Neurons with dendrites can perform linearly separable computations with low resolution synaptic weights [version 2; peer review: 2 approved, 1 approved with reservations]
F1000Research(2021)
摘要
In theory, neurons modelled as single layer perceptrons can implement all linearly separable computations. In practice, however, these computations may require arbitrarily precise synaptic weights. This is a strong constraint since both biological neurons and their artificial counterparts have to cope with limited precision. Here, we explore how non-linear processing in dendrites helps overcome this constraint. We start by finding a class of computations which requires increasing precision with the number of inputs in a perceptron and show that it can be implemented without this constraint in a neuron with sub-linear dendritic subunits. Then, we complement this analytical study by a simulation of a biophysical neuron model with two passive dendrites and a soma, and show that it can implement this computation. This work demonstrates a new role of dendrites in neural computation: by distributing the computation across independent subunits, the same computation can be performed more efficiently with less precise tuning of the synaptic weights. This work not only offers new insight into the importance of dendrites for biological neurons, but also paves the way for new, more efficient architectures of artificial neuromorphic chips.
更多查看译文
AI 理解论文
溯源树
样例
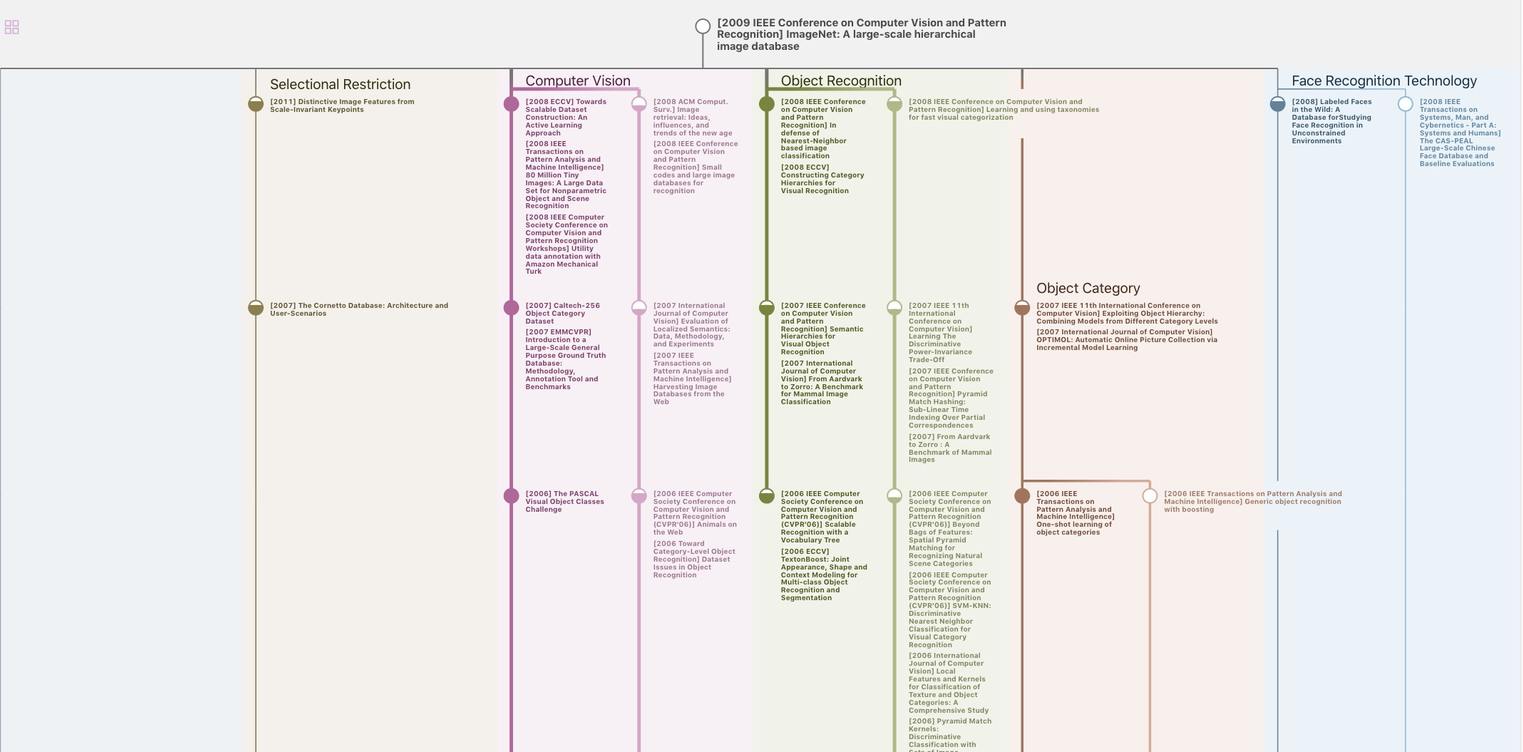
生成溯源树,研究论文发展脉络
Chat Paper
正在生成论文摘要