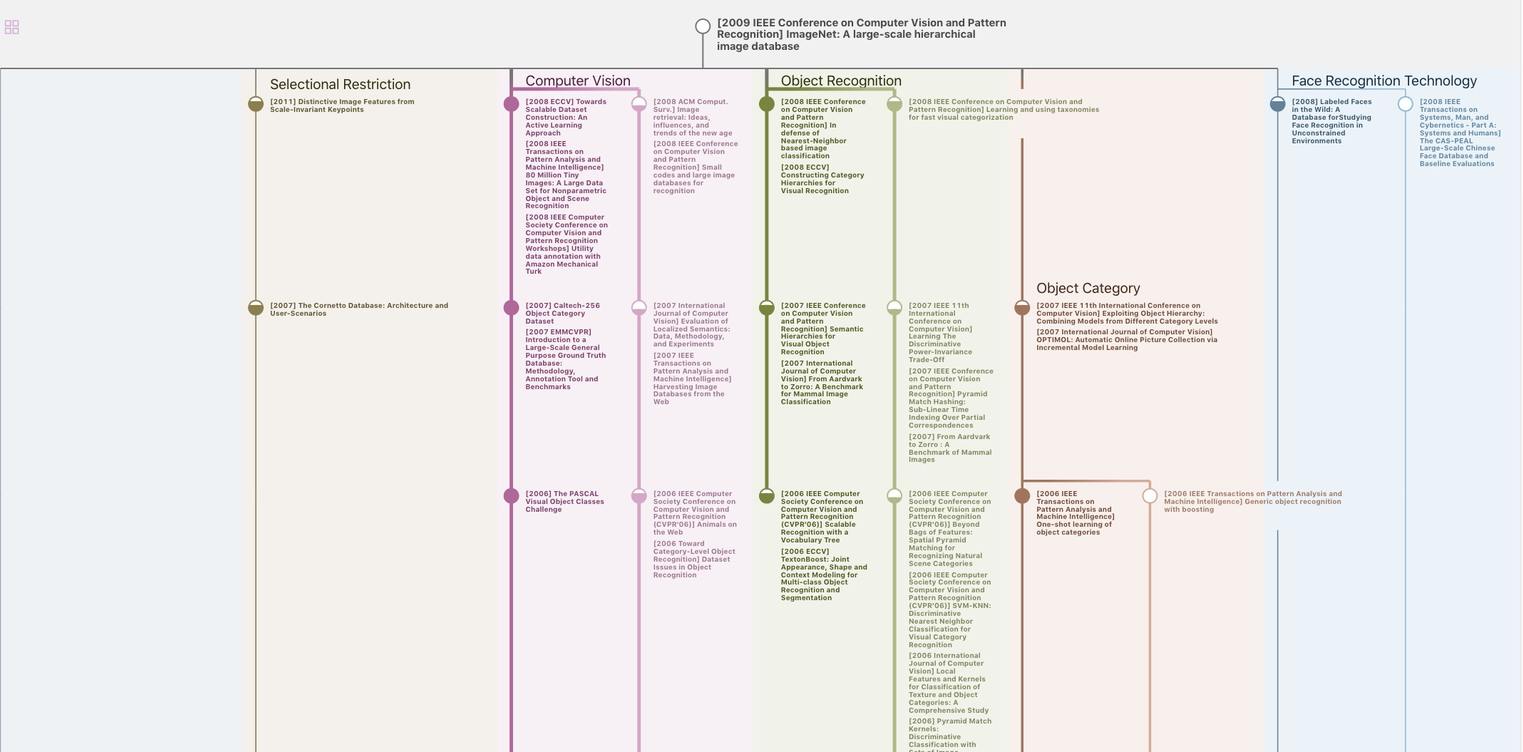
生成溯源树,研究论文发展脉络
Chat Paper
正在生成论文摘要