A reliable KNN filling approach for incomplete interval-valued data
Engineering Applications of Artificial Intelligence(2021)
摘要
Interval-valued data (IVD) is a kind of data where each feature is an interval, and embeds the uncertainty and variability information. However, the missing values (lower or upper bound, or both of them are missed) may occur in the process of data acquisition and transmission, which may lead to obstacles for data processing. To obtain good results, it is important for IVD to process (often ignore or fill) the missing values. A dataset including missing values is named as incomplete interval-valued (IIV) set here. Some ignoring and filling methods for numeric or symbolic data have been proposed, but they cannot be applied for IIV datasets directly. In this work, a reliable k-nearest neighbor approach (RKNN) for incomplete interval-valued data (IIVD) is proposed. A combining rule to determine whether a datum including missing values should be ignored or filled is designed. Those samples with the missing value for each feature will be ignored directly. It is different from existing ignoring methods that need to set the percentage of missing entries. For the rest of missing samples, they will be filled according to their K complete nearest neighbors, which can ensure the filled value more reliable. In so doing, RKNN can exclude a small number of missing samples that may increase uncertainty, and avoid the repetition of the filled values (like median or a fixed constant). The experiment results on 12 synthetic datasets and 4 real-world datasets demonstrate that the proposed method can process the incomplete interval-valued data effectively, and obtain a good classification performance simultaneously.
更多查看译文
关键词
Interval-valued data,Incomplete interval-valued set,Missing value,Combining rule
AI 理解论文
溯源树
样例
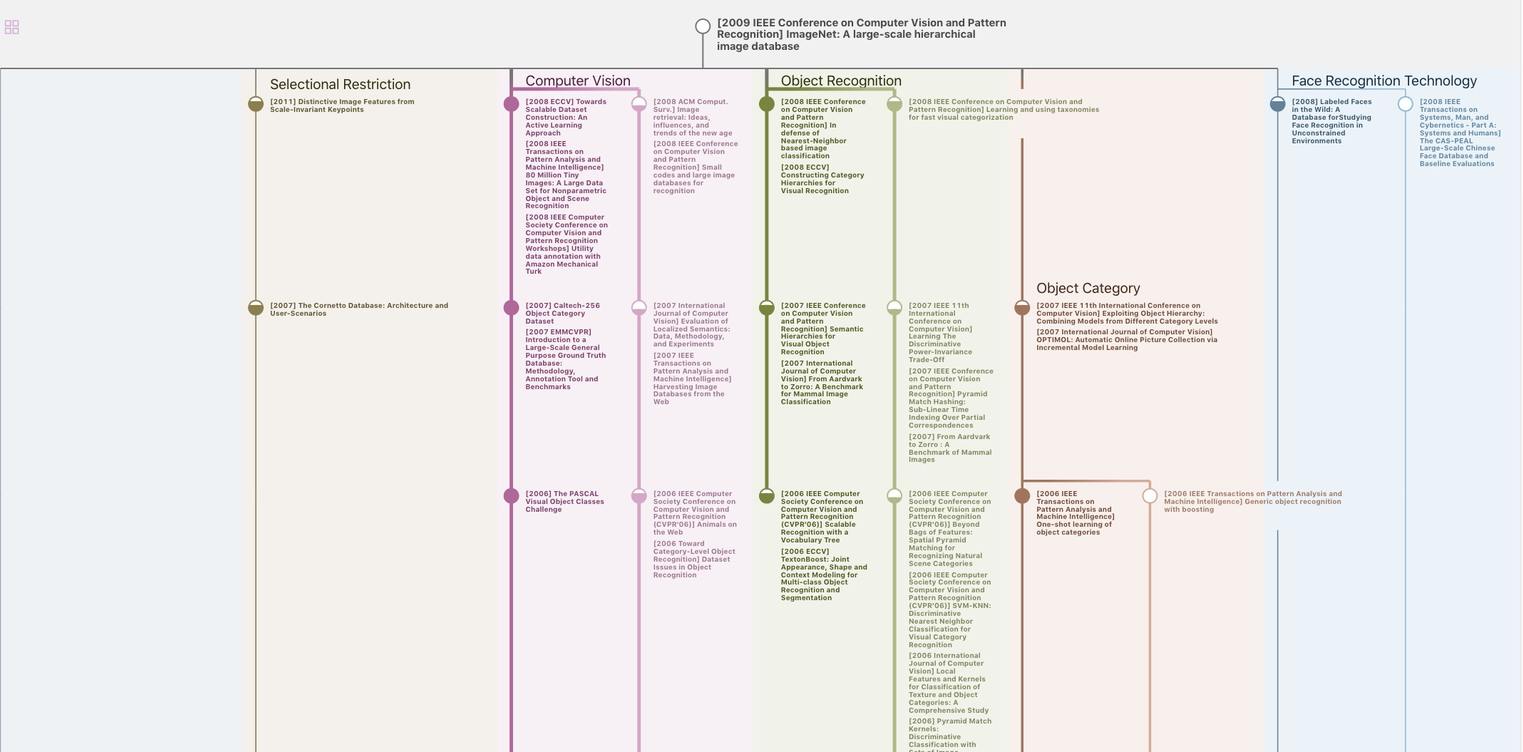
生成溯源树,研究论文发展脉络
Chat Paper
正在生成论文摘要