Complementary Evidence Identification in Open-Domain Question Answering
EACL(2021)
摘要
This paper proposes a new problem of complementary evidence identification for open-domain question answering (QA). The problem aims to efficiently find a small set of passages that covers full evidence from multiple aspects as to answer a complex question. To this end, we proposes a method that learns vector representations of passages and models the sufficiency and diversity within the selected set, in addition to the relevance between the question and passages. Our experiments demonstrate that our method considers the dependence within the supporting evidence and significantly improves the accuracy of complementary evidence selection in QA domain.
更多查看译文
关键词
evidence,open-domain
AI 理解论文
溯源树
样例
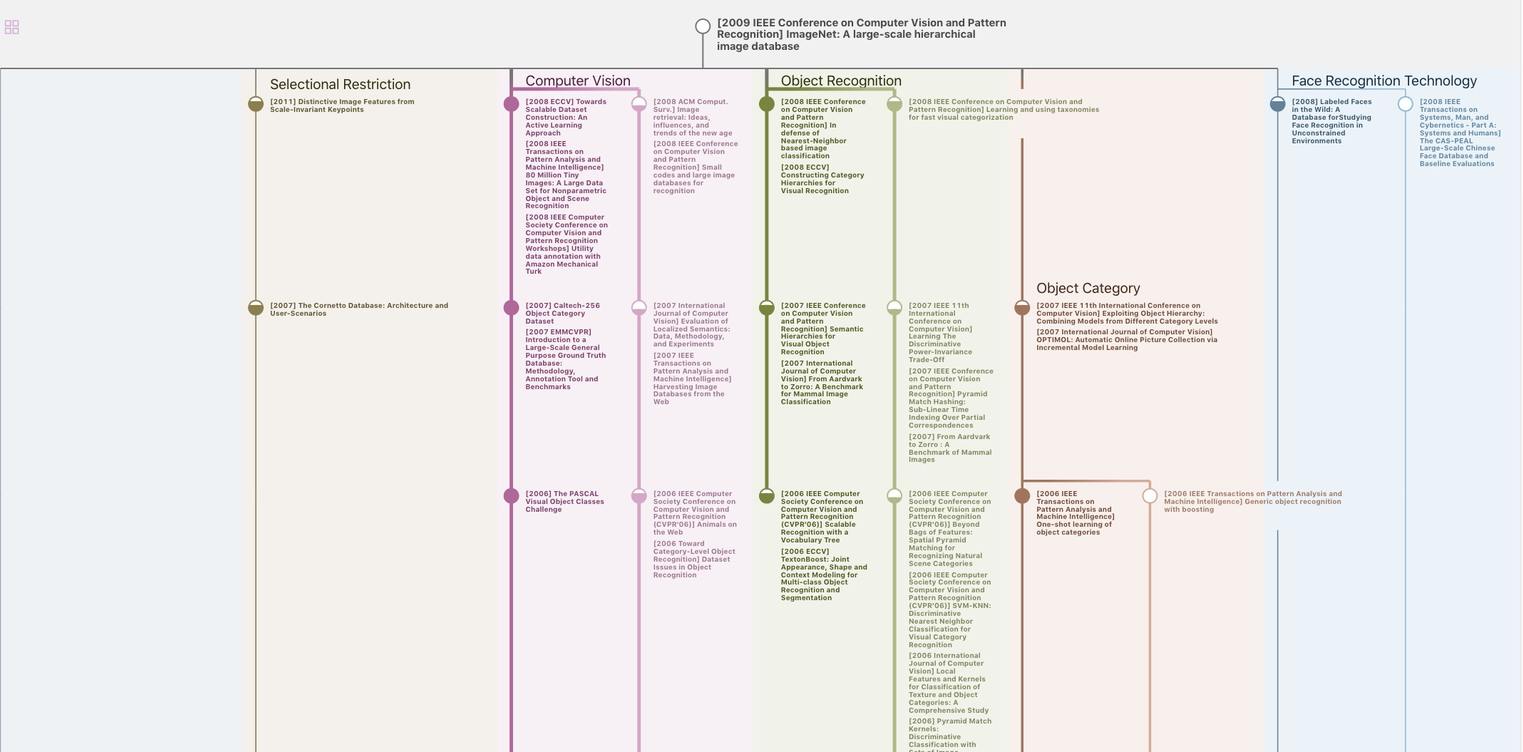
生成溯源树,研究论文发展脉络
Chat Paper
正在生成论文摘要