Interest-Driven Exploration With Observational Learning for Developmental Robots
IEEE Transactions on Cognitive and Developmental Systems(2023)
摘要
It has been emphasized for a long time that real-world applications of developmental robots require lifelong and online learning. A major challenge in this field is the high sample-complexity of algorithms, which has led to the development of intrinsic motivation approaches to render learning more efficient. However, only few works have been demonstrated on real robots and although these robots are supposed to share the environment with humans, there is hardly any research to integrate intrinsic motivation with learning from an interacting teacher. In this article, we tackle the efficiency challenge by proposing a novel extrinsic-intrinsic motivation learning scheme. We specifically investigate how to combine intrinsic motivation with learning from observation to accelerate learning. Our novel scheme comprises four elements: 1) a probabilistic intrinsic motivation signal yielding the robot's interest; 2) a probabilistic extrinsic motivation signal to expand the robot's knowledge by learning from observation; 3) novelty detection; and 4) novelty degree methods to enable the robot to decide autonomously how and when to explore. The efficiency as well as the applicability of our methods are benchmarked in simulation experiments and demonstrated on a physical 7-degree of freedom left arm of Baxter robot.
更多查看译文
关键词
Robots,Robot kinematics,Task analysis,Probabilistic logic,Training,Manipulators,Knowledge based systems,Developmental robot,direct inverse kinematics learning from observation,intrinsic motivation,learning,online learning,robot model learning,socially guided exploration
AI 理解论文
溯源树
样例
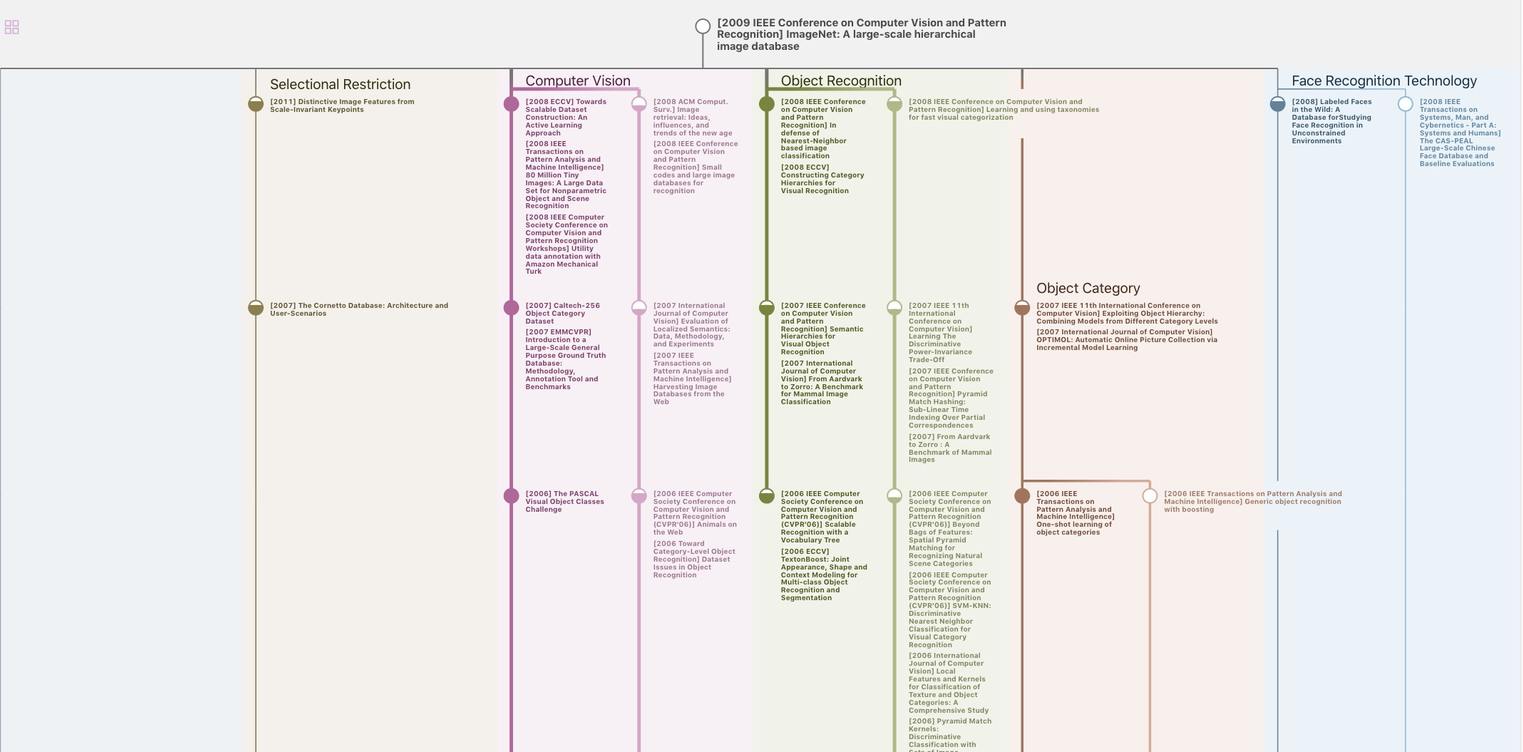
生成溯源树,研究论文发展脉络
Chat Paper
正在生成论文摘要