BANDIT THEORY: APPLICATIONS TO LEARNING HEALTHCARE SYSTEMS AND CLINICAL TRIALS
STATISTICA SINICA(2021)
摘要
In recent years, statisticians and clinical scientists have defined two new approaches for studying the effects of medical practice, extending the "gold standard" classical randomized clinical trial to remedy some of its defects, improve its fit to clinical practice, and conform more closely to ethical principles. The contextual multi-armed bandit provides a natural statistical structure for a learning healthcare system, allowing the optimization of patient outcomes by adaptively assigning treatments, while building in experimental strength for accuracy in learning. The sequential multiple assignment randomized trial has become the standard for comparing entire dynamic treatment strategies for the management of chronic disease, which more closely matches the goals and practice of clinicians. The theory and methods developed by Professor Tze Leung Lai over the course of his career are of central importance in bringing these two apparently different approaches to bear in efforts to improve clinical practice. We review these methods in this article.
更多查看译文
关键词
Clinical trials, medical and pharmaceutical statistics, se-quential analysis and optimal stopping
AI 理解论文
溯源树
样例
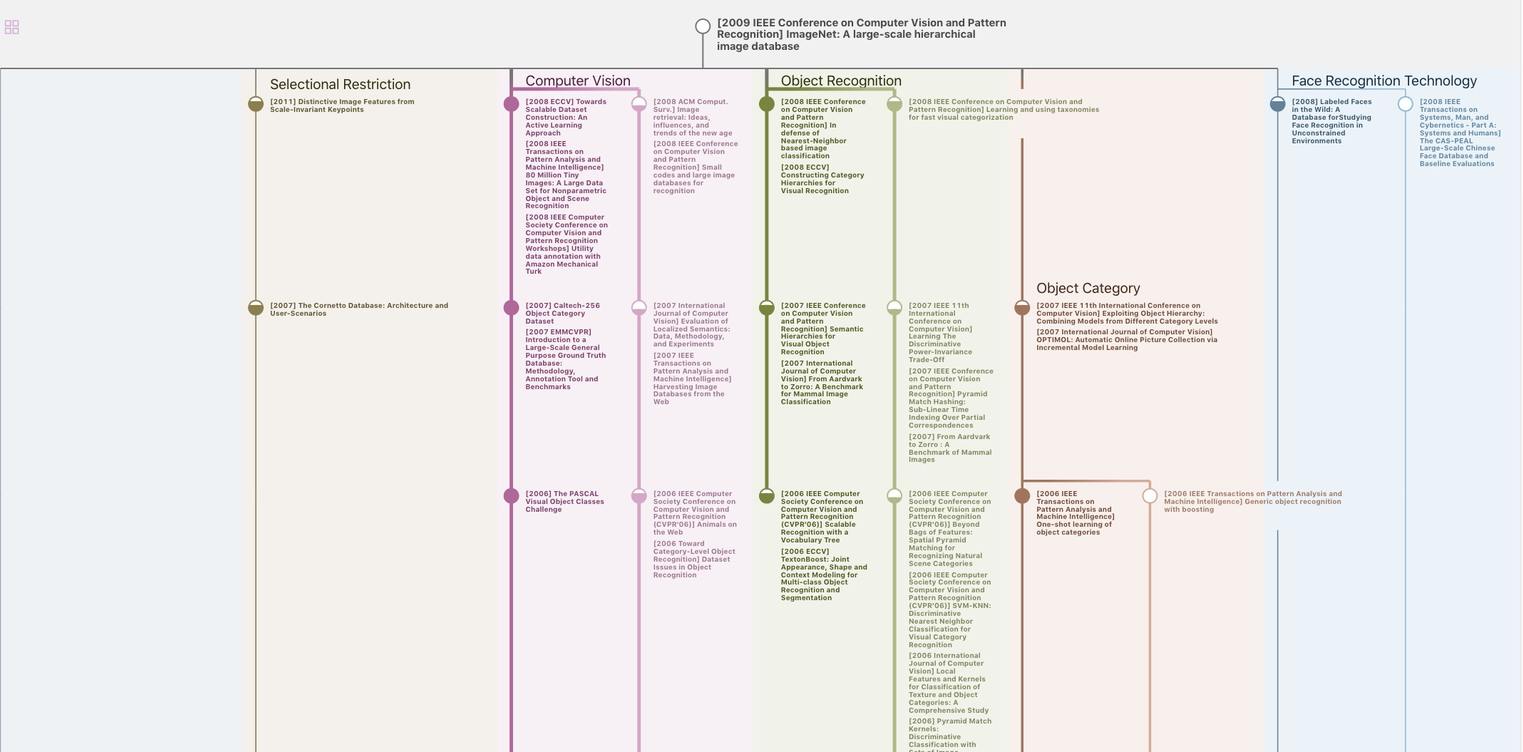
生成溯源树,研究论文发展脉络
Chat Paper
正在生成论文摘要