Effects of Lossy Compression on Remote Sensing Image Classification Based on Convolutional Sparse Coding
IEEE GEOSCIENCE AND REMOTE SENSING LETTERS(2022)
摘要
Lossy compression causes the degradation of the classification accuracy of remote sensing (RS) images due to the introduced distortion by compression. In this letter, a convolutional sparse coding (CSC)-based method is proposed to quantitatively measure such an effect. In detail, the filters used in CSC are learned by online convolutional dictionary learning (OCDL) to construct the dictionary. Thereafter, the sparse coefficient maps are obtained based on the alternating direction method of multipliers (ADMM) algorithm. In addition, multiple kernel learning (MKL) is used to estimate the corresponding classification accuracy. The experimental results demonstrate that our method performs better in predicting the classification accuracy of RS images compared with the other state-of-the-art algorithms.
更多查看译文
关键词
Image coding, Dictionaries, Image reconstruction, Feature extraction, Convolutional codes, Remote sensing, Prediction algorithms, Convolutional sparse coding (CSC), image classification, image compression, online convolutional dictionary learning (OCDL), remote sensing (RS) image
AI 理解论文
溯源树
样例
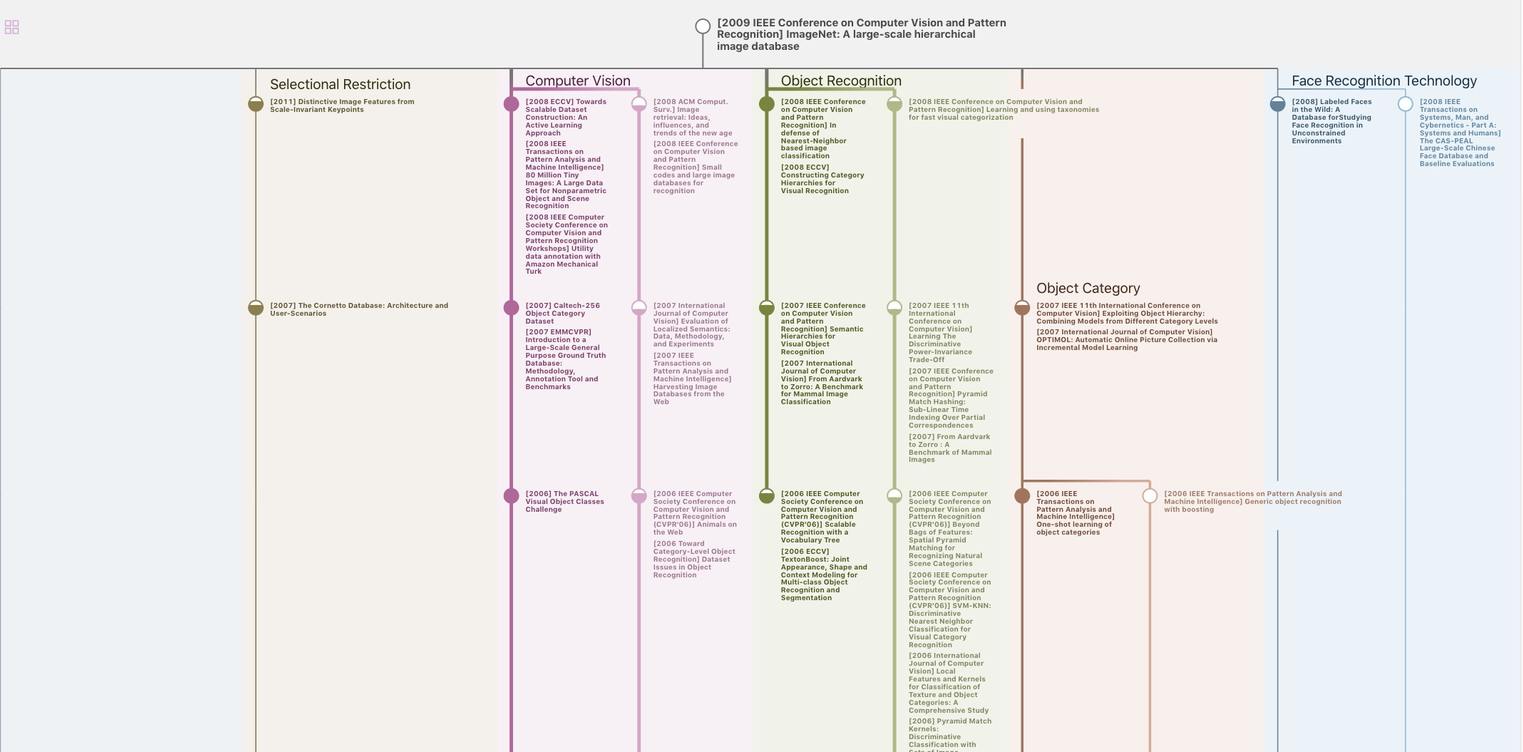
生成溯源树,研究论文发展脉络
Chat Paper
正在生成论文摘要