A Hyperspectral Anomaly Detection Method Based on Low-Rank and Sparse Decomposition With Density Peak Guided Collaborative Representation
IEEE TRANSACTIONS ON GEOSCIENCE AND REMOTE SENSING(2022)
摘要
The low-rank and sparse decomposition model (LSDM) has been widely studied by researchers and has successfully solved the problem of hyperspectral image (HSI) anomaly detection (AD). The traditional LSDM usually ignores the information of the low-rank matrix, which only detects the anomalous targets by using the sparse component. To utilize both the sparse component and the low-rank component comprehensively, an anomaly detector for HSIs based on LSDM with density peak guided collaborative representation (LSDDPCRD) is proposed in this article. First, the LSDM technique with the mixture of Gaussian model is used to decompose the original HSI, which can also alleviate the background noise contamination problem. Then, the low-rank matrix is detected by the density peak guided collaborative representation detection algorithm, while the sparse matrix is calculated according to the Manhattan distance. In addition, an entropy-based adaptive fusing method is designed to combine the results obtained from the low-rank matrix and the sparse component. It could choose the fusing weights adaptively according to the characteristics of an HSI. The experimental results indicate that the LSDDPCRD performs better than eight classical and state-of-the-art AD algorithms (GRX, LRX, SRX-Segmented, CRD, RPCA-RX, LSMAD, LRASR, and LSDM-MoG) on four real HSIs.
更多查看译文
关键词
Sparse matrices, Hyperspectral imaging, Detectors, Matrix decomposition, Covariance matrices, Collaboration, Task analysis, Collaborative representation, density peak, hyperspectral anomaly detection (AD), hyperspectral image (HSI), low-rank and sparse decomposition
AI 理解论文
溯源树
样例
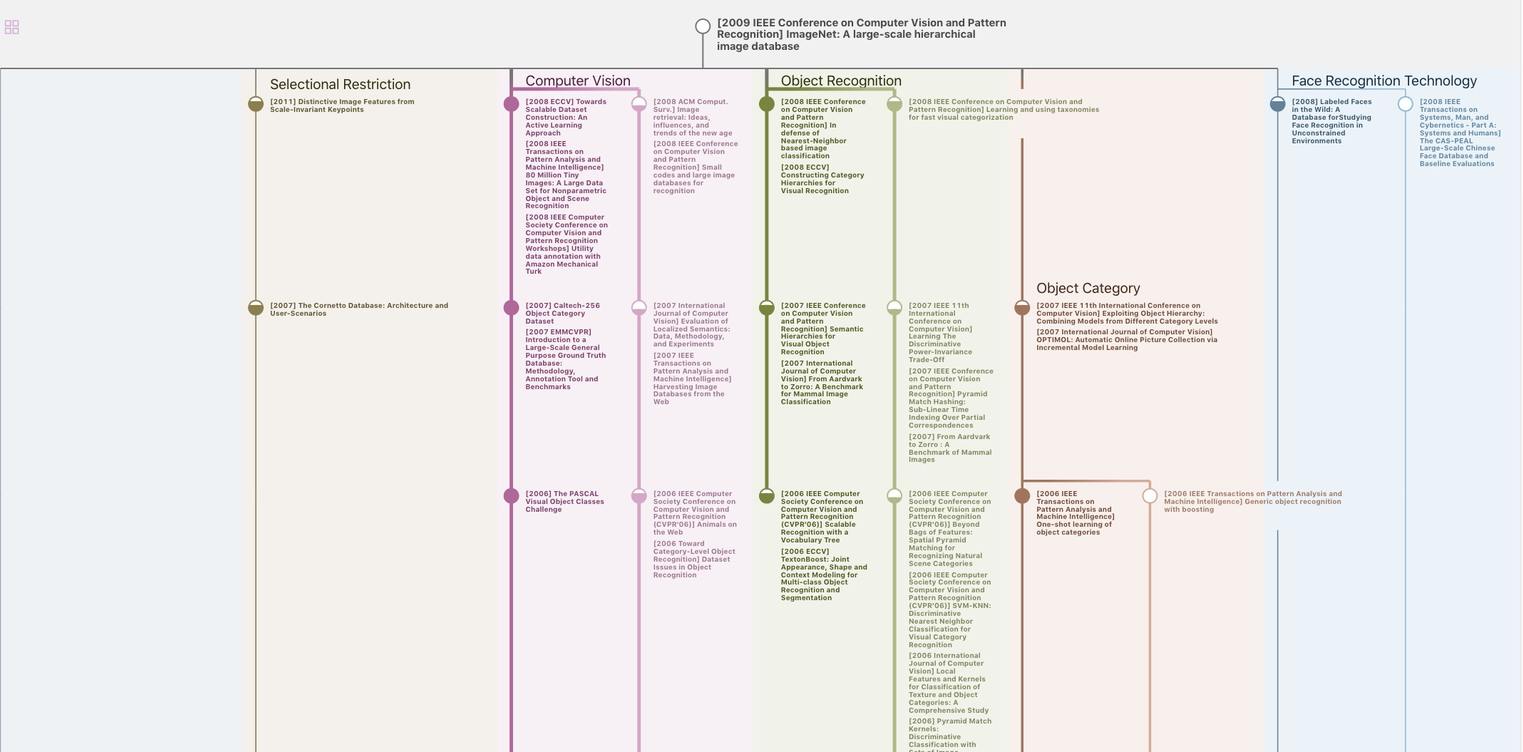
生成溯源树,研究论文发展脉络
Chat Paper
正在生成论文摘要